A New Era of Perception Research
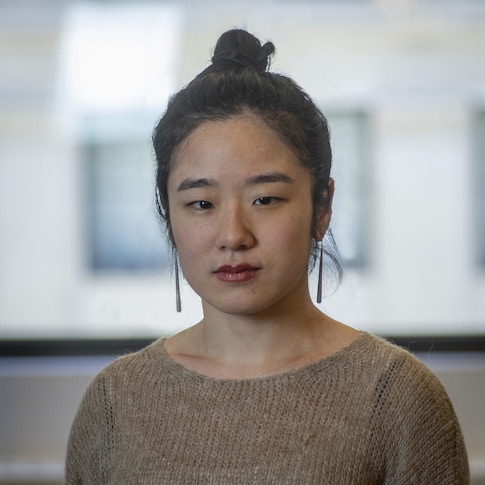
Scientists and philosophers have long been fascinated with how we perceive the world around us. In the era of high-powered computing, researchers are taking new approaches to old questions about perception. Flatiron Institute scientist Jingyang Zhou uses mathematics and computational models to understand how we perceive visual changes like contrast or brightness. Her research is laying the groundwork for illuminating the connection between fundamental neuroscience and human behavior.
Zhou has been a research fellow at the institute’s Center for Computational Neuroscience since 2020. Prior to that, she studied and worked at New York University, where she earned an undergraduate degree in mathematics and economics and a Ph.D. in psychology, and was a postdoctoral research scientist at the Howard Hughes Medical Institute.
Zhou spoke with the Simons Foundation about her work and about where the study of perception is headed. The conversation has been edited for clarity.
How do you study perception?
Perception is essentially our ability to identify and interpret our surroundings — a critical mechanism for navigating our world. To study perception, many researchers focus on the way in which sensory information, say, from our eyes, is represented by neurons in our brain. Other researchers, myself included, want to understand how those neural computations are then transformed into an understanding of what we see.
More specifically, the focus of my work is to build a theoretical mathematical framework for perception — how we can quantify the appearance of the physical properties around us, such as the color contrast of an image. Right now, I use pre-existing data on how our brains interpret change in images, though I’m starting to collaborate with experimental scientists at New York University and the University of Giessen in Germany to generate new data.
In one type of perceptual experiment, a subject is shown two versions of an image on a computer that are similar, but with slightly different hues. The subject is asked to distinguish between the two versions. From this experiment, we can measure how the subject’s perceived image change relates to the actual difference. In another perceptual experiment, subjects may be asked to rate how bright an image is on a scale of 1 to 10. From this, we can directly measure what the subject perceives in terms of the physical property of the image.
My research so far has been used to build a model describing this relationship between perception and physical change. However, it can get complicated because we aren’t actually capable of perceiving all changes. For example, we can make an image really bright, but at a certain point the changes in brightness become indistinguishable to us.
Traditionally, when people studied perception, they sought to quantify experimental data. In this approach, the more experiments you do, the more reliable your data become and the better your model can be. However, there are different ways to conduct perception experiments, and each might be measuring different aspects of perception. This can lead to the challenge of comparing apples to oranges.
By taking a computational approach, I’m hoping to build a model of perception that can connect different types of datasets more accurately. This work will help with quantifying data, identifying what we’re really measuring across different experiments, while also revealing how the different variables we’re measuring are connected.
How is technology changing the study of perception?
The advancements in computational and statistical techniques these days are giving us new perspectives on old questions. It used to be that we could only study one variable at a time. For example, we could show someone a red pixel, followed by a brighter red pixel, and ask them to tell us how they perceived the difference.
Now with theoretical and computational advances, we can measure thousands of variables all at the same time. This means we can show someone a whole still-life painting of a fruit basket and instead of just changing contrast or brightness, we can make apples be more orange-like. In this case, we can ask many more questions, such as what changes do they see in general. We can then input this extra information into artificial intelligence algorithms to better understand how we perceive an image. This increase in dimensionality and data is dramatically changing our theories and will introduce a lot more questions that we’ll be able to ask about perception.
Are we entering a new era of perception studies?
In some ways I think so. We’re in this interesting period of the field where we’re starting to do some things differently now that we have advances in technology and computer sciences. We’re building on the groundwork laid by earlier scientists who had a lot of theoretical insight but didn’t have the available technology to carry out certain experiments. With higher computing power, we’re starting to make big leaps in our understanding of perception.
There’s also beginning to be a move to specialization. In the past, a lot of perception studies have been done by single labs with scientists doing modeling, behavior and brain experiments all at once. Many of the researchers are trained in both the experimental and theoretical sides. But we’re getting to a point where we need to have a division of labor, where people are more specialized in one discipline or the other, in order to make further big steps in understanding perception. In the future, I see the field shifting further: toward more collaborations between specialists. I think this will really help launch a new era of perception studies.