Simons Investigators
- Email:
[email protected]
Simons Investigators are outstanding theoretical scientists who receive a stable base of research support from the foundation, enabling them to undertake the long-term study of fundamental questions.
The Investigator program has been discontinued.
Simons Investigators in Mathematics, Physics, Astrophysics and Computer Science
The intent of the Simons Investigators in Mathematics, Physics, Astrophysics and Computer Science programs is to support outstanding theoretical scientists in their most productive years, when they are establishing creative new research directions, providing leadership to the field and effectively mentoring junior scientists. A Simons Investigator is appointed for an initial period of five years. Renewal for an additional five years is contingent upon the evaluation of scientific impact of the Investigator.
Simons Investigators in Mathematical Modeling of Living Systems (MMLS)
This program aims to help the research careers of outstanding scientists working on mathematical and theoretical approaches to topics in the life sciences. A Simons Investigator in MMLS is appointed for five years.
Math+X Investigators
This program encourages novel collaborations between mathematics and other fields in science or engineering by providing funds to professors to establish programs at the interface between mathematics and other fields of science or engineering. A Math+X Investigator is appointed for an initial period of five years. Renewal for an additional five years is contingent upon the evaluation of scientific impact of the Investigator.
- Email:
[email protected]
- Email:
[email protected]
2023
Vinod Vaikuntanathan, Ph.D.
Massachusetts Institute of Technology
Vinod Vaikuntanathan’s research is in the foundations of cryptography and its applications to theoretical computer science at large. He is known for his work on fully homomorphic encryption, a powerful cryptographic primitive that enables complex computations on encrypted data, as well as lattice-based cryptography, which lays down a new mathematical foundation for cryptography in the post-quantum world. Recently, he has been interested in the interactions of cryptography with quantum computing, as well as with statistics and machine learning.
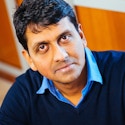
Santosh Vempala, Ph.D.
Georgia Institute of Technology
Santosh Vempala has made fundamental advances in the theory of algorithms: for sampling high-dimensional distributions, computing the volume of a convex body, optimization over convex sets, randomized matrix approximation, as well as basic problems in machine learning. In many cases, these were the first polynomial-time algorithms and co-evolved with insights into high-dimensional geometry and probability. Recent highlights include proving that sufficiently sparse linear systems can be solved faster than matrix multiplication (Ax=b, the workhorse of modern computation); extending sampling methods to non-Euclidean (Riemannian) geometries to make them faster (leading to practical methods in very high dimension); pioneering techniques for algorithmic robust statistics (immune to adversarial corruptions); and developing a rigorous theory of computation and learning in the brain in a biologically plausible model (how does the mind emerge from neurons and synapses?). He continues to be puzzled by whether an unknown polytope can be learned in polytime from samples, whether its diameter is bounded by a polynomial in its description length, whether its volume can be computed in polytime without randomization, and whether the answers to these questions will be discovered by humans or by AI.
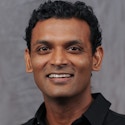
Virginia Williams, Ph.D.
Massachusetts Institute of Technology
Virginia Vassilevska Williams' research is broadly in theoretical computer science, and more specifically in designing algorithms for graphs and matrices, and uncovering formal relationships between seemingly very different computational problems. She is known for her work on fast matrix multiplication, both in co-developing the world's fastest algorithm and in proving limitations on the known techniques for designing matrix multiplication algorithms. Williams is also one of the original founders and leaders of fine-grained complexity, a blossoming new branch of computational complexity.
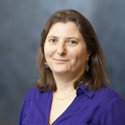
2022
Shayan Oveis Gharan, Ph.D.
University of Washington
Shayan Oveis Gharan’s research exploits deep tools from mathematics, such as the theory of real stable and log-concave polynomials, spectral graph theory and high-dimensional simplicial complexes to design and analyze algorithms for discrete objects. He is known for his results on improved approximation algorithms for classical optimization problems such as traveling salesperson problems, as well as his analysis of the mixing time of Markov chains to efficiently sample from complex probability distributions such as the uniform distribution over the bases of a matroid.
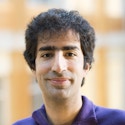
Shachar Lovett, Ph.D.
