3rd Workshop on Statistical and Algorithmic Challenges in Microbiome Data Analysis
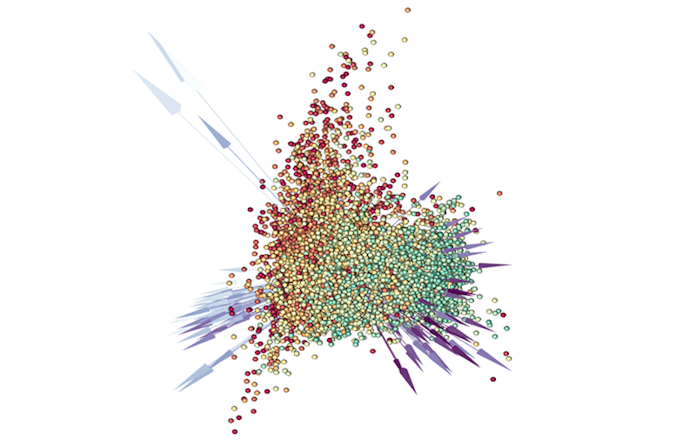
Center for Computational Biology (Simons Foundation, NYU), and the Center for Microbiome Informatics and Therapeutics (at MIT) will hold their Third Workshop on Statistical and Algorithmic Challenges in Microbiome Data Analysis at The Simons Foundation, 160 Fifth Avenue, 2nd Floor, G.D.F. Auditorium, New York, New York 10010 on April 1 – 2, 2019.
As with previous years, the SACMDA workshop is a forum to advance research within the growing community of developers of computational and statistical methods from the microbiome field and related disciplines. Dissemination, exchange, and synthesis of ideas is encouraged by inviting by junior faculty members or postdoctoral associates to present the tools and algorithms that they conceived and developed. Plenty of time will be allocated for discussion so that peers and senior faculty can provide constructive feedback and initiate collaboration.
Susan Holmes has been selected as our Keynote to inspire the group to rise to new challenges.
- Workshop Videos
-
Agenda
See detailed schedule here.
-
Participants
NameAffiliation
Abigail Armstrong University of Denver Francesco Asnicar University of Trento Bahar Behsaz University of California San Diego Antoine Bodein Université Laval Evan Bolyen Northern Arizona University Hector Corrada Bravo University of Maryland, College Park M. Luz Calle Rosingana Universitat de Vic (Barcelona, Spain) Liu Cao Carnegie Mellon University David Clausen University of Washington Rachel Colquhoun The European Molecular Biology Laboratory Christian Diener Institute for Systems Biology Juan José Egozcue Rubi Technical University of Catalonia Georg Gerber Massachusetts Host Microbiome Center Sean Gibbons Institute for Systems Biology Travis E. Gibson Massachusetts Host Microbiome Center Greg Gloor University of Western Ontario Susan Holmes Stanford University Jiyuan Hu New York University Langone Medical Center Lu Huang University of Pennsylvania Pratheepa Jeganathan Stanford University Xiaofang Jiang Massachusetts Institute of Technology / The Broad Devin Jones Montana State University Christopher Keefe Northern Arizona University Kim-Anh Le Cao University of Melbourne Hongzhe Lee University of Pennsylvania Jeff Leek John Hopkins Bloomberg School of Public Health Huilin Li New York University Langone Medical Center Catherine Lozupone University of Denver Cameron Martino University of California, San Diego Rajita Menon Boston University Hosein Mohimani Carnegie Mellon University Senthil Kumar Muthiah. University of Maryland, College Park Vera Palowsky-Glahn University of Girona Mihai Pop University of Maryland Christopher Quince University of Warwick Javier Rivera Pinto University of Girona Kimberly Roche Duke University Nicola Segata University of Trento Nidhi Shah University of Maryland Justin Silverman Duke University Siruo Wang John Hopkins Bloomberg School of Public Health Alex Washburne Montana State University Amy Willis University of Washington Jakob Wirbel The European Molecular Biology Laboratory -
Organizing Committee
Center for Microbiome Informatics & Therapeutics
Eric Alm, Ph.D.
