How Do Our Brains Classify Similar Objects? New Theory Works Out the Details
Distinguishing a cat from a dog is a simple visual task that even young children can do. But exactly how our brains (and artificial neural networks modeled after the brain) achieve this classification has been a complex and longstanding problem.
Now, a new theory developed by two researchers at the Flatiron Institute’s Center for Computational Neuroscience (CCN) in New York City is advancing how neuroscientists understand classification and, critically, the relationships that the brain uses in classifying similar objects.
The new theory, published in July in the journal Physical Review Letters, describes the geometric and statistical relationships in neural networks that underpin classification tasks, such as distinguishing a dog from a cat. The researchers revealed that two aspects of classification that were long considered separate are actually intertwined.
“Our findings could help us better understand how the brain accomplishes object recognition and could help train the next generation of machine learning algorithms,” says Albert Wakhloo, first author on the new paper and guest researcher at the CCN.
“We should be able to use the types of neural representation structure we describe in our research with the goal of making them more efficient and robust in classification tasks,” says SueYeon Chung, senior author on the paper, associate research scientist and project leader of the NeuroAI and Geometric Data Analysis Group at the CCN. Chung is also an assistant professor of neural science at NYU.
In our brains, populations of neurons work together to achieve different cognitive tasks, such as classification. During a classification task, a set of neurons will respond to visual clues such as the shape, color, orientation and size of an object.
Artificial neural networks, a type of machine learning program modeled after the brain, function in a similar manner. These networks use artificial neurons, which are interconnected nodes that exchange information. Instead of being explicitly told what characteristics make a dog, these networks learn by using training datasets, such as a catalog of dog images, which they use to infer what is and is not a dog. In order to make these networks more efficient, researchers have been studying exactly how our own brains are able to learn to classify objects — and how we learn to distinguish cats from dogs — in the hopes that the knowledge can be applied to artificial neural networks.
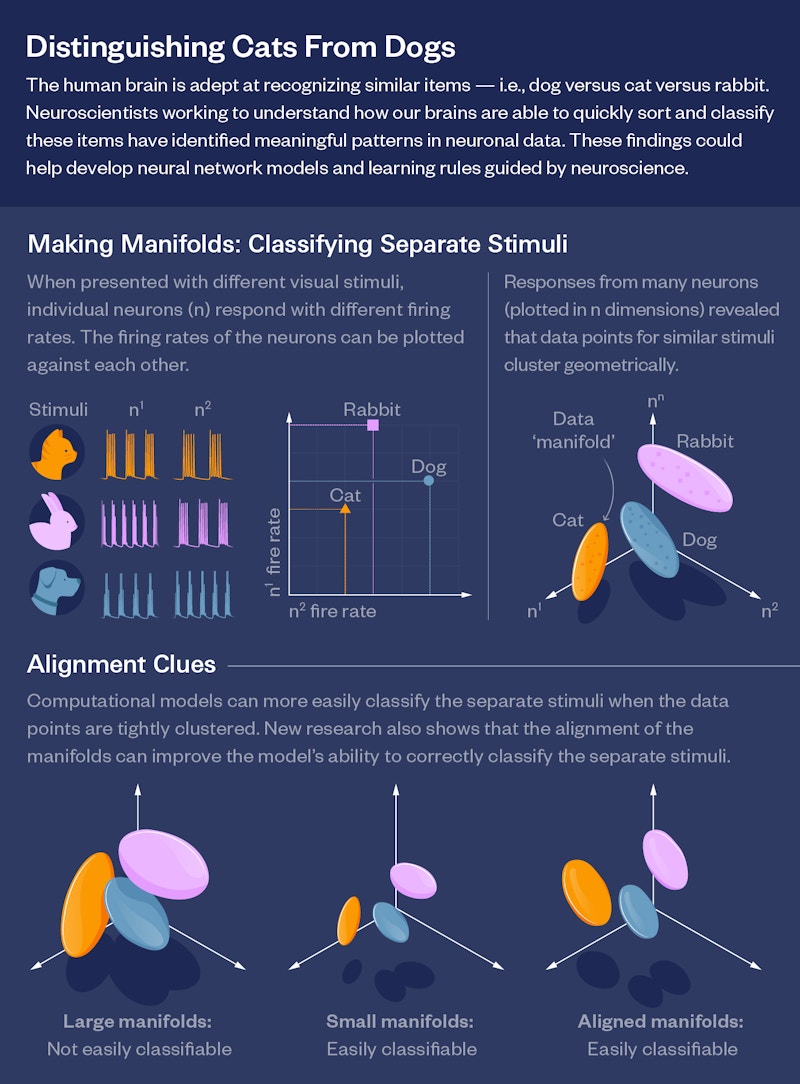
Understanding how we classify objects requires studying a large number of neurons in the brain, or artificial neurons within artificial neural networks, and is a significant challenge. In 2018, Chung developed a new theory on classification called the manifold capacity theory. To simplify the complex response of a population of neurons to classifying an object, the theory describes the geometry of the neural activities involved in the classification task. This geometry is known as a manifold, and every recognition task — such as identifying a dog or a cat — has a unique geometry, called a manifold representation. This theory captured the relationship among manifolds and how they determine the capacity of a network (how many things a network can classify). The findings showed the importance of manifold geometry when identifying images of, say, cats and dogs. When the manifolds were small, they were able to correctly identify more animals. At the time, the results were a huge step forward in describing how brains classify objects.
