
Finding Signals in the Noise with HumanBase
What once seemed impossible — sequencing an entire genome — is now fast, routine and cheap. With sequences from hundreds of thousands of human genomes now available to scientists, as well as growing catalogs of information on genes and their functions, researchers have a new problem: an overwhelming abundance of data that outstrips the traditional tools of analysis. Indeed, analyzing data — not generating it — has become the greatest challenge facing biologists today.
“In human biology, we have knowledge hidden in datasets,” says Olga Troyanskaya, deputy director for genomics at the Center for Computational Biology (CCB) at the Flatiron Institute and a professor of computer science at the Lewis-Sigler Institute for Integrative Genomics at Princeton University. But the deluge of human data demands a different approach than the ones used in traditional genetic studies of yeast and worms, for example. Model organisms such as these are amenable to controlled experiments in which scientists can engineer a genetic mutation in one population and compare the effects against a population without the mutation. By comparison, genomic data for humans contains far more complexity — and statistical noise — which has limited what scientists can tease from it. “We were inspired to come up with a resource for human genomics as comprehensive and powerful as what we had for model organisms,” says Aaron Wong, a data scientist and project leader at CCB.
Enter HumanBase, officially launched in 2018. HumanBase is an interactive platform that digs through data from 61,400 experiments from 24,930 publications to make predictions about how genes in specific tissues in the body are turned on, what they do and how they interact with each other. The results allow researchers to explore the genetic underpinnings of how diseases emerge and manifest differently from one tissue to the next. “It’s a one-stop shop for data-driven predictions in human molecular biology,” says Troyanskaya.

Since its launch, HumanBase has radically changed biologists’ ability to unearth discoveries from data. “When I started as a biologist, I preferred model organisms because humans were too hard to study. Because of the data we have and the ability to study it with HumanBase, humans are now more like model organisms,” says Chandra Theesfeld, a research scientist in Troyanskaya’s lab.
HumanBase’s capacity to make sense of complex human molecular data with the same level of precision achievable with model organisms represents a significant boon to the field of genetics. HumanBase has also bridged the gap between computational and experimental biology — two disparate areas of research that often remain siloed from each other. This is reflected in the collaborative approach exhibited by the representatives from both camps who make up the HumanBase team. For example, while the HumanBase computational scientists don’t need to know how to perform experiments, many have found this knowledge to be a critical tool for building HumanBase. Chris Park, a research scientist in the genomics group at CCB, became a postdoctoral fellow in an experimental biology lab at Rockefeller University after completing his doctorate in computer science at Princeton under Troyanskaya’s supervision. “I wanted to see how data is generated in the lab, to ultimately make better models using computational and statistical tools,” says Park. “I had to learn a specific way to wash my hands, and I was dissecting my own mouse brains.”
Others on the team are primarily experimental biologists, like Theesfeld. “I’m often the first user of any HumanBase method,” says Theesfeld. “I ask, ‘How can we help biologists? What would I do once I had this prediction?’ and that helps inform interface and feature development.”
The researchers agreed HumanBase could not have been developed anywhere but the Flatiron Institute. “We knew we needed top-notch machine learning and industrial-quality software that was professionally maintained,” says Troyanskaya, “and at the same time, we think a lot about how biologists ask questions, which is nontrivial. Flatiron is the unique place [where] you can do something like this.”
Indeed, the recent explosion in machine learning capabilities laid the foundation for HumanBase’s success. Machine learning methods are uniquely equipped to handle large datasets and to leverage the recent profusion of human genomic data. They can comb through data in ways that scientists cannot, in record time, and home in on connections that may be faint in any one dataset but stand out clearly across myriad datasets. By viewing individual data points as part of a network, machine learning can, in essence, see both the forest and the trees. This is how machine learning identifies biological associations from raw data, rather than relying on existing knowledge, for example, that a particular gene is associated with a disease. “We are not mining PubMed,” says Troyanskaya, referring to the centralized database of scientific and medical research publications, “but instead digging through mountains of data to uncover new knowledge.”
The machine learning algorithms and deep learning neural networks employed by computational biologists have long been inaccessible to biologists working in the lab, but Wong hopes that, with platforms like HumanBase, this will not always be the case. “There’s a huge unmet need to translate machine learning into something you can use at the bench,” he says.
Ultimately, the developers are optimistic that HumanBase will serve as a central resource for biologists, who can use the results to generate hypotheses for the next round of lab experiments. “We hope using HumanBase becomes standard practice,” says Theesfeld. “In the same way you wouldn’t publish a paper without consulting PubMed, you wouldn’t do an experiment without HumanBase. Thirty minutes of work on HumanBase can save you months, if not years, in the lab.”
