
Simons and NSF-Supported Research Centers Explore the Symbiotic Relationship Between Mathematics and Biology
If biology were like mathematics, our fates would be predetermined: Every cell division, organism, individual and population would follow logically from basic truths, or axioms. Happily, biology doesn’t exactly follow the science of patterns and order — mutations, variance and random chance have led to a rich, diverse field of biological research.
That being said, math does permeate the living world. From the famously logarithmic curve of a nautilus shell to the echoing periodicity of predator and prey populations, evidence of mathematics can be found in nearly every biological process. But historically, the two fields evolved along different paths. Mathematics consists of statements which follow from axioms, and applications of mathematical research often follow decades, or even centuries, later. Biology started and continues to focus on observable phenomena, with no corresponding biological axioms, or ‘first principles.’
But in recent years, that gap has begun to shrink. Mathematical biology — or the use of mathematics within biology — has emerged as its own interdisciplinary field, with implications in the ‘pure’ fields of both biology and mathematics.
“For the last few decades, there was a renaissance and redefining what it meant to be a mathematical biologist: Was it simply applying existing mathematical tools and tailoring them to get biological insights or was it suggesting new mathematical problems inspired by the challenges and perspectives of biology?” says Nina Fefferman, director of the National Institute for Mathematical and Biological Synthesis. “Both of those practices are now inextricably intertwined in order to get this wonderful feedback where new math is developed because it helps extend what we understand about biology, and that then also suggests how we could go back to think about math itself in new ways.”
To catalyze collaboration and research in this burgeoning interdisciplinary field, the National Science Foundation teamed up with the Simons Foundation in 2017 to create four NSF-Simons Centers for Mathematics of Complex Biological Systems. Established to search for what the NSF terms the “Rules of Life” and headquartered at Harvard University; Northwestern University; the University of California, Irvine; and the Georgia Institute of Technology, the centers allow scientists across disciplines to innovate at the vanguard of quantitative biology.
“There’s a perfect opportunity to invent new mathematical frameworks around fundamental biological questions, if we pose the questions correctly,” says Bridget Queenan, executive director of research at Harvard’s NSF-Simons center.
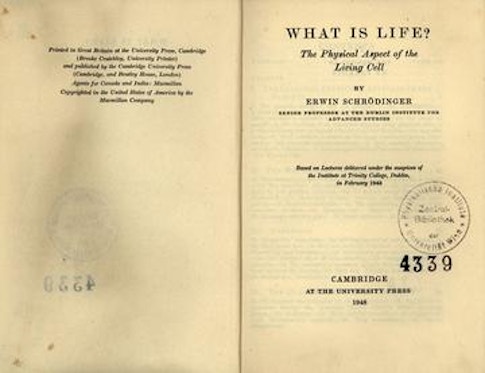
Growing beyond reductionism
Though biologists have been using mathematical tools since around the turn of the 19th century (mathematical diagrams appear in both Gregor Mendel’s groundbreaking paper “Experiments on Plant Hybridization” and in Charles Darwin’s seminal book On the Origin of Species, for example), it has taken decades if not centuries for researchers to develop mathematical tools based on biological facts, rather than ones borrowed from physics and nonliving systems.
Around World War II, molecular biology — which focuses on the biochemical entities that power life — took off. In 1944, the mathematical physicist Erwin Schrödinger wrote a short book called showing how physics and chemistry could help attack problems of biology. Both the biologist James Watson and the physicist Francis Crick have credited What Is Life? with inspiring them as they hunted for the double-helix structure and tantalizing code of DNA (found with X-ray crystallography by Rosalind Franklin). After that find, scientists from a vast array of disciplines started flocking to molecular biology.
