Looking for Patterns: Understanding How We See the World
At the Flatiron Institute, Tim Oleskiw combines experimental and computational methods to figure out how our visual systems work. He hopes his work can help spur advances in everything from better visual aids to improved search-and-rescue methods to more advanced self-driving car systems.
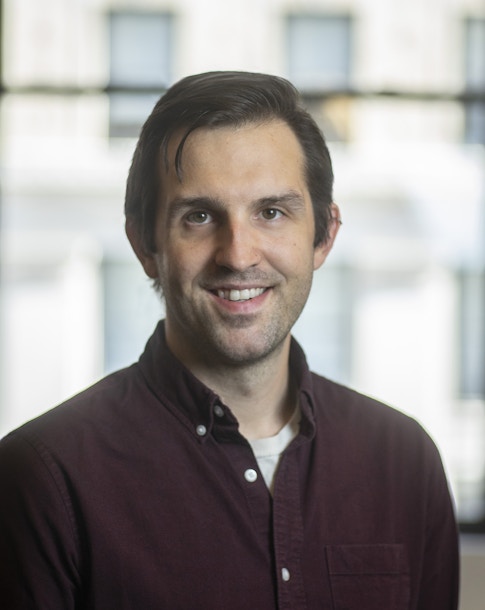
In the future, computational models that replicate human vision could help look for missing people in dangerous search-and-rescue missions. The same models could be used to design visual aids for people who are blind or who have low vision. But before that can happen, scientists need to figure out how signals from our eyes are processed and transformed in our brains.
Tim Oleskiw, a Flatiron Institute Research Fellow, is working to do just that. With experimental data and computational models, Oleskiw is trying to understand how the brain perceives fundamental signals of vision. He hopes this work can eventually be used to design visual aids and computational vision systems.
Oleskiw joined the Flatiron Institute’s Center for Computational Neuroscience as a research fellow in 2020. His experimental work is performed in the Visual Neuroscience Laboratory at New York University’s Center for Neural Science. Oleskiw received a doctorate in applied mathematics from the University of Washington and a master’s in computer science from York University.
Oleskiw recently spoke with the Simons Foundation about his work and about how we perceive the world around us. The conversation has been edited for clarity.
What projects are you currently working on?
I use experimental and computational methods to map parts of our visual system. I’m particularly interested in understanding the intermediate processes in vision: the point where our brain starts to make sense of surfaces, boundaries and shapes, allowing us to perceive individual objects in natural environments.
After light enters our eye and hits the retina, signals are sent to the primary visual cortex, V1, at the back of the brain. V1 responds to edges, such as object boundaries. For example, if I showed you a piece of paper that was half black and half white, neurons in V1 would respond to and identify the edge or boundary between them. I study how information that leaves V1 is processed in a subsequent region of the visual cortex called V2.
V2 deals with grouping and processing the edges or patterns of contrast detected in V1 into more meaningful signals. Features like the edge of an apple or the curve of a leaf are encoded in area V2, as well as another cortical region called V4. This information is then sent to other parts of the brain, like the inferior temporal cortex, tasked with recognizing the image. Finally, higher brain regions are then responsible for other processes, such as moving your arm to grab the object you recognize.
The first experiments on human vision date to the 1950s and were largely relegated to studies of V1. Now, we’re doing more of that same work — but in more complicated brain areas with more complicated computational tools.
How do you study these brain regions?
We use both experimental and computational techniques to gather and analyze data on how a real brain works. At our NYU facility, we train primates to watch a computer monitor, and we track their neural activity as they see different images: bars of color that show up in different locations on the screen in various orientations and sizes. With this, we’re trying to activate different neural regions to see which ones are responsible for different parts of vision. By recording the neural activity and modeling it, we can map the neurons and learn how they’re connected using machine learning and artificial intelligence.
Over the years, we’ve collected large datasets that we used to train a model that describes what neurons are involved with perceiving different visual cues. We are testing the model by using some extra data (not used for training our model) and showing it the same images that we showed the animals.
Why is it important to understand these visual processes?
In a nutshell: We still don’t really know how vision works. There have been many attempts through machine learning and artificial intelligence to solve certain visual tasks like facial recognition. Sometimes these tasks work, but they have noticeable limitations compared to our own visual system, and there’s much to be learned from studying how biological vision systems work. The approaches that we use are like reverse engineering, where we’re learning how the brain processes vision to be able to apply that to computer vison systems. Essentially, we’re breaking down vision into its individual components, studying each region of the visual system in order to make inferences about the system as a whole.
The end goal is to build computational models that replicate human-like vision. If we could build a machine that could perceive the world like humans do, then we could restore sight to someone who’s blind or improve the quality of life of people with low vision, for whom current technologies are insufficient.
It could also be used to automate vision tasks for search and rescue or operating in dangerous environments. It’s not uncommon for search-and-rescue personnel to use footage from an automated drone deployed over densely wooded terrain to search for a missing person. This requires a huge amount of work hours, and it’s currently too complicated a task for computational vision systems. If we can figure out how the human brain parses cluttered visual scenes into salient surfaces and objects — and distinguishes between trees, ground and people — we can automate the process to free up highly trained people for other tasks.
How far off are these applications?
My hope is in a few decades, well, we’ll have a pretty good idea for what each of these regions does, how they function, and that we’ll be able to model them. I think that the person who will benefit from the vision prosthetics we are developing has already been born. The technologies that could come out of understanding vision really have the potential to be life-changing.