
The Challenges of Proving Predictive Coding
Predictive coding — a theory of how the visual system works that focuses on comparing incoming information with an internal prediction — has been around for decades. Experimental explorations of this theory, however, have returned a mixed picture. Some scientists pursue the theory eagerly and find strong support for it. Many others make progress completely ignoring it, working under an entirely different model of the visual system.
Yet as the theory has gained steam in recent years, it is harder for the two sides to work peacefully in parallel without an interaction. For some skeptics, digging into the evidence has convinced them the theory has some merit. For others, testing the theory and finding null results has cemented the belief that predictive coding is not at play in the visual system. Despite this increased attention, hard and fast conclusions still seem elusive. More work — especially the development and use of methods for recording activity throughout the visual system, and models that explore the benefits of predictive coding — will be needed before this debate can be put to rest.
The most standard view of how the visual cortex processes information is ‘bottom-up.’ Cells in the primary visual cortex, V1, get input from the thalamus. By identifying patterns in thalamic firing, these cells respond to the presence of simple lines in their visual field. They then send connections to the secondary visual cortex, V2, which itself looks for patterns in the firing of the primary visual cortex, and responds to various corners and complex edges. The process repeats with more and more visual areas. In this way, a hierarchy is formed where cells respond in a ‘bottom-up’ way to objects of increasing complexity in the visual world.
Predictive coding flips this system around. Instead of cells representing exactly what they see in the world, predictive coding posits that the brain makes predictions about what it expects to see, and a cell’s response is shaped by whether that prediction is correct or not. While the theory is an interesting reimagining of sensory processing, it has drawn fire from scientists who find it ill-defined and unsupported. “There’s a lot of skepticism in certain sectors of the field,” according to Redmond O’Connell, a neuroscientist at Trinity College.
One of the earliest theories of predictive coding was put forth in a 1982 paper by bioengineer Mandyam Srinivasan and colleagues. The paper posited that lateral inhibition in the retina serves to cancel out predicted inputs, with the prediction based on an assumption that nearby ganglion cells will receive similar inputs. The idea was inspired by the way digital video files are transmitted: Only information about pixels that deviate from their expected value based on previous frames needs to be sent forward. The authors believed that the efficiency of such a strategy would also make it well-suited as a design for how information gets sent out of the retina.
Perhaps the most common account of how predictive coding could be implemented in the visual system, however, comes from a 1999 paper by Rajesh Rao and Dana Ballard. This model makes full use of the hierarchy of visual processing. In it, neurons at one layer of the visual hierarchy send connections back to the layer below that carry predictions about the activity at that layer. Those predictions are compared with what is coming into the layer from the bottom up, and an error is calculated. This error — rather than the full bottom-up information itself — is what gets sent forward to the next layer.
Rao and Ballard used this model to explain some widely observed features of visual neural responses. For example, cells that fire strongly for a stimulus in their receptive field will have a weakened response if that same stimulus extends slightly beyond it — into an area known as the extraclassical surround. In a predictive coding framework, the presence of the stimulus in the surround makes its presence in the center more predictable, and therefore there is less of an error in prediction for the neuron to signal; thus the firing rate is lower.
A siloed theory
The Rao and Ballard model forms the inspiration for much of the inquiry into predictive coding today. While a sizable population of vision scientists have searched for evidence for this scheme in the brain, they’ve still struggled to displace the standard bottom-up view.
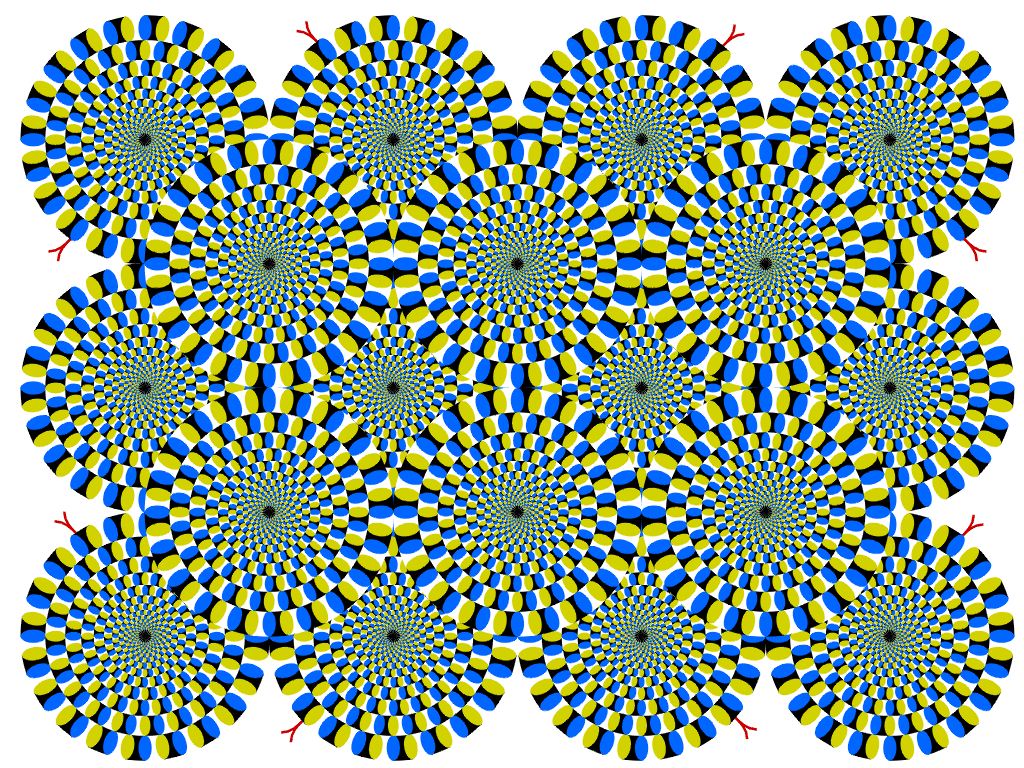
Predictive coding research is “siloed,” according to O’Connell, particularly as much of it has been performed in labs focused on human neuroimaging rather than electrophysiology. O’Connell has written a recent review with first author Kevin Walsh summarizing the evidence for and against the main tenets of predictive coding from a range of labs and experiments.