COVID-19 and Kidney Disease: An Unexpected Connection
Since its launch, HumanBase has proved strikingly adept at navigating biology’s thorniest problems. Most recently, it was used in a study investigating why people with diabetic kidney disease (DKD) show increased susceptibility to infection with SARS-CoV-2, the virus responsible for the deadly COVID-19 pandemic.
Since the virus was first identified last year, there has been a rush of activity to understand how it wreaks such havoc on the human body. Researchers made some headway early on when they discovered that a cell-surface protein called ACE2 binds with SARS-CoV-2, allowing the virus to enter cells — like a key unlocking a door. Researchers set out to investigate what was different about kidney cells with increased ACE2 expression. In cells from patients with DKD and from patients hospitalized with COVID-19, the researchers found thousands of genes that showed increased expression in tandem with ACE2 expression — hinting at a potential connection. They reported these findings last month in Kidney International.
“One way to interpret these results would be to dig through the literature, one gene at a time,” says Theesfeld. Instead, HumanBase provided a far more efficient way forward. It first found relationships between all the genes, then constructed networks of those relationships, and finally displayed the results in weblike maps showing how each gene was associated with the others, as if they were in a circuit. In this way, HumanBase identified clusters of genes, called modules, that have common functions in the cell. This strategy, called functional module detection, can analyze up to 4,000 genes at once. It can even suggest a function, or set of functions, for a previously uncharacterized gene. In the kidney study, functional module detection had already yielded connections between gene clusters and biological processes related to viral entry, replication and immunity. Importantly, the modules from both patient groups — those with DKD and those with COVID-19 — overlapped with each other and with modules generated from functional analysis of published data on genes relevant to SARS-CoV-2. An association between kidney disease and viral processes is intriguing, and scientists plan to test the association through experiments on kidney tissue in vitro. And because some of the genes identified in the study are targets of diabetes drugs, further experiments can probe the potential of known therapeutics to affect susceptibility to and progression of COVID-19.
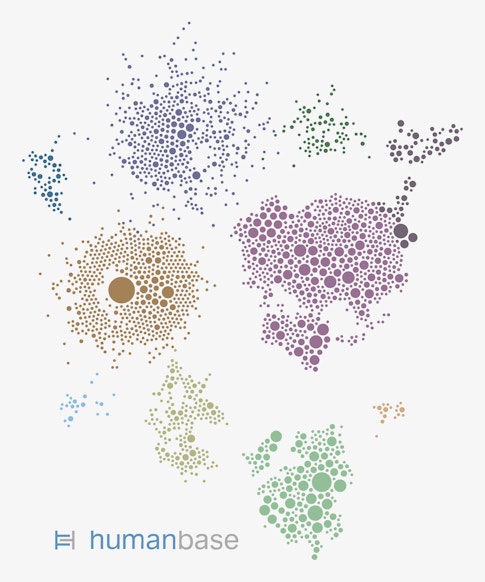
Julien Funk, a software engineer in the genomics group at CCB, led the effort to build HumanBase’s functional module detection. “Designing a dynamic, interactive visualization is quite different from designing the static visualizations you see in most published material,” says Funk. “I worked with biologists like Chandra [Theesfeld] to understand what aspects of the visualization were important from a scientific perspective,” such as the ability to see how genes in one module connect to genes in other modules, and to access increasingly specific views in which the user can view all the modules, zoom in on one module, and then zoom in on a gene within that module.
Some of the challenges in designing functional module detection were surprising, says Funk. “At one point when I showed the team a uniform, nicely laid-out module, they said biologists might not trust such a clean-looking layout of data, and of course we needed people to trust it.” Funk then focused on showing the biological connections, without worrying about making it look clean. The result of this iterative work is a visualization the community finds informative, intuitive and accessible, says Theesfeld. In the COVID-19 kidney study, for example, she adds that “instead of looking at lists thousands of genes long, we can visualize the data in terms of clusters and cut through the noise by bringing it to the level of the biological process.”
A Deeper Understanding of Autism and Beyond
The functional module detection strategy grew out of a 2016 paper published in Nature Neuroscience with Theesfeld, Wong and Troyanskaya among the authors. The paper showed how 2,500 autism-associated genes clustered into brain-specific modules, driving functions related to embryonic development, the senses and movement. Importantly, the modules contained genes that were not previously known to be linked to autism.
