
Molecular Clocks Offer New Insight into Aging
As we grow old, our memory may falter and our muscles may weaken — but how these changes unfold varies from person to person. On the molecular level, however, one consequence of aging is surprisingly consistent: The activity of our genes will become less tightly regulated than it is in our youth, due to a vast reshuffling of gene-regulating markers on our DNA.
This change involves small chemical tags known as DNA methylation marks, which are distributed by the millions throughout our genome and often silence gene expression. They are one form of the heritable changes, known as epigenetic modifications, that determine how cells use their genes. As we get older, methylation marks shift, fading from some spots and becoming more abundant in others. Some of these changes accumulate steadily enough that monitoring them has proven to be a reliable way of assessing age.
More than a decade ago, with new technologies for tracking epigenetic marks, scientists developed mathematical models known as epigenetic clocks, which use age-dependent methylation changes to estimate age. By analyzing dozens or hundreds of potential methylation sites, an epigenetic clock can usually identify a person’s chronological age within just a few years. In terms of predicting age, “these things work brilliantly,” says Jonathan Mill, a genomicist at the University of Exeter. “You could take a blood sample from anyone, and you could run it through a methylation array, and amazingly accurately, plus or minus a couple of years, you could say how old that person is.”
Now that it’s well established that DNA methylation is a useful indicator of age, investigating the cellular pathways that are represented by the clocks and what they reveal about aging mechanisms has become the “real frontier” of the field, says Trey Ideker, a cancer biologist at the University of California, San Diego, whose lab built one of the original clocks in collaboration with UCSD colleague Kang Zhang.
Since the first epigenetic clocks were developed 10 years ago, scientists have developed dozens of new versions, constructed from different methylation sites and fine-tuned to different purposes. Researchers are comparing these clocks to find clues about what is universal about the aging process. Their analyses point toward genes that may be particularly vulnerable to aging or active drivers of the aging process — including many known to be important for the brain’s development and function.
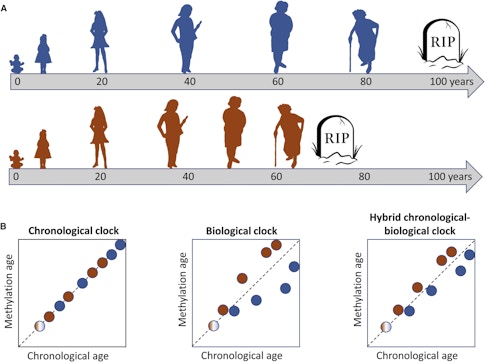
Scientists are also exploring how to use epigenetic clocks to study brain aging specifically — but many questions remain. Most epigenetic clocks were developed using data from accessible tissues, often blood samples, and it’s unclear if such tissues will be reliable indicators of cognitive aging. Clocks developed from other tissues tend to underestimate chronological age when used to analyze brain tissue. This could indicate that epigenetic aging proceeds more slowly in the brain, though scientists don’t fully understand why. To more precisely monitor aging in the brain, researchers have developed new brain-specific clocks that have created new opportunities to investigate aging’s relationship with neurodegeneration.
Predicting age
DNA methylation is a dynamic and influential regulator of gene expression in mammalian cells. The distribution of methylation marks varies in different cell types and at different stages of development, and these patterns are replicated and passed on when cells divide. A lot of information is packed inside those methylation marks, which fundamentally change the way individual cells use the genome, says Christopher Bell, a computational epigeneticist at Queen Mary University of London who is using the methylome to investigate pathophysiology associated with diabetes and obesity.
An assortment of enzymes busily adds and removes methyl groups as new DNA is synthesized and when cellular needs change. But as we age, the processes that manage these modifications become less faithful, leading to some epigenetic disarray. “We’ve always had this concept of random drift, where with age, you’re moving away from the set points that you should have,” Bell explains.
Over time, methylation marks appear in regions of the genome that should be unmethylated, and disappear from the areas where they should be. But while the overall trend is toward entropy, Bell notes that some specific methylation changes are predictable. This is at least partly due to age-related changes in tissues’ cellular composition, like changing numbers of neurons and glia in the brain, since different cell types have distinct, identity-defining DNA methylation patterns. Beyond this, little is known about why some segments of DNA become consistently more methylated with age and others consistently less so.
The first epigenetic clocks were reported in 2011, when new microarray technology facilitated large-scale analyses of the methylome. At first, the relationship between methylation patterns and age seemed to be a nuisance, says Ideker. Both his lab and Zhang’s began using methylation arrays to look for patterns in the blood of cancer patients that might signal the presence of disease. But graduate student Gregory Hannum found that the strong correlation with age overshadowed other associations in the data.