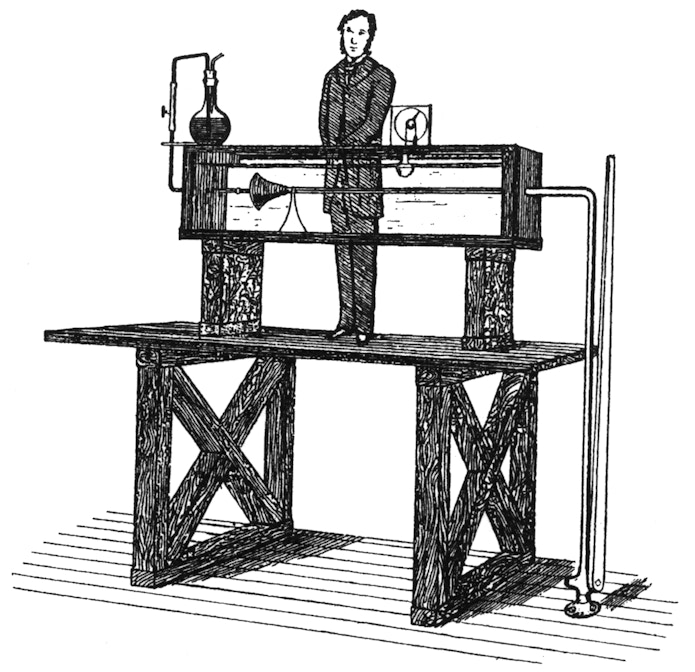
Physicists brought with them the philosophical framework of reductionism, the practice of cutting out all the ‘external’ factors to look at just one part of a problem. In a famous 1883 example highlighting physics’ centuries of reductionism, fluid mechanics pioneer Osborne Reynolds studied the flow of water inside a glass pipe, rather than in ocean waves. As Richard Carthew, director of the NSF-Simons Center for Quantitative Biology at Northwestern, says, reductionism “basically revolutionized the way biology is studied for many of us.”
Today, much biology research still operates firmly within that reductionist paradigm. “Many folks believe that molecules are going to hold the answer to biology,” Queenan says. “People have a lot of faith that we can ‘brute-force’ the important questions — if we measure every molecule at atomic resolution, every synapse, every cellular interaction, if we just get more data, then, somehow, knowledge will appear. Of course, it’s great to have data and it’s great to have the computational capacity to play with it. But no amount of interrogation will force molecules to disclose information they don’t have.”
A deep understanding of biology, then, likely won’t come from cutting things into smaller and smaller pieces and expecting information to manifest the way physics can manifest from reductionism. That’s because physics deals with nonliving things, while biology deals with the complexity of life. In 1960, the physicist Eugene Wigner published “The Unreasonable Effectiveness of Mathematics in the Natural Sciences,” an essay on how “unreasonable” it seems that we can take observations, jot down equations, manipulate them, and pop out actual predictions within the real world. But we use mathematics like this all the time in physics. Wigner also predicted, however, that finding the corresponding equations of life would be “much more difficult and confusing.”
“Physicists have successfully used the reductionist framework by focusing on relatively simple problems and cutting out external factors,” Queenan says. “The behavior of living things is less simple by definition, and if we cut out external factors the things die — at which point we can successfully use equations from physics to predict their behavior.”
“What you don’t realize is what it took for the math and physics community to get to the level where questions about nature can be condensed into a particular integral calculation,” says Madhav Mani, a Northwestern mathematician. He believes that biology needs to look beyond reductionism for its next steps toward finding those equations, though this is complicated by factors unique to the field. “In biology, most of the time, because of its relative theoretical and conceptual infancy, forget integrals, you’re not even sure what the variables should be. I believe that in our study of life and living matter, we have the problem of the wrong variables.”
Pointing to fluid dynamics, algebraic geometry and other famously complicated fields as examples, Mani observes that “when we just found the right variable, they’re simple.”
Meeting the challenges of biology head-on
Applications of math to physics have been abundant for many years; Wigner’s “unreasonable effectiveness of mathematics in the sciences” is a testimony to this. But biology is much more complex: Each individual organism adapts to an array of factors, from the makeup of its genome to the complexities of its ecosystem. In addition to adapting to these factors, living systems also have many interconnected parts and ‘emergent’ properties: traits whose expression is beyond the capacity of individual parts. For instance, in the brain, a neuron’s single axon will only send limited electrical impulses, but combining hundreds of millions of these pulses creates something as complex as a memory.
“Living systems just have all these beautiful and very interesting complexities that are not well handled by physical theories,” notes Rosemary Braun, a computational biologist at the Northwestern center.
Physics doesn’t offer an immediate way to measure differences between individuals in a species. This is where mathematical biology can step in, as seen in a recent study of fruit flies led by a team at Northwestern. Due to the way different species of fruit flies have adapted to different environments, the flies’ wings differ in small but significant ways. At Northwestern, Carthew, a biologist, teamed up with mathematician Mani to figure out a metric for, or rigorous way to measure, the differences. Older metrics identify visual ‘landmarks,’ such as particular intersections of veins, and measure the distance between those landmarks to create a coarse metric: ‘Closer’ wings in landmark metrics have similar distances between their landmarks. But landmarks arbitrarily chosen by humans cannot encapsulate all the data about the wings.