However, many DNA mutations that appear in the genome of a child with autism but not in those of the child’s parents or siblings — called de novo mutations — can’t be tied to a particular gene. Instead, they are found in the so-called noncoding regions of the genome. Scientists suspect noncoding regions play a role in the disorder by regulating how genes are turned on and off, and at what intensity, like a dimmer on a light switch. Unlike the clear correlation between DNA sequences, amino acids and proteins one finds in the coding region of the genome, the relationship between DNA sequences in the noncoding region and the regulation of genes was murky. Understanding this relationship would require figuring out the rules that link patterns in the DNA sequence with their functional impact on gene regulation in autism, and then finding these links, scattered loosely throughout the genome, with statistical power.
The HumanBase team tackled the problem with DeepSEA. A deep learning approach that is part of the HumanBase suite, DeepSEA can predict how DNA mutations in noncoding regions affect gene expression, at single-nucleotide resolution. Instead of relying on prior observed sequences important in turning genes on or off, or up or down, DeepSEA employs a deep learning model that learns how DNA sequences regulate genes — a process similar to learning to read rather than memorizing a set of words. From sequence alone, DeepSEA estimates the ability of particular proteins, like transcription factors, to bind to DNA and ultimately affect the expression of a nearby gene. Additionally, DeepSEA’s disease impact score estimates the likelihood that a particular DNA mutation plays a role in disease.
The Simons Foundation has long contributed to autism research through its autism research initiative, SFARI. SFARI has assembled 2,600 families into a group called the Simons Simplex Collection (SSC), a cohort in which one child is affected by autism and the parents and siblings are unaffected. Whole-genome DNA sequencing on nearly 2,000 families from the SSC identified de novo mutations for analysis with DeepSEA. DeepSEA discovered that children with autism had more mutations in the noncoding regions than controls did, with a higher functional impact on autism gene regulation. “We were excited, but cautious at first,” says Park. “Then we saw the brain was the most highly ranked tissue associated with the mutations.” In addition, many of the genes affected by the higher-impact noncoding mutations were also affected by previously identified high-impact coding mutations.
In an echo of the visualization challenges of functional module detection, the researchers found it difficult at first to present and explain the results of DeepSEA’s autism predictions. To maximize the impact of their findings, Park, Wong and the HumanBase team developed an interactive autism spectrum disorder browser within the HumanBase platform showing the location and predicted effect of nearly 130,000 autism-related de novo mutations throughout the genome. A link between specific mutations and physical manifestations of the disease may help to cluster patients with similar profiles. For example, some of the noncoding mutations might explain the variation in IQ seen in patients with the disease. Patient clustering could be important in the development of therapeutics targeting specific populations within the autism spectrum.
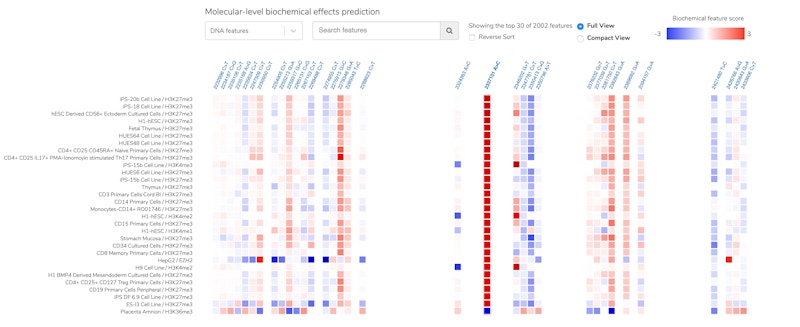
A Biology-Centric Computational Approach
“We know the noncoding region isn’t ‘junk DNA,’ but until very recently we haven’t had a way to make predictions with single-nucleotide resolution,” says Troyanskaya. Another HumanBase feature called ExPecto can predict tissue-specific gene expression from a given DNA sequence. “Theoretically you could take a piece of Neanderthal DNA and see which genes are more or less expressed compared to humans, according to tissue type,” says Troyanskaya. “The ability to make these predictions from sequence is a game changer.”
Keeping HumanBase nimble so it can incorporate new kinds of data as genomics technology accelerates in pace and resolution will be a challenge, says Wong, but a welcome one that will enable researchers to ask new kinds of questions. Efforts are also underway to make HumanBase even more user-friendly. “I’m planning a quick-start guide to help newcomers to HumanBase understand the context of each tool and how it might be useful in their research,” says Funk. Although HumanBase is meant to be easy to use, Park wants users to understand that the results can be plugged into additional advanced computational tools, if desired. “This is a resource for biology, with the perspective of biologists baked in,” he says.