Julia W.P. Hsu, Ph.D.
Professor, Erik Jonsson School of Engineering and Computer Science, University of Texas at DallasJulia W.P. Hsu’s website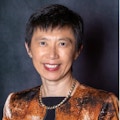
Julia W.P. Hsu is a professor of materials science and engineering in the Erik Jonsson School of Engineering and Computer Science at the University of Texas at Dallas (UTD) and holds the Texas Instruments Distinguished Chair in Nanoelectronics. She received her B.S.E. degree in chemical engineering from Princeton University, her M.S. and Ph.D. degrees in physics from Stanford University and did her postdoctoral training at Bell Labs. Before UTD, she worked at the University of Virginia, Lucent Bell Labs and Sandia National Laboratories.
Hsu’s research focuses on nanoscale materials physics on a wide range of materials, from superconductors to organic semiconductors. She has done extensive work on the electronic and photonic properties of materials and devices, in particular, the relationship between the microscopic atomic organization and macroscopic properties of complex materials consisting of dissimilar components such as organics and inorganics. Her current research activities include solution synthesis of metal oxides, physics and application of emergent solar cells, flexible printed electronics, and materials processing using photons. Hsu has over 230 publications, five patents and has given over 200 invited presentations at international meetings, universities and research institutions.
Hsu is a fellow of the American Physical Society (APS), the American Association for the Advancement of Science and the Materials Research Society (MRS). She is an Honorary International Chair Professor at Taipei Tech and was awarded a Hertz Foundation Fellowship, APS Apker Award, a National Science Foundation Young Investigator Award and a Sloan Foundation Research Fellowship. Her professional society services include MRS (meeting chair, board of directors, treasurer, operational committee chair), APS (Division of Materials Physics executive committee, award committee) and Electronic Materials Conference (organizer). She also served on the DOE Basic Energy Sciences advisory committee, National Academies review panels and numerous external advisory committees.
Merging machine and human intelligence for energy materials research
Julia Hsu is an experimental materials physicist who has studied materials physics phenomena using traditional approaches such as performing optimization by varying one variable at a time (OVAT). In materials research, optimization is ubiquitous, but the outcomes are typically affected by a multitude of variables. Furthermore, the various input parameters have complex and non-linear interrelationships, creating a combinatorically vast space of possible experimental conditions that cannot be exhaustively tested in practice since performing experiments is time-consuming and expensive.
Hsu’s Pivot Fellowship discipline will be machine learning (ML), which is at the intersection of mathematics, computer science and statistics, and is now used in many aspects of modern life, e.g., email spam filtering, shopping product recommendation or speech recognition. Bringing ML into materials synthesis and processing can guide expensive experiments to make the most effective use of limited resources and elucidate the complex interdependence among the variables.
During the fellowship, Hsu’s goals are to become proficient in ML approaches and apply them to optimization problems in materials synthesis and processing, particularly energy materials. Her self-learning so far has highlighted big challenges in becoming truly versed in the new discipline, including the different vocabularies and the wide variety of ML frameworks. The Pivot Fellowship offers an invaluable opportunity for me to be embedded in the research group of her mentor, Tonio Buonassisi at MIT, and learn how to effectively apply ML techniques to energy materials research. Not only will the mentorship shorten the time required for her to become proficient in the new field, but it will also minimize the possibility of going in erroneous directions were she on her own. Conversely, she brings domain expertise in diverse materials including polymers, metal oxides, semiconductors and hybrid materials, that will advance ML beyond treating all problems with the same “black box” approach. Furthermore, she believes that it would be more productive to augment the machine with materials science knowledge, physics-based rules, and prior experimental data, instead of “cutting humans out of the loop.”