University of California, San Diego
Shachar Lovett works broadly in theoretical computer science and related mathematics. He focuses on the study of structure and randomness, and how they are pivotal to our understanding of efficient computation. One facet of Lovett’s work is discovering the mathematical structures that underlie efficient computation, such as combinatorial structure in complexity theory, geometric structure in machine learning and algebraic structure in coding theory. Another facet is understanding the power of randomness in computation. Structure and randomness can be seen as complementary, and Lovett’s work aims to identify the fracture lines between structure and randomness, towards a better understanding of computation.
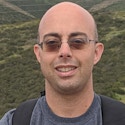
Gregory Valiant, Ph.D.
Stanford University
Gregory Valiant works at the intersection of algorithms, information theory, learning and statistics to understand how to extract as much information as possible from data in various fundamental settings. What information can be inferred if the amount of data is sublinear in the support size or dimensionality of the distribution in question? How do restrictions on the amount of available memory affect the time or amount of data required to learn or optimize? Can we make learning and estimation algorithms robust to outlying training data or settings where the test and training sets are dissimilar?
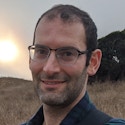
2021
Maria-Florina Balcan, Ph.D.
Carnegie Mellon University
Maria-Florina Balcan’s research spans machine learning, algorithms and algorithmic game theory. She introduced general techniques that helped put modern machine-learning paradigms on solid theoretical foundations, including learning from limited labeled data, distributed learning, noise-tolerant learning and life-long learning. She also provided fundamental contributions to the area of analysis of algorithms beyond the worst-case, by providing both new models of realistic (non worst-case) instances and general techniques for designing and analyzing algorithms derived in a data driven fashion.
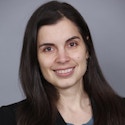
Amit Sahai, Ph.D.
University of California, Los Angeles
Amit Sahai’s research proposes fundamental new concepts in cryptography and establishes new feasibility results. He is best known for his works proposing the notions of indistinguishability obfuscation and functional encryption, and for his recent work establishing the feasibility of indistinguishability obfuscation and functional encryption for general computations based on well-studied hardness conjectures.
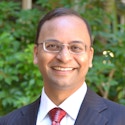
Thomas Vidick, Ph.D.
California Institute of Technology
Thomas Vidick’s research is at the interface of theoretical computer science, quantum information and cryptography. He is known for his work in the theory of quantum interactive proofs, including results on device-independent cryptography, certified randomness and the complexity of quantum multiprover interactive proof systems. The study of quantum entanglement provides a unifying goal behind all these areas and a focal point for his current research.
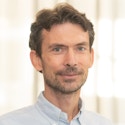
2020
Venkat Guruswami, Ph.D.
Carnegie Mellon University
Venkatesan Guruswami's research has led to major advances in the theory of error-correcting codes, approximate optimization, pseudorandomness and related complexity-theoretic and mathematical aspects. His work on list decoding has yielded codes with minimum possible redundancy for correcting worst-case errors. His recent works include notable progress on polar codes, deletion-correcting codes, codes for cloud storage and constraint satisfaction problems.
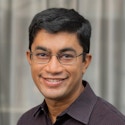
Omer Reingold, Ph.D.
Stanford University
Omer Reingold is the Rajeev Motwani professor of computer science at Stanford University. His research is in the foundations of computer science and most notably in computational complexity, cryptography and the societal impact of computation. Among his fundamental contributions are a small-memory deterministic graph walks, explicit constructions of lossless expander graphs, randomness extractors and pseudorandom functions, as well as establishing influential notions in the area of algorithmic fairness. He is an ACM Fellow, and among his distinctions are the 2005 Grace Murray Hopper Award and the 2009 Gödel Prize.
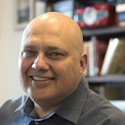
David Woodruff, Ph.D.
Carnegie Mellon University
David Woodruff works in the foundations of data science, specifically in data streams, machine learning, randomized numerical linear algebra, sketching and sparse recovery. One of his breakthroughs was the first randomized relative error approximation algorithms for least squares regression and low rank approximation that run in input sparsity time (i.e., time proportional to the number of non-zero entries in the input). His work on data streams includes the first optimal algorithms for approximately counting distinct elements, approximating the frequency moments and finding heavy hitters, as well as optimal lower bounds for the complexity of certain algorithms.
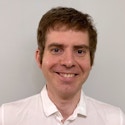
2019
David Blei, Ph.D.
