
Inner Workings of the Brain Explored at 2017 SCGB Annual Meeting
The Simons Collaboration on the Global Brain (SCGB), a grant program launched in 2014, seeks to discover how neural activity gives rise to the most mysterious aspects of cognition by pairing new technologies with powerful computational and modeling techniques.
This past September, SCGB investigators and fellows convened in New York City to share their findings at a two-day conference. The meeting marked the launch of SCGB’s newest round of grants, 20 collaborative projects that continue SCGB’s commitment to bringing together experimentalists and theorists. Researchers shared advances from previous projects as well as plans for their new endeavors. We describe here a handful of the more than 30 presentations at the meeting, which explored topics ranging from new imaging technologies to novel modeling and analysis techniques.
How circuits generate flexible behavior
The smell of freshly baked bread can be intoxicating if you’re hungry but repulsive if it recently made you ill. How is the nervous system engineered to flexibly respond to the same odor depending on the situation? Vanessa Ruta of Rockefeller University is exploring this question by studying olfactory processing in fruit flies.
Ruta’s work focuses on a structure in the fly brain known as the mushroom body, which is essential for olfactory learning: it enables a fly to become attracted to an odor when it predicts something good or avoid the same odor if it predicts something unpleasant. “The mushroom body is a critical node in the olfactory circuit that can translate odor signals into different types of behavioral responses,” she says.
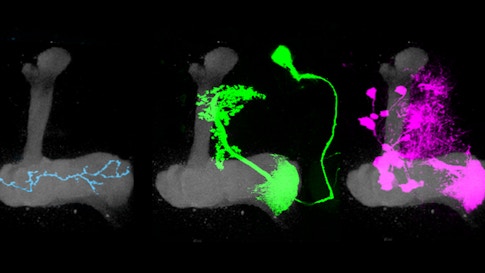
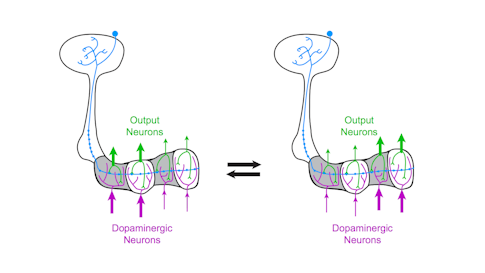
The mushroom body’s circuitry comprises three main cell types. Kenyon cells receive and integrate olfactory information using a unique pattern of activity to encode the identity of an odor. Kenyon cells relay this information to mushroom body output neurons (MBONs), a collection of 34 cells organized into compartments. Kenyon cells target MBONS in many compartments. Each compartment also gets input from a unique population of dopaminergic neurons.
Previous research has shown that dopamine neurons in the mushroom body are essential for olfactory learning, such as when a fly learns to associate a specific odor with a sugar reward. Some dopamine neurons respond to positive cues, such as sugar, while others are tuned to more aversive cues, such as a mild shock. When dopamine is released into a specific compartment it changes the synaptic connections between the Kenyon cells and MBONs (see diagram), triggering learning. Simply activating different dopamine neurons in the presence of an odor is sufficient to teach flies to like or avoid the same odor.
“The architecture of this system is beautifully set up — each compartment receives the same olfactory signals but gets unique instructive input from dopamine neurons,” Ruta says. “In this way, the mushroom body acts like a switchboard where the same odor signal can be rerouted to different output pathways depending on input from the dopamine neurons.” In other words, dopamine-induced learning controls this switchboard, redirecting olfactory signals through the mushroom body. The system allows animals to modify their responses to odors depending on their past experience. They can also respond differently to odors depending on their current situation, such as whether or not they are hungry.
Ruta’s work suggests that dopamine neurons play an additional role as well. They not only respond to positive or negative stimuli but also provide an ongoing record of what the animal is doing. Ruta’s team images dopamine neurons while flies actively track an odor, allowing the researchers to correlate neuron activity and behavior. They found that some dopamine neurons are active when the animal initiates motion, others when the animal is speeding up or turning into the odor plume. “We can simply look at activity of dopamine neurons and predict what the animal is doing,” Ruta says. “They represent distinct facets of locomotion with remarkable fidelity.”
