
The Largest Suite of Cosmic Simulations for AI Training Is Now Free to Download; Already Spurring Discoveries
Totaling 4,233 universe simulations, millions of galaxies and 350 terabytes of data, a new release from the CAMELS project is a treasure trove for cosmologists. CAMELS — which stands for Cosmology and Astrophysics with MachinE Learning Simulations — aims to use those simulations to train artificial intelligence models to decipher the universe’s properties.
Scientists are already using the data, which is free to download, to power new research, says project co-leader Francisco Villaescusa-Navarro, a research scientist with the Simons Foundation’s CMB (Cosmic Microwave Background) Analysis and Simulation group.
“The data will enable new discoveries and connect cosmology with astrophysics through machine learning,” says Villaescusa-Navarro, who leads the project with associate research scientists Daniel Anglés-Alcázar and Shy Genel of the Flatiron Institute’s Center for Computational Astrophysics (CCA). “There has never been anything similar to this, with this many universe simulations.”
The CAMELS team generated the simulations using code taken from the IllustrisTNG and Simba projects. The CAMELS team includes members of both projects, with Genel a part of the core team of IllustrisTNG and Anglés-Alcázar on the team that developed Simba.
About half of the simulations combine the physics of the cosmos with the smaller-scale physics essential for galaxy formation. Each simulation is run with slightly different assumptions about the universe — for instance, regarding how much of the universe is invisible dark matter versus the dark energy pulling the cosmos apart, or how much energy supermassive black holes inject into the space between galaxies.

The researchers designed the simulations to feed machine-learning models, which will then be able to extract information from observations of the real, observable universe. With 4,233 universe simulations, CAMELS is the largest ever suite of detailed cosmological simulations designed to train machine-learning algorithms.
“Machine learning is revolutionizing many areas of science, but it requires a huge amount of data to exploit,” says Anglés-Alcázar, who is also an assistant professor at the University of Connecticut. “The CAMELS public data release, with thousands of simulated universes covering a broad range of plausible physics, will provide the galaxy formation and cosmology communities with a unique opportunity to explore the potential of new machine-learning algorithms to solve a variety of problems.”
The CAMELS dataset is already powering research projects, with a wide range of papers utilizing the data in the works.
Pablo Villanueva-Domingo of the University of Valencia in Spain led one such paper. He and his colleagues leveraged the CAMELS simulations to train an artificial intelligence model to measure the mass of our Milky Way galaxy plus its surrounding dark matter halo, and the nearby Andromeda galaxy and its halo. The measurements — the first ever done using AI — put our galaxy’s heft at 1 trillion to 2.6 trillion times the sun’s mass. Those estimates are roughly in line with those made by other methods, demonstrating the AI approach’s accuracy.
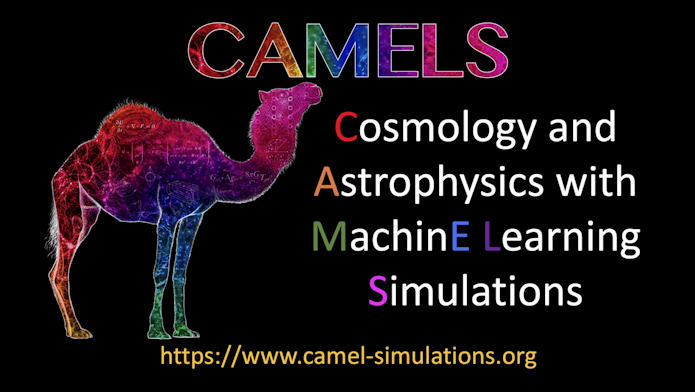
By clicking to watch this video, you agree to our privacy policy.
Meanwhile, Villaescusa-Navarro headed an effort to use the CAMELS data to estimate the value of two parameters that govern the fundamental properties of the universe: what fraction of the universe is matter, and how evenly mass is distributed throughout the cosmos. First, he and his colleagues used CAMELS to generate maps such as the distribution of dark matter, gas and different properties of stars. Then, using the maps, they trained a machine-learning tool called a neural network to predict the values of the two parameters.
“This is the same kind of algorithm used to tell the difference between a cat and a dog from the pixels of an image,” says Genel, who co-authored the paper. “The human eye can’t determine how much dark matter there is in a simulation, but a neural network can do that.”
The results showed the promise of leveraging CAMELS to precisely estimate such parameters in the future based on new observations of the universe, says Villaescusa-Navarro.
“These papers have done all this science that is very different from each other,” Villaescusa-Navarro says. “You can use the CAMELS data to weigh our own galaxy, use the signatures of mysterious fast radio bursts to learn about cosmology and astrophysics, or learn universal properties of subhalos” — smaller clumps of dark matter contained in larger dark matter halos.
“It’s exciting to see what other new discoveries this will enable,” he says.