As a result of this siloing, it can be hard to get an objective sense of where the evidence lies. Proponents of the theory can focus on experiments where signs of predictions can be found; everyone else can carry on assuming the standard model of visual processing. Both sides can even point to the same evidence to support its own side at times.
O’Connell actually approached his review as one of the skeptics. “Predictive coding wasn’t part of our everyday discussions in the lab,” he says. In a rare reach across the aisle, he brought on Andy Clark, a philosopher at the University of Sussex and a known supporter of predictive coding, to ensure his look into the topic would be balanced. “We consciously tried to be as open-minded as possible,” O’Connell says.
O’Connell found that, while many of the historical studies claiming to show evidence of predictive coding only weakly did so, more modern approaches have yielded stronger support. For example, a 2017 study found neurons in a region of the face patch system that responded to unpredicted face images in a manner consistent with the region’s location in the face-processing hierarchical. On the whole, however, results are still mixed.
One of O’Connell’s main conclusions from this investigation, however, was just how difficult it can be to test predictive coding. There are many different variants of the theory, and they can be mapped to neural activity in different ways. Therefore, coming up with a precise prediction for what to see in neural data is not trivial. What’s more, many assumed signs of prediction errors can actually be explained by slight modifications to the standard bottom-up view. “It’s not a simple matter to dismiss or prove this theory,” says O’Connell.
When the same stimulus is repeatedly shown, for example, the neural response to it begins to diminish, a phenomenon known as repetition suppression. Under predictive coding, this decrease in firing is interpreted as a decrease in prediction error: A repeated stimulus becomes very predictable. Yet this effect can be equally well explained in the bottom-up framework by assuming the presence of neural adaptation. That is, through cellular mechanisms such as synaptic depression, repeated inputs become less effective at driving a cell. There is therefore no need to abandon the standard view to explain this finding.
In search of prediction error
It was with this backdrop that Selina Solomon and the lab of Adam Kohn, a Simons Collaboration on the Global Brain Investigator at the Albert Einstein College of Medicine, embarked on a study to test the theory. “There has been a big debate about whether repetition suppression reflects predictive coding,” says Kohn. In their experiments, they wanted to do a thorough test of predictive coding that would require different types of predictions, rather than simply repetition suppression. The work tests if “there is some kind of more sophisticated internal process,” says Kohn.
In their study, the Kohn lab used a simple series of images to induce in their subjects — both rhesus macaques and humans — an expectation of what would come next. Specifically, the macaques viewed a series of oriented gratings that almost always had the same order. For example, in one experimental session, they may see five gratings in a row with angles of 90 degrees, 90 degrees, 90 degrees, 0 degrees and 90 degrees. The assumption is that, if the subjects see this sequence over and over, they will be able to predict the orientation of the grating that comes next. If their expectations are violated, then predictive coding would say there should be a noticeable prediction-error response.
To test this, the authors occasionally showed a different sequence of gratings. For example, they could replace the third 90 degree grating by a 0 degree one. When they did so, cells in both V1 and V4 responded the same way as when they saw the 0-degree grating in its normal position. That is, there was no prediction error, despite the fact that a prediction had been violated.
When the authors replaced the 0-degree grating in the fourth position with another 90-degree grating, cells responded slightly less than they did to the previous 90-degree grating they saw. This decrease in response is exactly opposite of what predictive coding predicts: An unexpected 90-degree stimulus should produce a large prediction error. Yet it is perfectly in line with adaption: More of the same stimulus causes a decrease in response.
The authors varied several parameters of the experiment to ensure their results were not specific to the details of these gratings. For example, they used stimuli of different contrasts, changed the length of the sequences, and trained the animals on the expected sequence for a longer period of time. In all cases, however, the vast majority of cells showed responses inconsistent with predictive coding. In fact, the only way the investigators could elicit a signal that looked reliably like a prediction error was when they explicitly instructed their human subjects to respond when a sequence deviated from the norm. Using EEG recordings from these subjects, they observed a stronger response when a deviant stimulus was seen. This difference wasn’t there, however, when participants were passively viewing the same sequences.