Real-world objects, however, exhibit intricate relationships and structures of similarity. In the same way that a species in the animal kingdom can be classified into subgroups like mammals, canines and domestic dogs, objects we see can be organized into a hierarchical structure based on their shared attributes. Chung and Wakhloo decided to take this organizational idea and integrate the interrelationships between attributes into Chung’s manifold capacity theory.
“By leveraging this theoretical framework we established in 2018, we aimed to shed light on the intricate relationship between neuronal correlations and their impact on the capacity of neural representations,” says Chung.
Whereas the previous theory was focused on identifying singular objects, their new work extends that to how objects are correlated and distinguished. To start, they worked out the mathematical description for the new theory.
What Chung and Wakhloo found was a connection between the network’s ability to distinguish between objects — such as a cat and a dog — and its ability to recognize similar traits, such as both animals having fur. These two aspects of recognition, often referred to as classification and disentanglement, are typically considered separate issues. The new findings show that they are correlated, and when the manifolds are organized well, they can improve classification performance.
“We’ve found there’s this nice duality; this relationship between small-scale and larger-scale structures,” Wakhloo says.
To understand duality, imagine you had a jar full of coins, where each coin represents a manifold. If the coins are haphazardly packed into the jar at odd angles, only a few coins can fit, and there is a lot of space between the coins. However, if the coins are neatly stacked, or if they are smaller in size, more coins can fit in the jar. The new paper, combined with 2018 findings, shows similar results for manifolds. Manifolds that are geometrically aligned improve a network’s ability to disentangle objects, and smaller manifolds help the network classify objects. The new theory mathematically describes this “stacking” and how the manifold alignment is important in recognition tasks, which adds to the previous theory of manifold size.
“In this new paper, we introduce a key enhancement to our previous theory by incorporating correlations between neuronal activities,” Chung says. “This crucial step brings us closer to unraveling the nature of neural population activities that underlie the complex organization of information, such as relationships between concepts and knowledge structures.”
With a functioning theory written down, Chung and Wakhloo applied the theory to data from a deep neural network designed for vision tasks. The theory was able to accurately predict the function of the network, which showed that the theory works.
The power of the new theory, according to the team, is that it can also be applied to real neural data to understand more fully how recognition works in the brain. Manifold alignment is important because it allows information in a network to be read out quickly and efficiently. Applying the findings to the brain could reveal insights into activity patterns or new properties. Additionally, the group is looking to apply their findings to training neural networks.
“The hope is that we can apply our theory to understand how neural networks are representing and manipulating the image and text data we feed them,” the team says. “It could help us understand how artificial intelligence systems function, which is an important step towards making them safer and more controllable.”