Quantum Cafe Webinar: Lei Wang
Title: Neural Canonical Transformations
Abstract: Canonical transformations play fundamental roles in simplifying and solving physical systems. However, their design and implementation can be challenging in the many-particle setting. Viewing canonical transformations from the angle of learnable diffeomorphism reveals a fruitful connection to normalizing flows in machine learning. The key issue is then how to impose physical constraints such as symplecticity, unitarity, and permutation equivariance in the flow transformations. In this talk, I will present the design and application of neural canonical transformations for several physical problems. Symplectic flow identifies independent and nonlinear modes of classical Hamiltonians and natural datasets. Fermi flow variationally solves ab initio many-electron problem at finite temperature.
February 23, 2021
Lei Wang: Neural Canonical Transformations
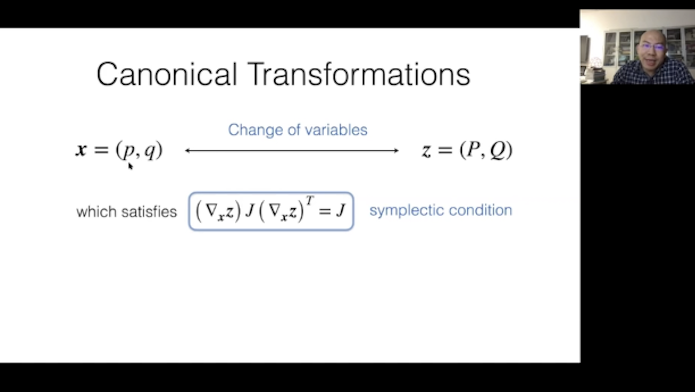
By clicking to watch this video, you agree to our privacy policy.