It’s not yet clear why dopamine neurons would provide an ongoing signal of what the animal is doing. Ruta theorizes that the animal’s actions could serve as a reinforcement that instructs learning. “It’s possible they are representing not just the sensory signals the animal receives, but the behavioral response to that signal,” she says. Moreover, these dopaminergic neurons may be involved in shaping and reinforcing behavior, suggesting an additional role for the mushroom body beyond olfactory learning.
Ruta notes that findings from fly studies will likely apply to mammals, which have similar dopamine systems. Indeed, Ilana Witten, an SCGB investigator at Princeton University who also spoke at the meeting, found that some dopamine neurons in the mammalian brain instruct learning while other seem to be involved in motor control and reinforcement of ongoing actions. “Remarkable parallels exist between invertebrate circuit motifs and their mammalian counterparts,” Ruta says.
The complexity of mammalian brains makes it difficult to study exactly how the interaction between motor control and reinforcement learning works. But in the fly, scientists can study how the same neuron carries different signals under different conditions. “We can say with certainty that the same neurons that drive learning also carry information about the ongoing behavior of animal, so we can explore why these signals might be combined at the level of these dopaminergic neurons,” Ruta says.
Ruta will further explore these questions as part of her new SCGB project, “The Representation of Internal State of The Fly Brain,” in collaboration with Larry Abbott, Richard Axel, Elizabeth Hillman, Yoshinori Aso and Gerald Rubin. Researchers will look at how internal state, such as hunger or satiety, influences sensory processing. “Where in the fly brain are changes occurring that alter this response?” asks Ruta. “Is it localized to a small area like the mushroom body or occurring at every level of the sensory processing hierarchy?”
Why don’t muscles move when we think about moving them?
When we think about reaching for a coffee cup, the motor cortex shows a pattern of neural activity similar to when we actually make the move. Why do similar activity patterns sometimes translate into movement and sometimes not?
Previous research suggests that neural activity patterns linked to movement are qualitatively different from those that aren’t. In mathematical terms, these patterns reside in different dimensions. In so-called ‘motor-potent’ dimensions, the linear combination of neuronal activity triggers a net output on muscle. In ‘motor-null’ dimensions, the summed activity essentially cancels itself out, having no effect on muscle.

Motor-null dimensions may offer the motor cortex the ability to plan a movement before executing it. In new research, Krishna Shenoy, a neuroscientist at Stanford, and collaborators suggest that motor-null dimensions also help the motor cortex process new sensory information during movement planning. “We think of those null dimensions as a scratch pad where computations can happen,” Shenoy says.
If someone bumps your arm as you as you reach for a coffee cup, the brain processes that sensory information and adjusts the arm’s reach accordingly. But exactly how that happens is puzzling. Neural recordings have shown that sensory information itself can travel to the motor cortex very quickly, so how does the brain have enough time to act on it? Shenoy predicts that new sensory information briefly lingers in motor-null dimensions and then shifts to motor-potent dimensions, where it directs movement.
The researchers tested this hypothesis in monkeys implanted with a brain-machine interface (BMI), a device that records neural activity from roughly 200 neurons in the motor cortex and translates it into some kind of output. In this experiment, the monkeys learned to move a cursor on a computer screening using just their thoughts. On some trials, scientists made the cursor jump, mimicking someone getting bumped while reaching for coffee. The monkey then had to alter its thinking to correct the cursor’s movement.
Because the researchers are using a BMI, they know precisely how neural activity maps to the cursor’s movement and can clearly define motor-null and motor-potent dimensions. They first record neural activity and the location of a moving target on a screen, which the monkey is trained to plan to reach to. This generates natural motor cortical activity. They then fit a decode model to the neural and kinematic data, which defines a weight matrix that transforms the former into the later. With the weight matrix in hand, they can then use standard linear algebra to define which dimensions are null and which are potent.