By contrast, the approach taken by the Northwestern team preserves much more information about wing features, such as angles between veins, hairs inside the wing, and edge bristles. Carthew’s lab painstakingly took thousands of images of fruit fly wings; Mani geometrically mapped the images into a uniform format. Using a tool called spectral decomposition, they found a single mathematical ‘mode’ along which all individual wings differ. That means that when fruit flies are raised with different genome sequences, temperatures or diets, they differ along this mathematical mode. Remarkably, this new metric also detects evolutionary differences: The longer ago species diverged, the farther apart the wings are along the mathematical mode.
Though the mathematical tools Mani used have been around for decades (and some for centuries), the real innovation was in posing the problem and figuring out which math to apply.
“The fact is, no one had done this before,” Mani says. “We went through a process of four years of identifying the question, finding how to address the question, finding the piece of mathematics, developing the tools, then finally doing the experiment.”
Mathematical biology is full of examples like the Carthew-Mani collaboration, in which people from both fields can make leaps that neither could make on their own. As Christine Heitsch, director of the Georgia Tech NSF-Simons center, put it, “You don’t necessarily need really powerful math in order to answer some of these biological questions, but you do need a really powerful mathematician: one who can understand these problems and ask the right questions about them.”
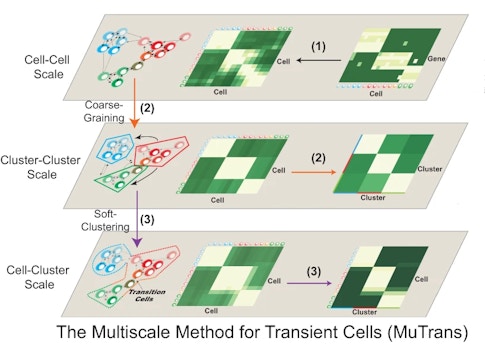
Researchers at the UCI center, for instance, recently applied mathematical manifold theory, which has been around since the mid-1800s, to ‘cell fate,’ or the study of cells and their future identities. Qing Nie, the director of the NSF-Simons center at UCI, the Center for Multiscale Cell Fate Research, led a group that created a dynamical manifold, a 3D surface with hills and valleys, to model cell fates. Every point in the manifold represents an individual cell, with valleys representing cell types. The geometric information of the manifold near a point shows the probabilities that a stem cell will transition into a particular cell type. Most biologists will never take a course in manifold theory, and most mathematicians won’t concern themselves with cells. The UCI center was key to the development of this new tool in understanding cell fate.
Also at the UCI center, bioengineer Tim Downing and applied mathematician Elizabeth Read have been using stochastic modeling, which takes randomness into account, to examine the epigenetics of a cell. While the genetics of a cell refers to its DNA, the epigenetics of a cell has to do with what’s on top of that DNA: the shape of the chromatin, or the helix, itself, and the molecules that surround it. If the DNA is a library of information telling the cell how to do its job, the epigenome is a librarian explaining which books, or genes, the cell needs and which it can ignore.
While we mostly understand how cells duplicate DNA when making more cells, the epigenome is still a mystery. Downing takes measurements over the course of a cell cycle (the time it takes for a cell to divide into two daughter cells), and Read analyzes them while simulating the underlying biological mechanisms. Together, they’ve found that enzymes travel along DNA and build the epigenome sequentially, rather than more diffusely, all at once. Their findings hint at the shape of the chromatin: If methylation (a biological process that changes DNA activity) happens in two disparate regions of DNA at the same time, this suggests that those locations are adjacent in the twisted DNA.
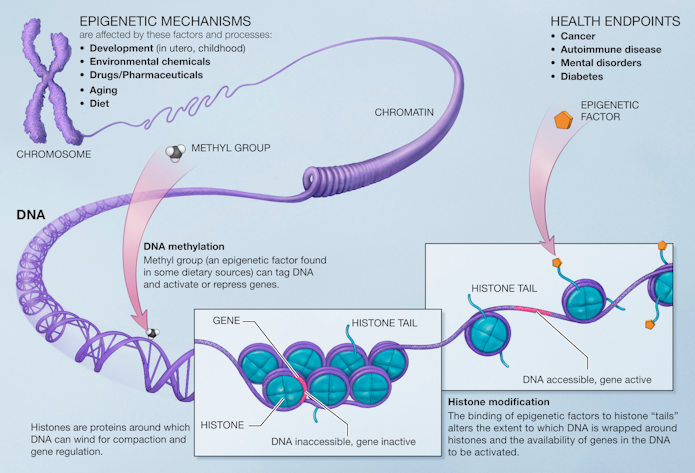
When mathematics and biology meet, serendipitous theories can pop up in both fields with enough prior knowledge. For instance, while working on ways to code RNA structures, she discovered something equivalent to a well-studied combinatorial object. The object was much easier to analyze in the context of RNA, and she ended up solving an open problem in combinatorics — a problem she never would have considered without the biological motivation.
