Flatiron Institute Seminar Series: David Hogg
Title: Machine learning, but structured like physical law
Abstract: Physical laws obey strict symmetries, such as rotational, translational, permutation, and coordinate symmetries, along with restrictions related to dimensions (or units). These can be enforced exactly by requiring models to be written according to geometric and algebraic rules. I demonstrate with toy examples that these ideas can be implemented simply, and that they help with accuracy and generalizability of existing machine-learning methods. More importantly these ideas might have an impact on interpretability, explainability, or symbolic approaches to learning with data. I’ll say a bit about causal structure too, since the strictly symmetric part of a data analysis is often hidden in a latent space that’s only observed indirectly. (Work with Soledad Villar, JHU and others.)
February 11, 2022
Flatiron Institute Seminar Series: David Hogg
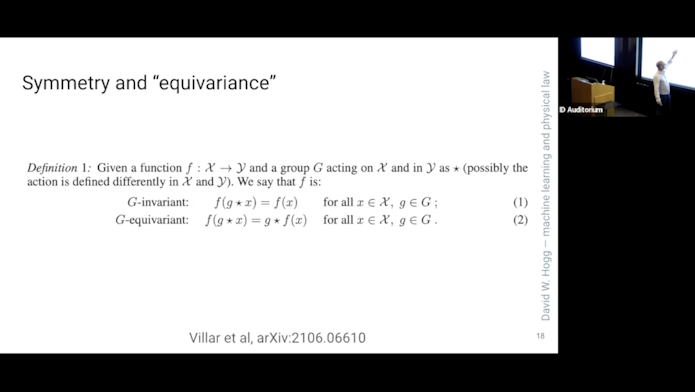
By clicking to watch this video, you agree to our privacy policy.