Shenoy’s team found that neural activity linked to the visual ‘bump’ did indeed exist in the motor-null dimension initially. After about 50 milliseconds, it morphed into the motor-potent dimension. “The data fall beautifully into those dimensions,” Shenoy says. The results suggest that the motor system performs computations locally before using that data to direct movement.
The researchers next plan to do that same experiment with monkeys trained to use a haptic robotic arm, which can detect tactile feedback. “Now that we can physically perturb the arm, we want to know if proprioceptive information also comes in to the null space first and to see how general this computational principle really is,” Shenoy says.
The researchers also want to apply the findings to their clinical work with people learning to use robotic arms. The software that translates brain activity into arm movement needs to know how to react if the robotic arm gets jostled. For example, the software needs to be able to distinguish motor cortex activity that is being processed in null dimensions from activity that is intended to drive arm movement. “We think that by understanding the null and potent dimensions, we can have people controlling robotic arms without the arm flailing around if it bumps into something,” Shenoy says.
How do visual inputs guide navigation in fruit flies?
To process visual motion, the brain has to compare a visual signal at different moments in space and time and decide whether the object is moving left or right. Neuroscientists have traditionally thought that different systems — primarily the vertebrate cortex and retina and the fly brain — employ different strategies for processing visual motion. Previous research suggests the vertebrate retina compares relevant neural signals using something like division, the cortex using a combination of addition and squaring, and the fly brain using multiplication. But Thomas Clandinin, a neuroscientist at Stanford University, and collaborators found evidence that challenges this view of the fly. Their research suggests that the fly brain uses addition combined with squaring, indicating that its computations closely resemble those seen in vertebrate cortex.
“That’s interesting in a broader context because if you think about motion processing as a paradigm for how the brain computes, you would think there’d be lots of different ways to solve this problem,” Clandinin says. “The result that flies use the same algorithm found in vertebrate cortex suggests that the computational space in the brain for this problem is actually quite small. Different animals converged on the same algorithm, even though they have very different wiring diagrams.”
Clandinin is now studying how the fly uses this visual information once it leaves the visual system. “We know a lot about visual processing and circuitry in parts of the brain that are strictly visual, but it’s unclear how visual processing pathways are organized downstream of these initial steps,” he says.
To address this question, Clandinin’s team has developed tools for imaging neural activity across the entire fly brain. The researchers use calcium imaging to capture brain-wide neuronal signaling and then register that activity on a standardized map of the fly brain. They can then compare brain activity from fly to fly.
The software, which is publicly available, “allows anyone to take calcium imaging from any part of the fly brain and register it to a central framework,” Clandinin says. Because the fly brain is so well-described, researchers can then identify candidate cell types within brain areas that respond to visual signals. “You can go from a survey level mode of looking at functional populations of cells to identifying specific cell types,” he says.
The researchers also developed a new way to map the structure of fly behavior. They first converted more than 1,000 hours of fly trajectories — such as flies walking and turning — into a series of velocities and accelerations, known as a kinematic field. “With enough data, you can describe every possible thing individual flies might do as they walk,” Clandinin says. Researchers searched for patterns in the data using independent components analysis and clustering methods, segmenting walking behavior into 15 subcomponents. (The components are different from how a human observer might define behavior — for example, very similar looking turns fall into three different categories.)
The ability to easily quantify behavior will allow scientists to decipher the rules for how visual input shapes an animals’ navigation, a central question in Clandinin’s new SCGB project, “Dissecting navigation and the general logic of episodic state computation.” Different members of the collaboration study different systems, so researchers will eventually be able to evaluate whether flies and mice use the same computational strategies.
Fast, three-dimensional imaging in behaving animals
Elizabeth Hillman, a biomedical engineer at Columbia University, described a microscopy technique she developed, called SCAPE (swept confocally aligned planar excitation) microscopy. With SCAPE, researchers can record cellular-level activity in freely moving animals in three dimensions. Hillman says her technique is capable of 3D imaging speeds 10 to 100 times faster than most laser scanning confocal, two-photon and light-sheet microscopy systems.
