Simple Networks Generate Complex Dynamics
Millions of research dollars pour into so-called ‘connectome projects,’ in which researchers seek to map the brain’s vast connectivity at various levels of detail. For instance, the FlyEM project at the Howard Hughes Medical Institute’s Janelia Farm Research Campus charts individual connections in the fruit fly. The Allen Institute for Brain Science has developed several atlases of the mouse brain. And both the National Institutes of Health and the European Research Council have human brain mapping projects underway.
Such efforts have sparked a heated debate among neuroscientists as to whether it’s wise to divert so many scientific resources to these massive projects. After all, neuroscientists have known the complete wiring diagram for one organism, the humble worm Caenorhabditis elegans, since 1986. And yet no one can predict how an individual worm will behave. So how important is it to understand the exact wiring diagram of the brain? Do scientists need to know the details of every single connection? Can connectivity alone predict how a circuit will behave?
New mathematical findings suggest that the precise way neurons are connected could be crucial for understanding circuit behavior. Carina Curto, a mathematician at Pennsylvania State University, has found that minor changes in the structure of simple networks can trigger major changes in activity. Curto presented her work at the Computational and Systems Neuroscience conference (Cosyne) in Denver, Colorado, in March.
“The central problem for me is how the connectivity of a network affects its dynamics,” Curto says. “You can tackle that problem at many levels. It’s a neuroscience problem. It’s a biology problem. But it’s also a math problem.”
Curto, along with her collaborator Katie Morrison of the University of Northern Colorado, set out to determine the mathematical rules governing ‘combinatorial threshold linear networks,’ or CTLNs. CTLNs are mathematical models in which the network’s ‘nodes’ represent neurons, each described by the same simple nonlinear equation. Curto chose how many nodes appeared in the networks and how they were connected. She then set the mathematical model into motion, taking note of whatever kind of activity arose. To keep things simple, she started out with tiny networks of, say, just five or nine nodes.
She found that a network might pulse rhythmically when wired up one way but behave chaotically when wired up in a slightly different fashion. Sometimes, a node would drop out of the action completely. Even rudimentary networks wired up according to the same rules could show wildly different activity. “I had complete and total knowledge of the network, and yet it was still really difficult to look at it ahead of time and predict what was going to happen, dynamically,” Curto says.
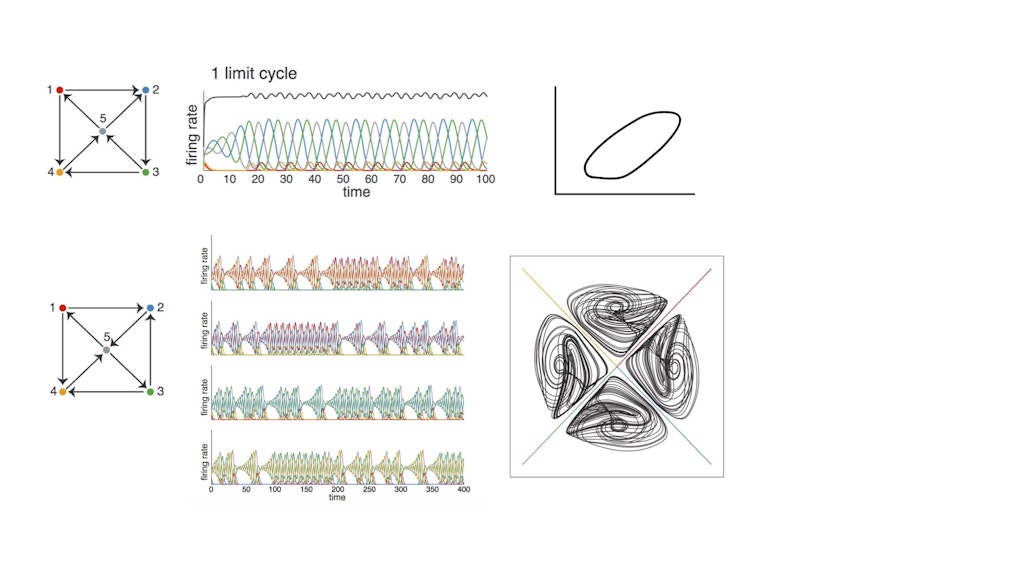
That counterintuitive finding was striking to Linda Wilbrecht, Cosyne’s co-chair and a biologist at the University of California, Berkeley who studies brain circuit development and plasticity. “As a biologist and an anatomist, I’m really focused on where cells project and how they form synapses, but until I saw her talk I didn’t fully appreciate how such a simple network could have such different properties with very small changes in connectivity,” Wilbrecht says.
By systematically varying the connections in these tiny networks, Curto found mathematical principles to describe their behavior. These fundamental insights allowed her to predict a network’s activity from its structure and to derive theorems that govern the behavior of CTLNs with hundreds — or even billions — of nodes.
Curto cautions her results aren’t meant to be taken as literal biological principles. Real neurons behave in diverse ways and can’t be accurately described by the same simple nonlinear equation. “I don’t expect rules this simple to hold up in any real neural circuit,” she says. “This is not a model of the brain. It’s a toy model that’s inspired by the brain, where we can develop some math.”
Curto’s work is “very powerful,” says Maneesh Sahani, a neuroscientist at University College London and an investigator with the Simons Collaboration on the Global Brain. But he cautions that substantial work would need to be done to extend her results to large networks in the brain, such as those in the mammalian cerebral cortex, where her mathematical assumptions may not hold. “One might wonder how easy it will be to take these sorts of mathematical ideas and make sense of very large networks with connectivity that we think is dictated by statistical or slightly random principles,” he says. “But again, you can’t even start until you’ve got the mathematics for these basic systems that she’s analyzed. So it’s clearly progress.”
Sahani thinks Curto’s work might be more immediately relevant to small, stereotyped circuits in invertebrates, such as the crab stomatogastric ganglion. He points to the work of Eve Marder and others who have shown that small tweaks in simple biological networks can give rise to very different neuronal firing patterns.
Indeed, Curto’s findings suggest that exact circuit diagrams are important — but they’re not enough. The field will need mathematical models to interpret them. Curto says she’s not surprised that mapping the C. elegans connectome has yet to yield a predictive model of the organism. “Even with six neurons, it’s hard to predict what’s going to happen from the connectome,” she says. “But my work is not just on exposing how difficult this problem is — I’m also trying to tackle it.”
Though Curto’s research might not directly apply to large, complex networks, the overall lessons might be relevant for large-scale mapping efforts. The European Union’s $1.3-billion Human Brain Project, for example, aims to simulate the brain on a supercomputer. Because scientists don’t know the human brain’s exact wiring diagram, they infer ‘local connectivity statistics,’ which describe how likely it is that any two neurons are connected, from experimental data. For example, modelers might decide that cortical neurons of a particular type have a 20 percent chance of connecting to neurons of the same type if they are within one millimeter of each other.
Curto’s results sound a note of caution for these simulations, even if they are based on impeccable experimental work. “You can’t just say local connectivity matches up and so my model is going to be faithful to what happens in the brain,” she says. “Small differences in global connectivity, even with the correct local connectivity statistics, could have a dramatic effect.”