Laura Driscoll, Ph.D.
Postdoctoral Fellow, Stanford University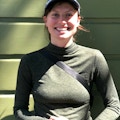
Laura Driscoll is a postdoctoral fellow at Stanford University where she works in the lab of David Sussillo and Krishna Shenoy. She has a bachelor of science in chemistry from the University of California, Berkeley and a Ph.D. in neuroscience from Harvard University. As a graduate student in the lab of Christopher Harvey, Driscoll studied the long-term reorganization of neuronal dynamics in the parietal cortex of mice performing decision-making tasks. Her current research focuses on flexible computation for context switching and throughout learning and memory. Her approach is to build artificial network models and statistical tools to better understand the constraints on networks that perform computation. Driscoll plans to start a joint theoretical research group with her collaborator, Lea Duncker. This structure of team-based community science will expand both the breadth of scientific questions their joint-group can approach, as well as their collective bandwidth for continued engagement addressing oppressive cultural practices in academia.
Project: Learning and consolidation of dynamical systems that perform computation
Flexible computation is a hallmark of intelligent behavior. Yet, little is known about how neural networks contextually reconfigure for different computations and throughout learning. The ultimate goals of our lab are to: (1) discover strategies that overcome computational constraints for lifelong learning through the study of artificial systems, (2) test which strategies are implemented in biological systems, and (3) improve statistical tools for reverse-engineering artificial systems for further hypothesis development. This work will guide experimental predictions for both behavior and neural population recordings. To test these hypotheses, we will conduct human psychophysics experiments in our own lab and collaborate with systems neuroscience labs measuring brain-wide optical and electrical data, working on an array of model systems. Extending the computation through dynamics approach from isolated tasks after learning to systems that continuously evolve in the context of multiple tasks will give us a framework to address learning and memory from a novel perspective.