Power Law Discovery May Explain Why You Can See the Forest and the Trees
The activity of neuronal populations in the visual cortex falls into a sweet spot that helps us pay attention to both the forest and the trees, according to new research by Kenneth Harris and Matteo Carandini at University College London, investigators with the Simons Collaboration on the Global Brain, and their collaborators. Harris presented the research at the Cosyne conference in Lisbon, Portugal, in March; the work was also published in Nature in June.
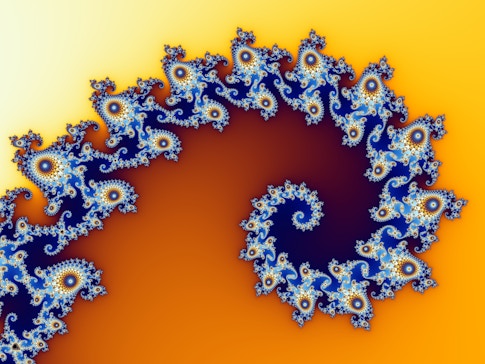
As scientists record from an increasingly large number of neurons simultaneously, they are trying to understand what structure the activity pattern takes and how that structure relates to the population’s computing power. A number of studies have suggested that neuronal populations are highly correlated, producing low-dimensional patterns of activity. That observation is somewhat surprising, because minimally correlated groups of neurons would be able to transmit more information. (For more on this, see Predicting Neural Dynamics From Connectivity and The Dimension Question: How High Does It Go?)
In the new study, Carsen Stringer and Marius Pachitariu, now researchers at the Janelia Research Campus in Ashburn, Virginia, and previously a graduate student and postdoctoral researcher, respectively, in Harris’ lab, used two photon microscopy to simultaneously record signals from 10,000 neurons in the visual cortex of mice looking at nearly 3,000 natural images. Using a variant of principal component analysis to analyze the resulting activity, the researchers found it was neither low-dimensional nor completely uncorrelated. Rather, neuronal activity followed a power law in which each component explains a fraction of the variance of the previous component. The power law can’t simply be explained by the use of natural images, which have a power-law structure in their pixels (neighboring pixels tend to be similar). The same pattern held for white-noise stimuli.
Researchers used a branch of mathematical analysis called functional analysis to show that if the size of those dimensions decayed any slower, the code would focus more and more attention on smaller and smaller details of the stimulus, losing the big picture. In other words, the brain would no longer see the forest for the trees. Using this framework, the researchers predicted that dimensionality decays faster for simpler, lower-dimensional stimuli than for natural images. Experiments showed this is indeed the case — the slope of the power law depends on the dimensionality of the input stimuli. Harris says the approach can be applied to different types of large-scale recordings, such as place coding in the hippocampus and grid cells in the entorhinal cortex.
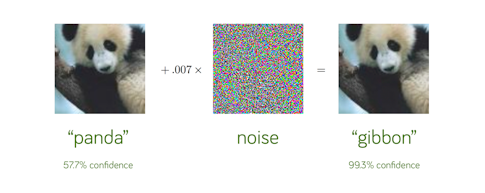
Artificial neural networks don’t seem to follow this type of power law, which Harris says may explain a puzzling flaw they have, known as an adversarial attack. Highly trained networks excel at image recognition, correctly identifying cats in a variety of different shapes, colors, poses and contexts, for example. But adding a sprinkling of noise to a photo can interfere with the network’s ability to categorize it. In one example, a network identified a photo of a panda with 58 percent confidence. Slightly distorting the image, to a level almost imperceptible to a human observer, wreaked havoc on the network’s recognition capability — it predicted the photo to be a gibbon with 99.3 percent confidence. Harris proposes that the brain’s power law protects it from this type of error by helping it avoid over-focusing on small details. Finding a way to engineer that mechanism into artificial neural networks may solve the problem, he says.