Ideker recalls Hannum’s frustrated report at a lab meeting: “He says, ‘Guys, I just can’t find any cancer signal. You know why? Because that damn age is such a pesky covariant. So many of the methylation marks just track age, and I can’t get rid of that signal.’ There was a pause, and we said, ‘You know, that’s interesting.’ ”
Shifting their focus, Ideker and colleagues found that by checking the methylation state of just 71 DNA sites, they could determine a patient’s age from their blood sample. They did this using machine-learning algorithms that analyzed their data to identify which of the genome’s potential methylation sites were most useful for predicting an individual’s age, as well as what weight each of those sites should carry in the age calculation. “Then you basically take those sites, you multiply each by a weight, you sum the whole thing up, and that’s your age,” Ideker says. Tested against additional samples, the method, which became known as the Hannum clock, identified subjects’ chronological age within five years.
Soon after, Steve Horvath’s team at the University of California, Los Angeles, used a similar strategy to find age-associated methylation changes in 51 types of human tissue. Their findings led to the Horvath clock, which assesses age by tracking 353 methylation sites throughout the genome and works with similar accuracy in a range of tissue samples.
A slew of studies have used the Hannum and Horvath clocks to tie accelerated epigenetic aging to conditions from heart disease and cancer to depression, Alzheimer’s disease and chronic pain. Likewise, some studies have picked up links between accelerated epigenetic aging and declines in cognitive function and structural changes in the brain.
Common changes
During training, machine-learning algorithms zero in on a small subset of the thousands of methylation sites in the genome that change with age, selecting those that are most useful for estimating age within a particular group. By training their models on different sets of samples, and even changing the way age is defined, researchers have developed epigenetic clocks for different purposes.
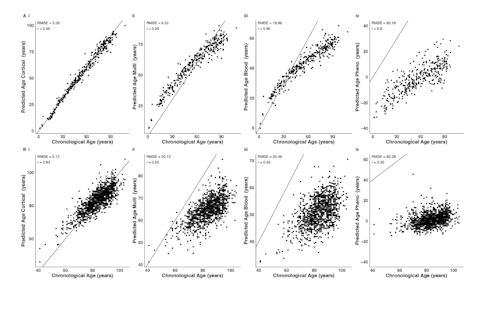
The first generation of clocks was designed simply to predict chronological age. Some new clocks take a more nuanced approach by aiming to estimate biological age — a more elusive but likely more meaningful measure. Everyone adds years to their life at the same rate, but biological aging is variable: a complex composite of age-related changes that are influenced by our lifestyle, genetics and environment. There is no single agreed-upon measure of biological age. Epigenetic changes are one indicator. So are shortening telomeres, physical frailty, and numerous other molecular and physiological variables.
By calibrating new epigenetic clocks to clinical measures of aging, researchers have found that they can better represent biological aging and more accurately predict outcomes like disease, cognitive decline and mortality. For example the PhenoAge clock developed by Morgan Levine, a biologist at Yale University, in New Haven, Connecticut, and Steve Horvath was trained to predict a measure of biological age that incorporates nine clinical biomarkers, such as creatine levels and white blood cell counts, as well as chronological age. Because PhenoAge and other “second-generation” clocks appear to better represent biological age than the first-generation clocks, they may be more informative for mechanistic studies that aim to understand aging in order to slow or prevent its effects.
While the exact composition of every epigenetic clock is unique, certain sites within the genome seem particularly susceptible to age-related methylation changes. Identifying methylation sites that consistently change across different types of aging tissues or in different mammalian species may be particularly informative when it comes to teasing out the biological mechanisms that underlie aging.
Developmental genes targeted by the epigenetic regulators known as polycomb group proteins are frequently represented in epigenetic clocks, including targets of polycomb repressive complex 2, which influences stem cell differentiation, neuronal identity and microglial activity in the brain. Other genes that are well represented in epigenetic clocks and that may be particularly relevant in the brain are ELOVL2, which encodes an enzyme involved in fatty acid synthesis, and the neuropeptide somatostatin.
Clocks designed to work across species may be particularly useful for elucidating what is universal about aging. Last year, Ideker’s lab built an epigenetic clock that predicts age using blood samples from both dogs and humans. And Steve Horvath and colleagues report on bioRvix that they have developed three different pan-mammalian clocks, using data from 59 tissue types and 128 species. The methylation sites selected for these clocks hint at conserved aspects of the aging process, and could be valuable leads for investigating the mechanisms behind age-related decline.
Developmental gene networks are heavily represented by the methylation sites in both Horvath’s pan-mammalian clock and Ideker’s human/dog clock. Ideker notes that developmental genes were not prominently represented in the original human epigenetic clocks. “Those don’t uncover this because they just grabbed in the bag and found some really good sites at predicting age in this particular cohort,” he says. Forcing the machine-learning algorithms used to train the clock to find features that are conserved among species is likely to generate more functional insights, he says.
