From Statistics to Gerbils: Learning About Neural Connections With Social Rodents
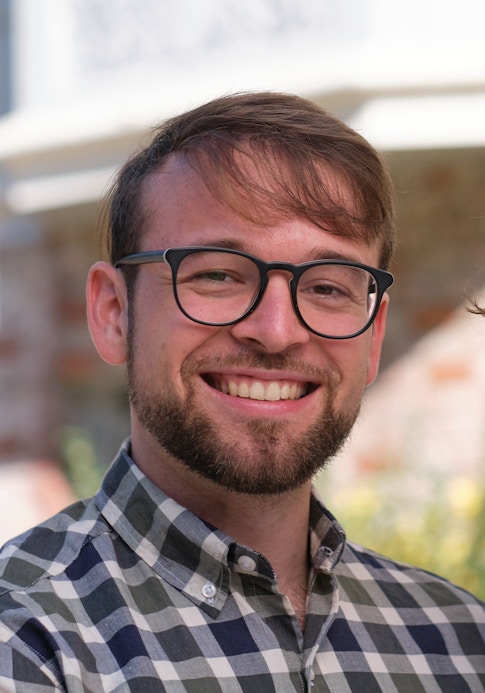
In the classical view of the brain, neurons are assigned specific tasks as we learn. Certain neurons fire as we hear a dog bark, another group is triggered when we recognize a flower. But neuroscientists are learning that this is a simplified view. In reality, a particular stimulus doesn’t always activate the same neurons.
This variability in our neural networks is the focus of Alex Williams, an assistant professor at New York University (NYU) and an associate research scientist and project leader at the Flatiron Institute’s Center for Computational Neuroscience (CCN). In his work, Williams uses statistical models to figure out how neural connections change over time.
Prior to joining the Flatiron Institute, Williams worked at Google Brain, Sandia National Labs, the Salk Institute and Brandeis University. He earned his doctorate in neuroscience and completed postdoctoral research in statistics at Stanford University.
Williams recently spoke to the Simons Foundation about his work with gerbils and how our brain’s neural circuits change over time. The conversation has been edited for clarity.
What does it mean to have variability in neural circuits?
We’ve all had those days where it seems like our brain isn’t functioning at full capacity. Maybe you’re feeling sleepy, or you just can’t find the right words when someone asks you a difficult question. And then there are days where you’re feeling sharp and take everything in stride. On each of these days your brain is more or less configured the same way, but you experience very different levels of performance.
We know some of this has to do with environmental or behavioral factors, like how much sleep you’ve gotten or if you’re offered a reward for completing a task. But there’s also a certain level of natural variability that slowly accumulates over time in neural circuits. While externally it may look like there’s one way to complete a task, there are actually many different approaches your brain can take. You have nearly a hundred billion neurons and trillions of synaptic connections. Your brain can take many paths to get to the correct solution or response. Benign forms of variability in the circuit configuration can build up over time. This variability is called representational drift, and it is another phenomenon we’re trying to study.
Are there any theories on what causes representational drift?
One theory is this drift occurs because your brain is constantly being maintained as pieces grow old, die and are replaced. For example, the proteins your brain cells use to connect with each other turn over on the order of days. This might cause your neural configuration to slowly change over time. But in some preliminary studies we’re seeing representational drift over shorter timescales, so there is likely something else going on as well.
What projects are you currently working on?
I’m working in two areas right now. The first is a big collaboration with my experimental colleagues David Schneider and Dan Sanes at the Center for Neural Science at NYU. Together, we’re studying a species of rodent, the Mongolian gerbil, to understand their natural behavior and how they communicate.
These animals have highly sophisticated social behaviors and a family social structure. We’ve been watching their behavior over the course of a month as new pups grow to adolescence and learn to interact with family members and other gerbils. The gerbils’ social structure is built on vocal communication, and we’re currently studying whether they can recognize the voices of individual family members. Toward that end, we’re going to explore how their brain responds differently to family members versus non-family members. To do this, we’re recording neural signals from the gerbils’ auditory cortex, as well as taking video and microphone recordings from inside their cages.
Currently, we’re working on characterizing the behavior and vocalizations with statistical analyses. The gerbils have a very sophisticated vocal repertoire; a single family can make 10,000 vocalizations a day. We want to be able to predict a gerbil’s response to another family member’s verbal call. With terabytes of data from 24/7 video and microphone recordings, we’re building a statistical model that can automatically identify who made which vocalization. This will allow us to analyze the family’s interactions to predict what vocalization will come as a response. This is just the first stage in this project and I’m looking forward to seeing what discoveries we make with this project in the future.
My second project is developing new models for dealing with neural circuit data, particularly for analyzing datasets of representational drift. These datasets can be, for example, neural data from a mouse navigating a maze. When an animal navigates a maze, we’d expect the same neural circuit activity every time the task is performed. Instead, there’s a variability in the neural circuitry that can’t be explained only by differences in physically completing the task, such as the mouse taking a slightly different path one time. We’re trying to figure out where the variability comes from by developing new mathematical and statistical methods for dealing with the data.
What role do statistical methods like yours play in the neuroscience field today?
Theoretical and computational neuroscience is much more strongly informed by statistics today than it was even 10 years ago. Datasets have become larger, more complicated, more plentiful and publicly shared. As such, there is a strong push for theorists to work directly with experimental data and for experimentalists to integrate their results with sophisticated mathematical methods. Tools from statistics and machine learning — many of which are still being developed and refined — are already a central element of many neuroscientific studies, and I think the importance of these tools will only continue to grow from here.