Jacob Bien, Ph.D.
Associate Professor of Data Sciences and Operations, Marshall School of Business, University of Southern CaliforniaJacob Bien’s website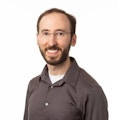
CBIOMES Project: Statistical learning for marine ecosystems
Oceanographers have assembled a wide range of techniques for measuring marine ecosystems. Having many different data types, or “views,” of these ecosystems promises to provide a more nuanced picture of the inner workings of the complex underlying systems. However, realizing this promise requires statistical methods that can reliably integrate these myriad data sets together in a principled, reproducible and computationally feasible way that will highlight important relationships. We focus on developing new statistical methodology in collaboration with oceanographers in CBIOMES.
Our work revolves around three main data types: (1) flow cytometry data; (2) taxonomically structured microbial amplicon data; and (3) environmental data (especially those collected via satellite). Each of the planned projects involves the integration of a pair of these data types. For example, one project is focused on using measured environmental factors to predict what phytoplankton subpopulations will be measured via flow cytometry on a cruise (and to predict these subpopulations’ relative abundances). Another project is focused on connecting flow cytometry data and microbiome amplicon data, which are two different ways of measuring the microbes present in an environment. Finally, a third project involves data-adaptive, taxonomy-based aggregation of amplicon data based on environmental covariates. Each of these projects will involve development of new statistical methodology, data-focused collaboration and implementation and distribution of open-source software.
Bio:
Jacob Bien is an associate professor in the Department of Data Sciences and Operations in the Marshall School of Business at the University of Southern California (USC). He received a B.S. in physics and a Ph.D. in statistics from Stanford University. Before joining USC, he was an assistant professor at Cornell University in the Department of Biological Statistics and Computational Biology and in the Department of Statistical Science. Bien’s research focuses on statistical machine learning and in particular the development of novel methods that balance flexibility and interpretability for analyzing complex data. He combines ideas from convex optimization and statistics to develop methods that are of direct use to scientists and others with large datasets. Particular areas of focus include variable selection, clustering, prototype selection and the modeling of dependence in high-dimensional data. His work has been supported by the National Science Foundation, both through a CAREER award and through a grant on high-dimensional covariance estimation, the National Institutes of Health, and the Simons Foundation. He serves as an associate editor of Biometrika and the Journal of Computational and Graphical Statistics and holds a Dean’s Associate Professorship in Business Administration at USC.