Pixelated Movies Yield Faster Way to Image Many Neurons
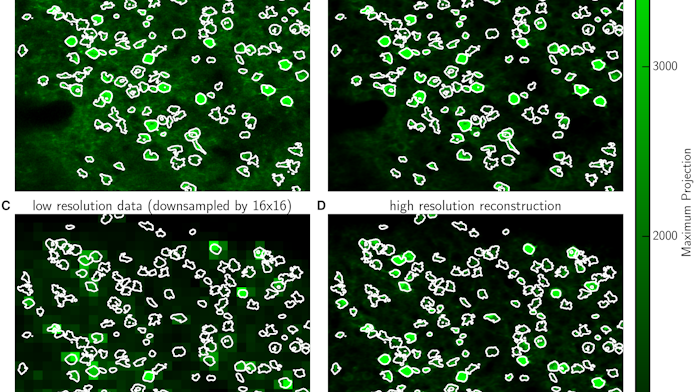
Optical imaging techniques, such as calcium imaging, have revolutionized our ability to track the activity of hundreds of neurons simultaneously. These methods yield huge movie files of twinkling neurons, but analyzing the vast amount of data is often slow and computationally intensive.
“Calcium imaging is one of the best methods we have now for recording from as many cells as possible simultaneously, but extracting useful signals from these giant movies is a big issue,” says Liam Paninski, a statistician at Columbia University and an investigator with the Simons Collaboration on the Global Brain. “We want to jointly optimize optical parameters and the computational methods we apply to extract as much information from the brain as possible.”
Paninski and his collaborators have developed two new techniques that dramatically speed up analysis without sacrificing important information. That makes it possible to image larger numbers of neurons or to enhance temporal or spatial resolution. The research was published in PLoS Computational Biology in August.
In the early days of calcium imaging, scientists would label individual cells by hand and then track each neuron’s activity over time. Over the past few years, researchers have developed automated ways to annotate and analyze imaging data. Paninski’s team showed that compressing the movie before analysis — basically, averaging every five frames — dramatically speeds up the process without sacrificing accuracy.
In a second new technique, the researchers developed a two-stage recording process that makes it possible to record movies at a much lower spatial resolution while still tracking the activity of single cells. At the start of an experiment, scientists use high-resolution imaging to log the location and shape of individual cells. With this information in hand, they can then switch to a much lower spatial resolution for the rest of the experiment.
The resulting movie becomes much more pixelated, making individual cells difficult to resolve with the naked eye. “But because you know the shapes of the cells, you can still extract the information accurately,” Paninski says. “By recording many fewer pixels per second, you can spend the pixel budget over a larger field of view or to image cells over a faster frame rate.”
Both methods use a technique called matrix factorization, in which the imaging movie is converted into a two-dimensional matrix. Scientists then apply standard computational tools to break the matrix into simpler parts. Paninski’s group and others, including researchers at the Simons Foundation’s new Flatiron Institute, have been developing these methods over the past couple of years. These methods are available to the neuroscience community through CaImAn, a software toolbox for calcium imaging data analysis developed by members of the Flatiron Institute Neuroscience group.
Scientists interested in using these tools can access them on GitHub. The researchers have already incorporated the first technique into the software and are working with other groups to develop versions for different types of optical imaging.
Paninski, in collaboration with Eftychios Pnevmatikakis and Andrea Giovannucci at the Flatiron Institute, are now using these techniques to try to analyze calcium imaging in real time, which will make it easier to optimize experiments on the fly. Currently, scientists process their data after the experiment, meaning they can’t diagnose potential problems in real time. Real-time analysis could also enable new types of experiments, allowing scientists to focus on a specific type of neural signal or to stimulate a population of neurons. “If you want to perturb ongoing neural dynamics, you need to be able to close the loop,” Paninski says.
Moreover, this approach might expand the reach of brain-computer interface experiments, in which brain activity is used to control a computer cursor or other output. Existing brain-computer interface experiments typically use multi-electrode arrays. “If we can do this with optical imaging,” Paninkski says, “it opens a whole new kind of experiment that we wouldn’t be able to do otherwise.”