Scott Linderman, Ph.D.
Columbia University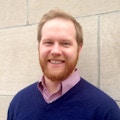
Scott Linderman is an Assistant Professor in the Statistics Department and the Wu Tsai Neurosciences Institute at Stanford University. He works at the intersection of machine learning and neuroscience, developing new models and algorithms to better understand complex biological data. Scott was a graduate student at Harvard University with Ryan Adams and Leslie Valiant and then a Simons Collaboration on the Global Brain Postdoctoral Fellow at Columbia University with Liam Paninski and David Blei. His methodological work has focused on a variety of neural data analysis challenges. He has built probabilistic models to discover latent network structure in neural spike train recordings, and state space models to find low-dimensional states underlying neural and behavioral time series. He develops inference algorithms necessary to fit probabilistic models at scale, and works closely with experimental collaborators to apply these methods across a variety of model organisms. His lab at Stanford aims to develop the computational and statistical techniques necessary to extract scientific insight from next-generation neuroscience datasets.
Project
“Discovering Latent States of Neural Activity and Behavior”
For much of the history of neuroscience, neural activity was observed one neuron at a time or a handful of neurons at a time. Now, thousands of neurons can be observed simultaneously, and their activity can be correlated with precise measurements of an animal’s repertoire of behaviors. These developments offer an unprecedented opportunity to link the complex dynamics of neural activity to natural behavior, but the massive data sets generated by new techniques pose extraordinary statistical and computational challenges. It is unclear, for instance, how to formulate a joint model of neural activity and behavior when their relationship could, in principle, be infinitely complex. Fortunately, preliminary observations of large-scale neural recordings reveal that groups of neurons reliably participate together during behaviors, greatly reducing the realm of possibilities. But how this reduction occurs is an open question. One obstacle is a lack of sophisticated statistical and computational tools. I have developed models known as “switching linear dynamical systems,” which can identify both discrete and continuous latent states of neural activity and behavior. For example, these models can learn to segment movies of mouse behavior into interpretable “syllables,” like turning right, turning left, or rearing up on its hind legs. Moreover, these models can discover the continuous dynamics of neural activity that underlie these behavioral syllables. Because these models are computationally intensive to fit—prohibitively so using traditional methods—I have developed novel algorithms to scale these models to modern data sets. Models such as these will allow for the design of new experiments in which neural activity is manipulated in real time, leading to causal explanations of brain and behavior.