Columbia University
David Blei studies probabilistic machine learning, including its theory, algorithms and application. He has made contributions to unsupervised machine learning for text analysis, approximate Bayesian inference with variational methods, flexible modeling with Bayesian nonparametrics and many applications to the sciences and humanities.
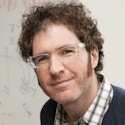
Oded Regev, Ph.D.
New York University
Oded Regev works on mathematical and computational aspects of point lattices. A main focus of his research is in the area of lattice-based cryptography, where he introduced the Learning with Errors (LWE) problem. This problem is used as the basis for a wide variety of cryptographic protocols, including some of the leading candidates for post-quantum secure cryptographic standards. He is also interested in quantum computation, theoretical computer science and, more recently, molecular biology.
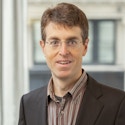
Brent Waters, Ph.D.
University of Texas at Austin
Brent Waters is a leader in the field of cryptography. His pioneering work introduced the concepts of attribute-based encryption and functional encryption. He is known for developing novel proof techniques including lossy trapdoor functions, dual system encryption and punctured programming analysis in cryptographic code obfuscation.
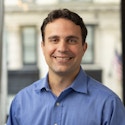
2018
Constantinos Daskalakis, Ph.D.
Massachusetts Institute of Technology
Constantinos Daskalakis works on computation theory and its interface with game theory, economics, probability theory, statistics and machine learning. His work has resolved long-standing open problems about the computational complexity of the Nash equilibrium, the mathematical structure and computational complexity of multi-item auctions, and the behavior of machine-learning methods such as the expectation-maximization algorithm. He has obtained computationally and statistically efficient methods for statistical hypothesis testing and learning in high-dimensional settings, as well as results characterizing the structure and concentration properties of high-dimensional distributions.
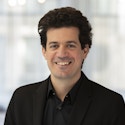
Ran Raz, Ph.D.
Princeton University
Ran Raz’s main research area is computational complexity theory, with a focus on proving lower bounds for computational models. He works on Boolean and algebraic circuit complexity, communication complexity, probabilistically checkable proofs and interactive proof systems. In the last years, he studied relations between communication complexity and information complexity of communication protocols and worked on unconditional lower bounds on the number of samples needed for learning, under memory constraints.
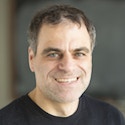
2017
Scott Aaronson, Ph.D.
University of Texas at Austin
Scott Aaronson has established fundamental theorems in quantum computational complexity and inspired new research directions at the interface of theoretical computer science and the study of physical systems.
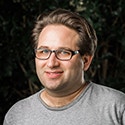
Boaz Barak, Ph.D.
Harvard University
Boaz Barak has worked on cryptography, computational complexity and algorithms. He developed new non-black-box techniques in cryptography and new semidefinite programming-based algorithms for problems related to machine learning and the unique games conjecture.
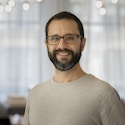
James R. Lee, Ph.D.
University of Washington
James R. Lee is one of the leaders in the study of discrete optimization problems and their connections to analysis, geometry and probability. His development of spectral methods and his work on convex relaxations has led to breakthroughs in characterizing the efficacy of mathematical programming for combinatorial optimization.
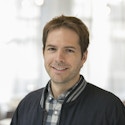
2016
Madhu Sudan, Ph.D.
Harvard University
Madhu Sudan is known for his work in computational complexity theory. He has made fundamental contributions in the areas of probabilistically checkable proofs, nonapproximability of optimization problems and computational aspects of error-correcting codes. More recently, he initiated the study of universal semantic communication.
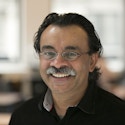
David Zuckerman, Ph.D.
University of Texas at Austin
David Zuckerman is a leader in pseudorandomness and randomness extraction, an area that his early work pioneered. He has a number of beautiful and important results in construction and application of extractors, including applications to coding theory, computational complexity and cryptography, as well as his recent breakthrough result with two-source extractors.
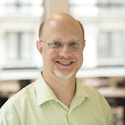
2015
Dan Boneh, Ph.D.
Stanford University
Dan Boneh is an expert in cryptography and computer security. One of his main achievements is the development of pairing-based cryptography, giving short digital signatures, identity-based encryption and novel encryption systems.
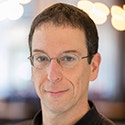
Subhash Khot, Ph.D.
New York University
Subhash Khot initiated a new direction in computational complexity theory and approximation algorithms, based on his Unique Games conjecture, which is currently one of the most important conjectures in theoretical computer science.