Traditional light sheet microscopy employs a pair of objective lenses positioned around a carefully mounted sample. The process of forming a 3D image is fairly slow because the focal plane of one lens has to be moved to stay aligned with the moving position of the light sheet formed by the other lens. SCAPE uses a single objective lens, and neither the sample nor the lens needs to move during scanning. That means the target itself doesn’t need to be held still, making it possible to capture freely moving animals and even the intact mouse brain.
SCAPE’s single lens emits a diagonal sheet of light, while fluorescent light generated by the sheet is detected back through the same lens. A mirror within the system causes the sheet of light to move through the sample sideways, while the same mirror causes the plane of focus of an obliquely aligned camera to move simultaneously. “It’s a neat way of having the camera always focused on the plane as the plane sweeps through the sample,” Hillman says. “As we move the sheet from side to side, we make a 3D image without moving the sample.”
Stacking the images together creates a three-dimensional reconstruction. “You can image at 10 to 20 volumes per second with no other moving parts,” Hillman says. “We’ve spent the last two years pointing it at every sample we can think of, finding new experiments it allows us to do.”
By clicking to watch this video, you agree to our privacy policy.
So far, researchers have imaged fruit fly larvae as they crawl, as well as evoked and spontaneous activity from the entire adult Drosophila brain.
At the SCGB annual meeting, Hillman presented preliminary results of whole-brain imaging in tethered adult flies whose neurons were engineered to express calcium-sensitive fluorescent proteins. “The beauty is that fly brains are just about the right size to fit in the field of view of a microscope,” Hillman says. “Our technology can give an image of the whole brain at cellular resolution and at a speed fast enough to watch the neurons flashing.”
The researchers have paired the SCAPE system with a setup that allows flies to walk on a rotating ball as they navigate a virtual reality maze or respond to different odors. “My dream is to literally capture every single neuron in brain while the animal is continuously behaving, when it walks, smells odors or eats,” Hillman says. “This is a way we can really get down to how the brain works as a whole. Which parts contribute to different behaviors? How do they change over time? What is the role of spontaneous firing?”
To confirm they are able to image flies at a single-neuron resolution, researchers used SCAPE on flies with genetically labeled subsets of neurons that have already been well characterized. Hillman is working with Liam Paninski, another SCGB investigator at Columbia, to analyze the enormous datasets that SCAPE produces, as well as with many other investigators to optimize SCAPE for a range of different applications in biology and neuroscience, from imaging the beating heart to the awake mouse brain. In her SCGB project, Hillman is working with Larry Abbott, Yoshinori Aso, Richard Axel, Gerald Rubin and Vanessa Ruta to apply SCAPE to understanding the internal state of the fly brain.
The technology was recently licensed to Leica Microsystems, which is developing a commercial version. Hillman’s team is also developing a well-documented, simplified SCAPE system to enable other labs to build their own.
RNA barcodes for tracing neurons
Neuroscientists have developed numerous ways to tag neurons and visualize their connections across the brain. But most of these approaches have an inherent limitation. Each neuron must be tagged with a different label, and techniques that employ fluorescent markers can resolve only a certain number of colors. Xiaoyin Chen, an SCGB fellow in Tony Zador’s lab at Cold Spring Harbor Laboratory, described two new techniques for tracing neuronal projections, MAPSeq and BaristaSeq. Both use labels based on RNA rather than fluorescent markers or dyes to trace neuronal projections. “There is no tracing involved and no theoretical limit on diversity,” Chen says.
For MAPSeq, researchers inject barcodes — 30 letter stretches of RNA — into part of the brain. The barcodes enter neurons and diffuse down their axons. To determine where these neurons project, researchers dissect brain regions of interest and search for barcodes from target neurons by sequencing the RNA. “It turns the projection tracing problem into a sequencing problem,” Chen says. “Sequencing is fast and cheap.”