Vicki Mountain
Claire DuvalletFlatiron Institute / New York University
Richard Bonneau, Ph.D.
Michelle BadriUniversity of California, San Diego
Rob Knight, Ph.D.
James Morton, Ph.D. -
Poster
Guidelines
Following the guidelines below, abstracts should be submitted to as a pdf files [LAST NAME-FIRST NAME.PDF]. DEADLINE: March 4. In order to be considered to present a poster, in addition to submitting your abstract, you must register for the conference. While registering, please indicate that you will be submitting an abstract and provide the abstract title.
Guidelines must be followed for your abstract to be considered! Abstract Guidelines:
- Abstract Title: Arial, Bold, 12 pt., centered. Maximum of 150 characters, including spaces.
- Abstract Authors: Arial, 12 pt., centered. Include, all authors full name and affiliation. Use superscript to indicate multiple or varying affiliations.
- Authors Address(es): Arial, 11 pt., justified.
- TEXT ONLY abstract: Arial, 12 pt., justified. Maximum of 500 words.
- Maximum size 48″w x 42″h
Submit here
Example
Microbes are STICKY – Inference and Topological analysis of microbial ecological networks
Christian L. Müller1, Zachary D. Kurtz4, Emily R. Miraldi1, Richard Bonneau1, 2, 3
1 Simons Center for Data Analysis, Simons Foundation, New York, NY 10010
2 Department of Biology, Center for Genomics and Systems Biology, NYU, New York, NY 10003
3 Courant Institute of Mathematical Sciences, New York University, New York, NY 10012
4 Departments of Microbiology and Medicine, New York University School of Medicine, New York, NY 1001616S-ribosomal sequencing and metagenomic measurements of microbial communities reveal phylogeny and the abundances of microbial populations across diverse ecosystems. While changes in microbial community structure are demonstrably associated with certain environmental conditions (from metabolic and immunological health in mammals to ecological stability in soils and oceans) and associations between microbes, identification of underlying mechanisms and microbial ecological networks requires new statistical tools. A key challenge is that metagenomic and 16S data are typically compositional (counts are normalized to the total number of counts in the sample due to limits in sequencing capacity). Thus, microbial abundances are not independent, and traditional statistical metrics (e.g., correlation) for the detection of OTU-OTU relationships can lead to spurious results. We will first present SPIEC-EASI (SParse InversE Covariance Estimation for Ecological Association Inference), a statistical method the inference of microbial ecological associations from metagenomic datasets that addresses both of these issues. SPIEC- EASI combines compositional data transformations with algorithms for sparse neighborhood and inverse covariance selection. Because no large-scale microbial ecological networks have been experimentally validated, SPIEC-EASI is accompanied by a set of computational tools to generate realistic OTU count data from a set of diverse underlying network topologies. SPIEC-EASI outperforms state-of-the-art methods in terms of edge recovery and network properties on realistic synthetic data under a variety of scenarios. SPIEC-EASI also reproducibly predicts previously unknown microbial associations using data from the American Gut project. Given this method we will then present a topological analysis of inferred networks (from > 50 different 16S data sets) that span several ecological environments, ranging from mammalian gut to fresh water habitats. Characterizing these networks based on induced sub-graph (graphlet) spectra reveals that the topology of microbial networks across most ecological habitats is STICKY, a network class that has so far only been reported to accurately describe selected subsets of protein-protein interaction networks. Lastly, implications on the required number of samples as a function of network type/topology will be discussed. For a preprint describing our inference method see: http://arxiv.org/pdf/1408.4158v2.pdf
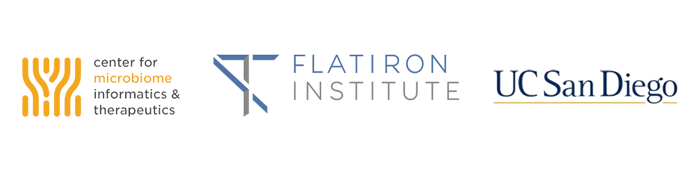