Neural ‘Recycling’ Key to Short-Term Learning
Credit: Melissa Neely
When you’re driving a new car with touchier brakes or more responsive steering, you quickly learn to adjust, gently tapping the brake or turning the wheel. How does the brain adapt to these novel conditions? New research shows that in the short term — over the course of a few hours — animals repurpose existing neural activity patterns when learning a new task rather than create entirely new patterns. The findings suggest that biological factors constrain how the brain learns over short timescales, limiting the repertoire of patterns that groups of neurons can produce.
Byron Yu, a neuroscientist at Carnegie Mellon University, and collaborators studied the link between neural activity and behavior using a brain-computer interface, a device that converts activity recorded directly from groups of neurons into an action, such as the movement of a robotic arm or a cursor on a computer screen. Scientists are developing these kinds of interfaces to help paralyzed people control robotic limbs.
But Yu points out that brain-computer interfaces can also help researchers address basic neuroscience questions by simplifying the complex brain-behavior circuit. The ability to reach for a cup of coffee depends on an array of factors — the activity of thousands of neurons that underlie movement, ongoing sensorimotor feedback, and other variables. But scientists don’t know the algorithm the brain uses to convert activity to movement. With a brain-computer interface, researchers delineate an algorithm, or mapping, that converts neural activity from a defined set of cells into movement. They can then change that mapping and study what happens as the animal learns to adjust.
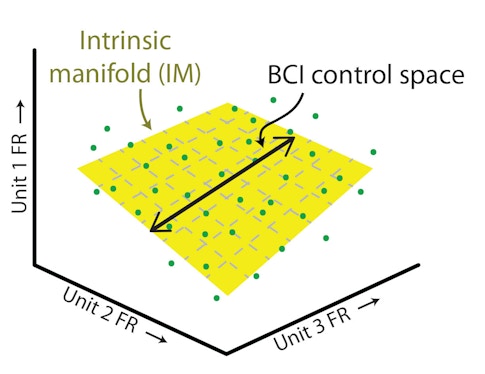
In the new study, Yu, Matthew Golub, Steven Chase, Aaron Batista and other collaborators trained monkeys to move a cursor on a computer screen using a brain-computer interface that records activity from 90 neurons in the motor cortex. (The number of neurons is determined by the recording device.) They then mapped the activity of individual neurons against each other, creating a population activity space where each axis represents the activity of one neuron. A three-dimensional version of neural activity space is shown here. Yu and others have previously shown that neural activity doesn’t have free rein in this space. “It lies in a lower-dimensional space, the intrinsic manifold, that presumably reflects constraints imposed by the underlying circuitry,” Yu says.
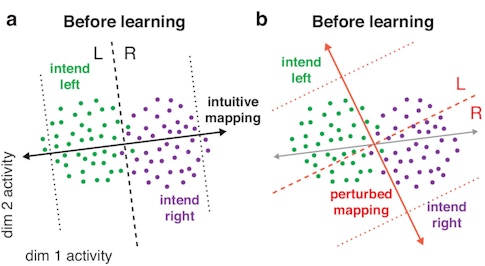
The researchers used an approach called factor analysis to identify 10 factors that represent that manifold and created a neural activity-to-movement mapping that lies within it. For example, to move the cursor to the left, the monkey might have to shift neural activity to a point in the left half of the neural activity space. To move the cursor right, the monkey might have to shift neural activity to the right. With practice, animals can learn to control the cursor quite effectively. But when researchers change the mapping, the same activity patterns are no longer effective (a and b).
In previous research, Yu, Batista and collaborators showed that certain types of mappings are easier to learn. Animals more quickly adapt to a within-manifold mapping, where neural activity patterns within the existing manifold are tied to different movements. Outside-manifold mapping, where animals have to generate new activity patterns beyond the existing manifold, are more challenging. “That implies the structure of the network, [which defines the manifold], can shape learning,” Yu says.
In the new study, published in Nature Neuroscience and presented at the Cosyne meeting in Denver in March, the researchers wanted to better understand how neural activity changes with learning, specifically examining how neural activity evolved during within-manifold remapping. The activity patterns that occur within the manifold are called the neural repertoire.
The team first outlined different strategies that the monkey might use and predicted how each would alter the neural repertoire. The optimal way to learn the new mapping would be via a strategy called realignment — the animal’s neural activity patterns would shift to optimally accommodate the new mapping. This method generates activity patterns outside of the original neural repertoire. With a second approach, rescaling, the contribution of individual dimensions to the neural activity patterns shifts. A third method, reassociation, repurposes activity patterns within the existing repertoire. In other words, the animal might use the pattern of activity that previously corresponded to leftward movement to move the cursor upward.

To determine which model the monkeys actually use, researchers analyzed how the neural repertoire would shift in each case and how each model would alter performance, comparing results to the experimental data. “They set up these hypotheses in a very formal way and did a lot of work to determine which looks more like the data,” says Valerio Mante, a neuroscientist at the University of Zurich and SCGB investigator who was not involved in the study. “It’s a nice example of what you get by using sophisticated math to try to understand neural responses.”
The researchers found that the neural repertoire remained largely unchanged after remapping, suggesting that reassociation best explains learning. The animals’ performance supports that conclusion as well. Realignment and rescaling predict that the monkeys would perform better after the remapping. But in reality, they perform worse, as predicted by reassociation. “Learning is not only constrained by the intrinsic manifold; it’s further constrained by a neural repertoire,” Yu says.
The findings show that in the short term, learning is suboptimal, which surprised the researchers. “I think what the animal is doing is even more sophisticated than what we thought,” Batista says. “Reassociation seems like the hardest option, but that must be because changing the network is even harder.”
The study also reflects the shift in the field from looking at single neurons to examining populations of neurons. “All of these hypotheses live in the space of population responses,” Mante says. “The whole conceptual framework has moved to the population level.”
Mante says the results make sense in the context of the theory that the motor cortex acts as a dynamical system, meaning its dynamics unfold based on a set of initial conditions or inputs. “Different initial conditions lead to different dynamics, which lead to different movements,” Mante says.
With reassociation, the dynamics of the system can remain the same, while the initial conditions — or remapping — change. “The set of trajectories don’t need to change; you just need to change how they are related to different targets,” Mante says. In that context, reassociation is simpler than the other hypotheses, he says.
Yu and collaborators are now looking at how learning changes neural activity over longer time frames, such as several days. “We know that different types of learning take place at different timescales and involve different mechanisms,” Batista says. Preliminary results show that given enough time, the brain can overcome the constraints observed in the short term, generating new activity patterns outside of the original manifold.