Schooling Fish May Offer Insights Into Networked Neurons
By clicking to watch this video, you agree to our privacy policy.
Say a group of friends is deciding where to go for dinner. A few people want Italian, a couple want sushi, but most have no preference. In an ideal democracy, the majority vote should win. But if the minority is highly vocal — two people really, really want sushi — they can disproportionately influence the group.
What role do the undecided diners play? You might predict they would simply slow the decision-making process. But research by Iain Couzin, director of the department of collective behavior at the Max Planck Institute for Ornithology in Konstanz, Germany, and collaborators suggests that undecided members of a group can have a profound effect on group behavior. Analyzing decision-making in schools of actual and model fish, researchers found that undecided fish democratize decision-making. These individuals tend to adopt their neighbors’ point of view, strengthening majority opinion and dampening the voice of the vocal minority. “It doesn’t matter how strong the minority voice is, the group will make a democratic consensus,” Couzin says. “These individuals play a very important role.”
Couzin studies the collective behavior of fish, birds, primates and other species, deciphering the rules that govern group behavior. His work has revealed how simple local rules can give rise to complex flocking patterns, such as the striking murmurations of starlings, when hundreds to thousands of birds swirl in a coordinated pattern. The research may have implications for neuroscience, perhaps providing a new perspective for analyzing how collections of neurons perform computations.
“It seems very likely to me that there will be some deep parallels between collective computation done by groups of organisms and by populations of neurons,” says Aaron Batista, a neuroscientist at the University of Pittsburgh. Batista and collaborator Byron Yu, an investigator with the Simons Collaboration on the Global Brain, are developing new ways of analyzing the behavior of populations of neurons. “We look for inspiration wherever we can get it,” Batista says. “There could be a lot in the collective ecology literature that we can borrow from.”
By clicking to watch this video, you agree to our privacy policy.
Couzin, who presented his work at the Cosyne meeting in Denver in March, treats groups of animals as a dynamic network. Rather than being physically connected, each member is linked to its neighbors via behavior. The movement of a fish in a school, for example, is determined by the movement of its nearest neighbors. “The types of systems we study are fluidlike brains, where individuals are moving relative to each other,” Couzin says. Neurons also form dynamic networks. Though anatomical connections among neurons are fairly static, functional connections change with learning and context.
Like fish schools, networks of neurons also contain undecided — or untuned — members, neurons that don’t seem to respond to a specific stimulus. This parallel particularly interested neuroscientists at Couzin’s Cosyne talk. Couzin and collaborators have shown that their findings can be broadly generalized, with relevance to physical spin systems and coupled oscillators, for example. “Because we’ve conducted models of this that extend beyond animal groups, we feel confident that this might extend to neural groups,” Couzin says. “Having a proportion of untuned neurons will cause the system to be extremely fast and accurate.” (The reasons for this lie in some complex mathematics. The process is reminiscent of stochastic resonance, in which a weak signal can be enhanced by the addition of white noise. The untuned neurons act somewhat like the “white noise” and help to convey the signal.)
“This example of the power of ignorance highlights the importance of thinking about the role of the individual versus the collective,” says Elad Schneidman, a neuroscientist at the Weizmann Institute of Science in Israel and an SCGB investigator who has collaborated with Couzin. “I think that struck a chord with neuroscientists.”
Couzin speculates that incorporating untuned cells into a network could make it more efficient. A network designed to detect direction of motion, for example, could consist entirely of neurons that respond to a specific direction. But that might be metabolically and evolutionarily costly. A looser system, in which some neurons aren’t direction-specific, might accomplish the same goal more efficiently.
“It’s inspirational from an analogy point of view,” says Brent Doiron, a neuroscientist at Pittsburgh and an SCGB investigator. “There is a lot of heterogeneity and a lack of specificity in the brain, in some sense just waiting to be coopted into a task.”
One challenge in neuroscience is that researchers often focus on cells with specific tuning — those that respond to a certain location in a maze, for example — and ignore untuned cells, making it difficult to understand their role in the network. But new technologies for recording from many cells simultaneously is making the study of untuned cells possible.