This search for prediction errors thus largely came up empty. Kohn was not surprised by these null results, however. In fact, he found it fun to write a different kind of paper: “A lot of neuroscience is, you find something and magnify it and overinterpret it,” Kohn says. But not finding an effect was welcome. When studies work according to plan, he says, “they don’t actually provide an interesting outcome.”
Getting the research community to accept a negative finding can be a challenge, however. “The field of predictive coding, like many fields, is very ideological,” says Kohn. “The people who tend be reviewers for papers on the topic like it.”
A proxy for prediction
The work was “very nicely done,” according to Georg Keller, a neuroscientist at Friedrich Miescher Institute for Biomedical Research in Switzerland who studies predictive coding in mice, and who was not involved in the Solomon-Kohn study.
Yet for Keller, asking whether neurons encode a prediction error for sequences of images they are simply viewing passively is not the right question. “There is not such a thing as a prediction error. Prediction error of what?” While the stimulus is easy to control, the key challenge for Keller in testing predictive coding is knowing what the predictions are. “We need a proxy for what we think the animal is predicting. Movement is a much better proxy,” according to Keller, because “ethologically relevant stimuli are more likely to be predicted.”
Keller’s lab has explored how visual responses are modulated by an animal’s own behavior. For example, they showed that the primary visual cortex receives inputs from motor areas conveying information about the expected visual flow that should come from self-movement. He has also fleshed out a way for local cortical circuits to calculate prediction errors.
Keller’s work taps into a long history of studying what is known as an efference copy. Efference copies occur when a part of the brain responsible for generating movement sends a copy of that information to sensory areas to allow those areas to cancel out the impact of the action.
A canonical example of an efference copy can be found in the African mormyrid weakly electric fish. These fish produce and sense electrical signals in order to communicate with others and to detect prey. The production of their own electrical discharge, however, can interfere with their ability to sense their surroundings. When the motor command to produce a discharge is sent, therefore, a copy is also sent to the systems responsible for sensing electrical signals. By canceling out the predicted sensory effect of their own discharge, they can continue to receive information from the environment. The brain area responsible for this prediction and cancellation is the electrosensory lateral line lobe, a hindbrain structure similar to the cerebellum in mammals.
While this is in many ways a clear example of predictions canceling out expected bottom-up input, work under the banner of predictive coding frequently focuses on the cortex, not the cerebellum. To Keller this is mainly a matter of what is being predicted. “Where errors are easy to calculate, use the cerebellum. For harder, more complex transformations, cortical circuits are likely engaged,” he says.
Many meanings
The fact that expectations from passively viewing images and the cancellation of expected optic flow from movement both fall under the same name supports a claim made by both O’Connell and Kohn: that predictive coding means many things — perhaps too many for it to be useful as a unified theory. “The term is not used the same way by different groups,” says Kohn. After all, the original Rao and Ballard model was meant to explain predictions over space at one point in time — yet many studies of predictive coding today look at predictions over time.
This diversity may partially explain why Kohn’s group found few signs of prediction errors where others have found several. And tests of predictive coding have spanned a wide range of species, sensory systems, recording methodologies and task designs. Understanding how these different results relate is like being given pieces from multiple different jigsaw puzzles and trying to fit them together. It is hard to come to any concrete conclusion. Indeed, Kohn recognizes that this single study does not disprove predictive coding. “Absence of proof is not proof of absence,” he says.
So what is the way forward for predictive coding? How can all the seemingly conflicting evidence be resolved? The answers can be as diverse as theories of predictive coding itself.
Kohn advocates an empirical direction: “Rather than strengthening predictive coding as a theoretical framework, we should record simultaneously across multiple stages of the visual system.” Even scientists not fond of predictive coding as a theory acknowledge that there are many top-down connections in the visual system, the functions of which are not entirely clear. “We’re in a phase where we need a basic characterization of the flow of signals between stages of the visual system,” Kohn says.
Keller believes the theory does need work. “I’m quite sure it’s wrong. But I think it is wrong in interesting ways,” he says. One exciting direction for him is to think about the role of prediction errors in learning. Several recently developed algorithms in machine learning have made progress using schemes inspired by predictive coding. Keller believes that understanding how those algorithms work may inspire new takes on predictive coding in the brain.
Keller also acknowledges the need for new experimental methods to really test the specifics of the theory. Because predictive coding posits sets of cells with different functions — i.e., those representing predictions and those representing errors — the ability to selectively record from and modulate different cell types is crucial. Keller’s lab is working to develop genetic tools to do just that.
O’Connell has come around to predictive coding as he has delved into it, but he is skeptical it will provide a unifying single-process theory of sensory processing, a goal he refers to as a “potentially insurmountable challenge.” Rather, he believes there are elements of the theory that may be correct, and that we should simply take what works.