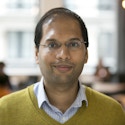
Christopher Umans, Ph.D.
California Institute of Technology
Christopher Umans works on complexity theory, in particular, algorithms and randomness in computations. He has established new upper bounds for the complexity of matrix multiplication and developed a novel algorithm for polynomial factorization.
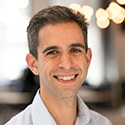
2014
Moses Charikar, Ph.D.
Princeton University
Moses Charikar is one of the world’s leading experts on the design of approximation algorithms. He gave an optimal algorithm for the unique games, a central problem in complexity theory. His work sheds light on the strengths and limitations of continuous relaxations for discrete problems. He has uncovered new obstructions to dimension reduction and compression of geometric data. His algorithms for locality-sensitive hash functions are now de facto standard in real-life applications.
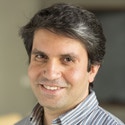
Shang-Hua Teng, Ph.D.
University of Southern California
Shang-Hua Teng is one of the most original theoretical computer scientists in the world, with groundbreaking discoveries in algorithm design, spectral graph theory, operations research and algorithmic game theory. In joint work with Dan Spielman, Shang-Hua introduced smoothed analysis, a new framework that has served as a basis for advances in optimization, machine learning and data mining. His work laid foundations for many algorithms central in network analysis, computational economics and game theory.
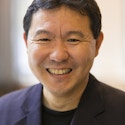
2013
Rajeev Alur, Ph.D.
University of Pennsylvania
Rajeev Alur is a leading researcher in formal modeling and algorithmic analysis of computer systems. A number of automata and logics introduced by him have now become standard models with great impact on both the theory and practice of verification. His key contributions include timed automata for modeling of real-time systems, hybrid automata for modeling discrete control software interacting with the continuously evolving physical environment, and visibly pushdown automata for processing of data with both linear and hierarchical structure such as XML documents.
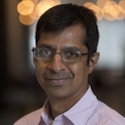
Piotr Indyk, Ph.D.
Massachusetts Institute of Technology
Piotr Indyk is noted for his work on efficient approximate algorithms for high-dimensional geometric problems. This includes the nearest neighbor search, where given a data point, the goal is to find points highly similar to it without scanning the whole data set. To address this problem, he co-developed the technique of locality sensitive hashing, which proved to be influential in many applications, ranging from data mining to computer vision. He has also made significant contributions to sublinear algorithms for massive data problems. In particular, he has developed several approximate algorithms for massive data streams that use very limited space. Recently, he has co-developed new algorithms for the sparse Fourier transform, which compute the Fourier transform of signals with sparse spectra faster than the FFT algorithm.
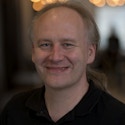
Salil P. Vadhan, Ph.D.
Harvard University
Salil Vadhan has produced a series of original and influential papers on computational complexity and cryptography. He uses complexity-theoretic methods and perspectives to delineate the border between the possible and impossible in cryptography and data privacy. His work also illuminates the relation between computational and information-theoretic notions of randomness, thereby enriching the theory of pseudorandomness and its applications. All of these themes are present in Vadhan’s recent papers on differential privacy and on computational analogues of entropy, which are elegant, impressive, and far-reaching.
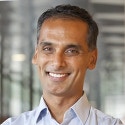
2012
Sanjeev Arora, Ph.D.
Princeton University
Sanjeev Arora has played a pivotal role in some of the deepest and most influential results in theoretical computer science. He started his career with a major contribution to the proof of the PCP theorem, widely regarded as the most important result in complexity theory in the last 40 years. The PCP theorem states roughly that every proof, of any length, can be efficiently converted into a special format, in which correctness can be verified with high probability by reading small parts of it. The PCP theorem revolutionized our understanding of optimization problems and opened new directions in coding, cryptography and other areas. Arora is also known for his breakthroughs in approximation algorithms, having solved longstanding open problems. Notable examples include his algorithms for the Euclidean traveling salesman problem and for the sparsest cut in a graph. Arora has made important contributions on many other topics, including the unique games conjecture (a conjectured strengthening of the PCP theorem) and the power and limitations of hierarchies of linear and semidefinite programs.
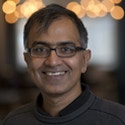
Shafrira Goldwasser, Ph.D.