Instead of focusing on specific genes or methylation sites, Levine’s team is looking for functional similarities between existing epigenetic clocks. She thinks it’s likely that most epigenetic clocks are integrating similar kinds of information, even though they use unique sets of methylation sites to capture that information.
Each clock likely uses methylation patterns to report on many different aspects of the aging process, Levine explains. Some methylation changes may be related to cell replication, for example, while others may be a signal of senescence. “When we talk about ‘mechanism,’ I don’t think you can look at the whole clock, because they’re multifactorial,” she says. “There are different kinds of epigenetic changes, and they mean different things for function.” Her group is looking for sets of methylation sites, or modules, that behave similarly across clocks and tissues. By breaking the clocks into functional components and studying each component separately, she hopes to learn both how they work and how to make them better.
A brain-specific clock
To date, most epigenetic clocks have been trained using blood samples, and researchers are exploring how relevant these clocks are to understanding brain aging. It’s likely that some aging processes represented by the clocks are tissue-specific, while others are more universal. The developers of the pan-mammalian clock, for example, note that they found many genes that were affected by age-dependent methylation changes across tissues, but they also identified sites near circadian-rhythm genes whose methylation status changed only in aging brain tissue.
Intriguingly, Ideker’s new human/dog clock includes numerous methylation sites impacting neuroepithelial cell differentiation, as well as the assembly and regulation of synapses. The biological significance of those changes is unclear, but Ideker says he’s eager to learn why neuronal development genes are a core conserved part of a blood-based epigenetic clock.
It’s not yet clear how well methylation patterns in the blood track with aging in the brain. “How fast your blood is aging is not necessarily a good proxy for how fast your brain is aging,” Levine says. But she notes that some processes that accelerate brain aging — such as inflammation — are multisystemic. “So picking up those things in the blood clocks actually can tell us something about the likelihood that your brain is aging faster or slower,” she says.
Broadly, Levine says that multitissue or blood-based clocks tend to underestimate the age of the brain. This may be in part because the clocks pick up on methylation errors introduced during cell division, and most neurons in the brain do not divide. But other differences likely come into play, Mill says, noting that epigenetic aging varies even within the brain itself, with the cerebellum in particular aging very slowly.
Still, Levine says age-related methylation changes are sufficiently conserved across tissues that “You can use the same clock, regardless of the tissue type.” Several researchers have reported “reasonable” correlations to chronological age when various blood-based and multitissue clocks are used to analyze brain tissue.
But in its studies of Alzheimer’s disease, Mill’s group has found that blood- and multitissue-based clocks don’t accurately predict the age of brain tissue. This could be due both to the tissue discrepancy and because their samples came from older donors, whose ages are sparsely represented by most clocks. There is some evidence that the pace of epigenetic aging slows as we get older, so it’s important that a clock be calibrated for the right age span, particularly when studying conditions that manifest later in life, Mill says.
Last year, Mill and colleagues developed the first human brain-specific clock. They trained and tested their clock using nearly 1,400 samples of human cortex from donors between the ages of 1 and 108. The new clock more accurately identified the chronological age of tissue donors using samples of cortical tissue than clocks that are not brain-specific, highlighting the importance of applying the right model to a particular problem. “You really want to be looking in the tissue type — or, even better, the cell type — that’s relevant for the phenotype you’re studying,” Mill says. Using a clock that is not specific to the brain to study the relationship between aging and neurodegeneration could yield misleading results, he cautions.
The new clock gives researchers a more precise way to assess epigenetic aging in studies of donated human brain tissue. Likewise, brain-specific clocks will be useful for studying age-related neurodegeneration and cognitive decline in animal models. A clock developed specifically for estimating age in the mouse brain, for example, can help researchers monitor how neuropathology progresses as epigenetic age increases. With a new Illumina microarray now available for analyzing the mouse methylome, Bell says he expects standardized mouse clocks will become widely used in the coming years.
Another role for the clocks, Mill says, is in characterizing cell models. Preliminary work his group has done with University of Exeter colleague Ellis Hannon suggests that induced pluripotent stem cell–derived neurons have an epigenetic age similar to that of fetal cells. That’s a concern, Mill says: “Cell lines that are being used to model things like Alzheimer’s disease or diseases of cognitive decline in later age maybe aren’t relevant, really, because your model is the age of a newborn.”
Researchers agree that it’s not essential to understand how epigenetic clocks work in order for them to be useful. Some researchers hope it may be possible to develop a clock that predicts an individual’s risk of cognitive decline, and that by providing a way to monitor aging, they will accelerate clinical studies evaluating potential interventions. But if the clocks can also provide insight into the mechanisms that underlie brain aging, it’s worth finding out, says Levine. “By actually understanding their direct link to pathology, I think [the clocks will] be more informative and useful for developing targeted therapies,” she says.