MAPSeq requires scientists to remove and analyze segments of brain tissue, destroying fine scale detail. So Chen developed a technique called BaristaSeq, in which barcodes are sequenced in intact brain slices. This approach preserves anatomical information and allows scientists to examine connectivity at greater resolution.
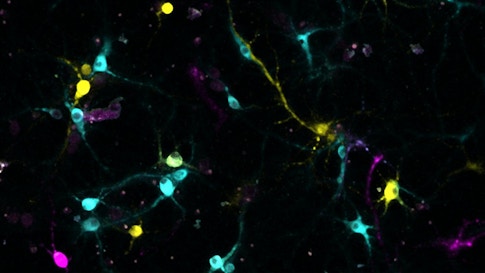
BaristaSeq employs the same sequencing reaction and reagents as Illumina sequencing machines, a popular commercial sequencing technology. But the reaction is done in a brain slice under a microscope. Each letter of the barcode — A, T, G and C — emits a different color during the sequencing reaction, producing a color-coded version of the barcode’s sequence. BaristaSeq is much faster than traditional tracing techniques; Chen says he can process 1,000 to 3,000 cells per brain in two weeks.
So far, Chen has used the techniques to identify different types of neurons in the auditory cortex. He first confirmed the technique works, using MAPSeq data to identify known types of projection neurons. He then used BaristaSeq to further subdivide these categories and examined whether cells that project to similar brain areas reside in similar laminar layers. He found that neurons from the same place tend to project to the same collection of regions.
Large-Scale collaboration at the International Brain Lab
Anne Churchland, a neuroscientist at Cold Spring Harbor Laboratory, described the International Brain Lab, a collaboration among 21 different labs across the United States and Europe. Funded by the SCGB and the Wellcome Trust, the IBL aims to uncover key neural computations that underlie decision-making. Researchers will employ the same decision-making task across all labs, enabling them to compare and combine results.
The collaboration has an ambitious agenda. In five years, IBL members want to produce a brain-wide activity atlas of decision-making and a brain-wide theory of decision-making, as well as mechanisms for data sharing. In the process, they hope to launch a new way of doing neuroscience.
“A project of this size sounds daunting, but several core elements will support its success,” Churchland said. These include a shared behavioral paradigm, a first in neuroscience, as well as immediate data sharing, routine replication of results, and close collaboration between experimentalists and theorists, a central theme in all SCGB projects.
To make it possible to compare results across labs, IBL members will standardize every aspect of their experiments, from the behavioral apparatus, mouse strains and husbandry to the neurophysiology and data acquisition hardware, histological procedures, data formats, storage and analysis code. The data the IBL collects will be available to all SCGB investigators.
The researchers will measure neural activity using both calcium imaging and Neuropixel probes, a new technology that can simultaneously record activity from hundreds of neurons in different parts of the brain. “We will divide the brain and each lab will work on a specific part,” Churchland said.
Each brain area will be monitored by more than one group, so the groups can replicate results. That will be inherently challenging, because neural signals vary from animal to animal, Churchland notes. “Deciding what constitutes replication is difficult; we will need to develop this,” she said.
The IBL team includes a range of theoretical and computational expertise, including biophysics, cognitive behavioral modeling and the statistics of neural data analysis. This diversity will allow the IBL to build models of the decision-making process at a variety of levels of detail, as well to exploit cutting edge statistical analysis and machine learning tools on its rich datasets. Researchers hope that close coordination of theorists and experimentalists to will accelerate the pace of the model-hypothesis-experiment-analysis research cycle.
The scale of the collaboration, unprecedented in neuroscience, will likely raise a number of issues in the practice of science. For example, Churchland noted that people often ask how they will attract postdoctoral researchers, who need high profile papers to get subsequent academic positions, into this type of group endeavor. Churchland said that because the project expects to produce so much data, postdocs should have the opportunity to produce both traditional and consortium style papers. She cited other examples in other fields, particularly from genomics, where large consortiums publish papers with many authors.
Additional reporting by Brian DePasquale.