Between Knowing Nothing and Knowing for Sure: The Science of Uncertainty
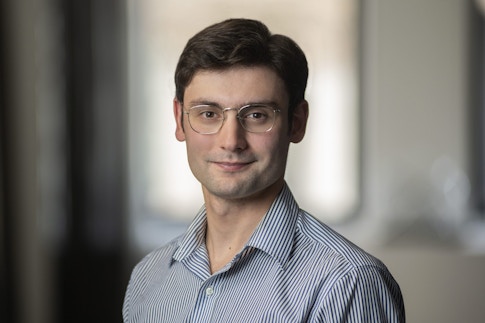
As a self-proclaimed statistics democratizer, Charles Margossian works to demystify the complexity of powerful statistical methods for scientists in all disciplines — from astronomy to epidemiology. He focuses on Bayesian modeling, which has grown in popularity with computational advances in recent years, and its implementation in free, open-source statistical software, such as Stan. Bayesian modeling can be an incredibly powerful tool to learn from imperfect observations — but only if it’s implemented properly.
Margossian is a Research Fellow at the Flatiron Institute’s Center for Computational Mathematics (CCM). Prior to joining CCM, he earned a doctorate in statistics from Columbia University in 2022, and a bachelor’s degree in physics from Yale University in 2015.
Margossian recently spoke to the Simons Foundation about his work and dreams for a more democratic future.
What is Bayesian modeling?
Bayesian modeling is a type of statistics that uses the language of probability to describe unknowns. It’s helpful in cases where you have imperfect data. This could mean a relatively small or incomplete set of measurements or abundant but noisy observations with which we try to understand an incredibly complicated phenomenon. Imagine you were trying to model the skeleton of a newly discovered dinosaur species, but you only had half of its fossilized bones. The data tell you something about the dinosaur, but not enough to differentiate between wildly different models of the skeleton. Bayesian methods help us quantify that uncertainty and allow us to understand how much we can learn from the data.
Bayesian modeling is useful in all types of situations — from sports betting to pharmacology to cosmology. In all these fields, quantifying uncertainty is hugely important. Take Google Translate, for example. Google Translate is essentially a model that, given an input sentence, will return the sentence in a different language. The model is programmed to always provide an answer, but because there can be a lot of nuances in language, it’s not always right. Getting the wrong answer is OK if you’re just trying to order dinner in a foreign country, but if you’re working on a critical problem, it’s not enough. For example, if you’re hoping to decide what COVID-19 lockdown policies should be implemented in your state, or the efficacy of a new drug treatment, you need to know how accurate that answer from the model is.

When the COVID-19 pandemic started, I was a doctoral candidate at Columbia University. I joined an international collaboration of scientists, led by epidemiologists from Switzerland, who wanted to figure out the disease’s mortality rate. This was challenging because, at the time, testing was not widely available, and people with severe symptoms were given priority. As a result, we had an incomplete and biased dataset. By using Bayesian modeling and a statistical software tool called Stan, we were able to develop a model and compute a distribution of possible mortality rates. This gave us best-case and worst-case scenarios, which is what policymakers need in order to make decisions in an incredibly uncertain situation. Moreover, we had to find a balance between not throwing away the data on the grounds that they were flawed, and simultaneously acknowledging these flaws and adjusting our conclusions accordingly. We published our findings, and a technical discussion on how Stan helped us achieve our goals, in two peer-reviewed journals.
What aspect of Bayesian modeling do you work on?
My work is primarily methodological. I work at making Bayesian modeling more accessible to scientists and making sure they are using it correctly.
Bayesian modeling is very challenging mathematically and computationally. Only in the past few decades have we had the computational capabilities to even attempt some applications that previously were too complex to be computed. As Bayesian methods become more feasible, more people want to use them. However, this can be tricky because many Bayesian algorithms have a lot of parameters that need to be tuned. Unless you’re an expert in statistics and algorithms, it can be very difficult to fine-tune your method for it to work well — or even at all — for a specific application. A lot of my research revolves around creating self-tuning algorithms that can adapt to different problems without needing extensive input from the users. This is part of a broader push in the field to build user-friendly algorithms that a scientist can apply to their problem and data.
I’m also working on frameworks to help scientists pick the right kind of Bayesian algorithm for their project. In the past, people thought there could be an algorithm so general that it could be seamlessly applied to almost every problem. Successful, general-purpose algorithms do exist, but sometimes we need strategies that are specifically adapted to the problem that we’re interested in, especially when working with complex models or large-scale data. In practice, there are lots of different candidate methods to choose from, raising the question: Which is best for a certain project? In my work, I engage with two approaches to answer this question. The first one is to establish the strengths and weaknesses of an algorithm by studying it theoretically and trying it out on many different problems. This gives us rules and, more often, heuristics for when to apply a technique. But sometimes, we cannot know if a method works until we try it. The question becomes: Can we tell that a method fails after trying it, and can we understand why it fails? This is where the second approach comes into play: diagnostics. To make this approach accessible to scientists, I am implementing such diagnostics in open-source statistical software, such as Stan, with my colleagues at CCM and at other institutions. This is a potent way to make the technology available, given that Stan already has thousands of users across a wide range of fields — epidemiology, pharmacology, political science, economics, astronomy, physics, ecology and more.
Through these projects I’m trying to walk a line between ease of use and oversimplicity. I want to alleviate the burden for the scientist so that they can use a high-performance algorithm without having to expend too much effort, but I also don’t want them to be mindlessly pushing a button and automatically trusting the result. This can lead to the recurrent issue in statistics where people fail to appreciate the nuances of a statistical method and come to the wrong conclusions.
One way we can circumvent this issue is by creating software implementations that give a lot of informative warning messages and that automatically run diagnostics after the application of an algorithm. In that respect, Stan is one of the more verbose statistical software tools out there. Another way of addressing this issue is by teaching people. I go to a lot of conferences and speak to scientists so they can understand the statistical methods they need for their work. If we want to democratize statistical algorithms, then we need to teach people how to use them in an informed and responsible way.
At the Flatiron Institute, I get to interact daily with scientists, and I can help them with their data. They might come to me with an issue, and we’ll figure out which algorithm would be best and what diagnostics should be used to know if it’s working.
What kinds of projects have you helped with?
In addition to the COVID-19 project, I’ve worked on problems in pharmacology, genomics and astronomy. Currently I’m working with some of the astrophysicists here at the institute’s Center for Computational Astrophysics who look at gravitational waves, which are ripples in the fabric of spacetime. They want to identify where the gravitational wave signals originated, which could be two black holes colliding somewhere in the universe. But the data are very noisy and imprecise, so you have to follow up with observations from another telescope. To do this, you have to know generally where to point the telescope.
Using Bayesian analysis, we can map all the plausible places where the event could have occurred and figure out where to point the telescope to look at it. We’re working to fine-tune the Bayesian algorithm so that it can work quickly and accurately. Ultimately, I hope other astronomers will see this work and be able to apply it to their own projects.