Later, a biology student started working with Heitsch’s object and tried using a graph, or collection of dots with edges between them, to describe the RNA folding. The student thought they could list all possible paths as a first step, but Heitsch knew that the enumeration would be a computational nightmare because of existing mathematics in graph theory.
“Graph theory is not a type of mathematics they’re normally exposed to,” Heitsch says, noting that many biology majors only take calculus. “There are all sorts of mathematics being used these days to analyze biology. If you understand the biology well enough, you can see how math like this can be a very powerful tool. We’ve been successful in expanding the biologists’ horizons with what is possible with mathematics.”
Modernizing the marriage of biology and mathematics
The ultimate goal of the NSF-Simons centers is to foster a virtuous cycle where observations from ‘wet labs’ of experimental biologists lead to new mathematics from ‘dry labs,’ which then spurs new experimental setups for more ‘wet’ experiments.
For instance, Braun develops mathematical tools to search for changes at the molecular level of thousands of genes that are affected by circadian rhythm, continuing a line of research that won several researchers the Nobel Prize in physiology or medicine in 2017 by identifying the so-called clock genes in fruit flies. The lab she collaborates with measures every gene of the fruit fly every two hours for 27-36 hours under varying conditions; Braun’s algorithm then finds patterns within the noisy data, looking for genes that exhibit periodicity, or a connection to circadian rhythm. With the wet and dry data together, the labs figure out how circadian clocks help fruit flies adapt to environmental changes. Braun has immense respect for the wet lab and “how much knowledge and insight and intuition goes into designing and carrying out those experiments and how much patience you have to have.” She bemoans “transactional” collaborations and revels in her relationship with her biologist collaborators.
“Some people just want the statistics done so that they can put it in the grant or paper and you never discuss the science again — that’s a little bit unfulfilling,” Braun says. “At the end of the day, I like the back-and-forth where we can do some analysis, come back with suggestions for different experiments, and have a real dialogue between experiment and theory. The experiments that the lab is carrying out now are experiments that we were deeply involved in designing, so that we would be able to apply these types of methods to the data that comes out.”
Measurements for those experiments and data have advanced by leaps and bounds since Wigner’s original 1960 essay. When mapping genes for the Human Genome Project in the 1990s, scientists around the world would label one or two genes or proteins with a particular fluorescent marker and then take an image to find the locations within the genome. Nowadays, scientists can mark all 20,000 or so genes at once with spatial single-cell genomics. This impressive feat presents a massive data problem for mathematical biologists. Happily, mathematics has tools for identifying and isolating relevant information, bridging the gap between massive data tables and figuring out what genes are actually doing.
With new assays, CRISPR technology and more “big data,” Fefferman explains, mathematical biologists benefit from incorporating more machine learning and computer engineering tools into their work.
“In a very real sense, the mathematical techniques developed for the computational capabilities to handle big data are an incredibly beautiful challenge,” Fefferman says. “It’s like saying, let’s put a jigsaw puzzle together, but you only get to have 20 random pieces of the puzzle at once, and every time you place one, you get to have another piece. And the puzzle is 50,000 pieces.”
The NSF-Simons center researchers hope that the rigor and abstraction of mathematical thinking will not only help solve biological puzzles but also accelerate biological discovery. For instance, as Queenan puts it, an Earth-centered universe with concentric circles of planetary orbits was “a reasonable first guess” by Ptolemy and others. And observations at the time resulted in the concept of epicycles, which were used to accurately predict where a planet was at any given time but gave no insight into how they worked and couldn’t be extended to new objects. “It wasn’t a terrible model,” says Queenan. “It just wasn’t the best. To ensure that biology doesn’t settle for a similarly phenomenological understanding of life for a millennium, we need to combine the best parts of biology with the best parts of mathematics.”
To avoid being led astray by purely descriptive theories like epicycles which do not help explain the world, researchers hope to bring biology and mathematics closer together so they can understand how logical conclusions follow from axioms of the biological world.
“One of my big concerns is that we can take these incredibly rich datasets, run them through very sophisticated analysis pipelines, and get predictive results — but are we just getting really great epicycles?” Braun says. “Right now, it’s such an exciting time, because it asks of us as a community to think about that, and to think about what first principles of biology should look like.”