Massachusetts Institute of Technology
Shafi Goldwasser has had tremendous impact on the development of cryptography and complexity theory. Starting with her thesis on “semantic security”, she laid the foundations of the theory of cryptography. She created rigorous definitions and constructions of well-known primitives such as encryption schemes (both public and private key versions) and digital signatures, and of new ones that she introduced, such as zero-knowledge interactive proof systems invented with Micali and Rackoff. Continuing her work on interactive proofs which allow a probabilistic polynomial time algorithm to verify mathematical proofs via interaction with a powerful prover, Shafi and her co-authors extended the notion of interactive proofs to two-prover systems. The original motivation was cryptographic, but they turned out to be of great significance in complexity theory, paving the way to the equivalent formulation of PCP (probabilistically checkable proofs). The expressive power of two-prover systems is huge (non-deterministic exponential time). furthermore, Shafi and her co-authors showed the connection between a scaled down variant of these systems and the hardness of approximation results for NP-hard problems, which led to the PCP theorem. On the algorithmic front, a problem of great significance is that of recognizing (and generating) prime numbers. Shafi and Kilian designed efficient probabilistic primality provers, which output short proofs of primality, based on the theory of elliptic curves. Together with Goldreich and Ron, Shafi originated the field of combinatorial property testing, devising a class of sub-linear algorithms to test properties in dense graphs.
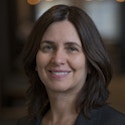
Russell Impagliazzo, Ph.D.
University of California, San Diego
Russell Impagliazzo has made many deep contributions to cryptography and complexity theory. Russell and collaborators showed that one-way functions exist if and only if pseudorandom generators exist. In other words, one can generate sequences of bits for which it is computationally hard to predict the next bit with accuracy much better than random guessing if and only if there are easy-to-compute functions that are hard to invert on the average. Russell also showed that there are worlds in which certain cryptographic primitives are strictly inequivalent. For example, there are worlds where one-way functions exist but public-key encryption is not possible. One of Russell’s major contributions in complexity theory is the exponential-time hypothesis and its implications. The hypothesis states that there are problems where it is hard to speed up the brute-force solution even by a small amount. Russell helped establish the first complete problem for this class. In joint work with Avi Wigderson, Russell showed that if there are problems in exponential time that require exponential-sized circuits to solve, then any efficient algorithm that uses randomization has an equivalent, efficient one that does not.
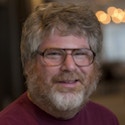
Jon Kleinberg, Ph.D.
Cornell University
Jon Kleinberg is noted for his creativity, intellectual ability, research scholarship, diversity of research interests and the impact of his work. He is best known for his contributions in establishing the computational foundations for information retrieval and social networks. His information retrieval work includes the use of link analysis (e.g., hubs and authorities) for ranking, classification and identifying web communities, the web as a graph, and understanding the success of latent semantic analysis. His work in algorithmic social networks (a field that he can be said to have started) includes the understanding of “small worlds” and decentralized search, analysis of bursty streams and influence spread in social networks. Kleinberg has done work in many other fields, including approximation algorithms, communications networks, queuing theory, clustering, computational geometry, bioinformatics, temporal analysis of data streams, algorithmic game theory, online algorithms and distributed computing. His influence is augmented by popular papers in Science and Nature and by two widely used texts, one with Tardos, Algorithm Design, and one with Easley, Networks, Crowds, and Markets: Reasoning about a Highly Connected World.
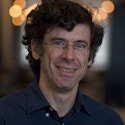
Daniel Spielman, Ph.D.
Yale University
Daniel Spielman’s work has been important to three distinct research communities: theoretical computer science, applied mathematics, and operations research. His work on smoothed analysis of linear programming provides mathematical justification for why the simplex method to solve problems works well in practice even though worst-case analysis shows that there are instances in which it takes exponential time. A small random perturbation converts any linear programming instance into one that, with high probability is solved efficiently by the simplex algorithm. Similar perturbation results hold for many other problems and provide an alternative to worst-case analysis, which may be too pessimistic. His codes based on expander graphs achieve near-optimal rate and nearly linear time encoding and decoding algorithms. In joint work with Teng, Spielman gave a method of preconditioning a Laplacian matrix A, which yields a near-linear-time algorithm for solving the system Ax = b. This leads to highly efficient algorithms for circuit analysis and network flow computations.
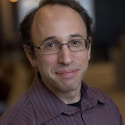