Batista says the findings could help inspire new experiments. “If we find an untuned neuron, we tend to ignore it because we don’t know what to make of it,” he says. “Iain’s work suggests we should be paying more attention to untuned neurons and looking for some contextual change that makes it tuned. That hadn’t occurred to us.”
Information flow
A key question both in groups of animals and in networked neurons is how information flows through the system. When a school of fish detects a potential predator, the school changes course to evade the threat. In reality, just a few members of the group actually spot the predator, and their alarm rapidly spreads across the group. Couzin studies this kind of information flow by using machine learning and computer vision techniques to infer hidden networks of interactions. “Then we can ask what makes an individual influential in the network,” he says.
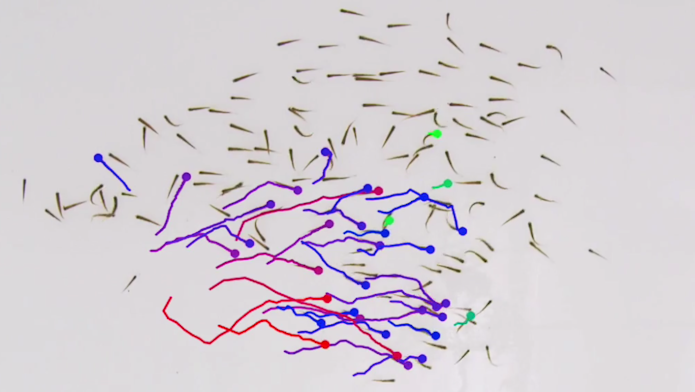
By clicking to watch this video, you agree to our privacy policy.
Schooling fish predominantly use visual information to make decisions. To understand how a startle response from a handful of fish propagates so efficiently, Couzin and collaborators developed software that tracks motion and estimates body posture. They then reconstruct what every individual in the school sees at each moment in time. With this information, they can determine which visual features startle an individual fish.
His group found a surprising relationship between network topology and flow of information. Epidemiological studies of networks suggest that the most influential — or infectious — individuals are those who are strongly connected to others and whose neighbors have diverse connections. In other words, you’re more likely to spread the flu if you have lots of friends and if your friends have an array of friends.
But Couzin’s group found that information flows through a network differently than a contagion does. “We found that social behavior is fundamentally different from disease-type processes,” he says. “The most socially influential individuals are connected strongly to a few individuals, who tend to have high cliquishness.”
Couzin says that this pattern emerges because individuals are seeking reinforcement from others. You’re more likely to believe a rumor if you hear it from multiple sources rather than just one person. “If someone has a different political view, you might spend all day with them and not change your mind, but you would catch the flu from them,” he says.
Networks of neurons may have a similar property. Growing evidence suggests that neurons act as coincidence detectors, meaning that cells don’t respond to an initial stimulus but wait for a second event. This minimizes the probability of responding to false alarms.
Of course, there are obvious differences between schooling fish and neural circuits, so it’s unclear whether the tools Couzin and collaborators have developed to study information flow in animal groups would work for networks of neurons. The neural networks that form the brain are much more complex than networks of schooling fish. Fish schools have many fewer members than neural networks, which might be made up of thousands of cells. And the brain has a diverse array of neurons, including inhibitory and excitatory cells. “I have a break of symmetry in the system I study that he doesn’t have to deal with,” Doiron says.
Moreover, the rules that govern a school’s dynamics — the closer two fish are, the stronger their interaction — are much simpler than those governing neural plasticity. Synaptic changes are influenced by a host of molecular and biological forces, which neuroscientists are still trying to understand.
Couzin also has more complete information about the group he’s studying. He can track every member of the school, whereas neuroscientists have access to a small fraction of the neurons in the larger network. At this point, researchers can’t reconstruct the information that neurons use to make decisions. Batista notes that this is changing, as researchers develop new tools for looking at both anatomical connectivity and activity simultaneously. “I think we are getting to the point where we can see if neurons operate more like an epidemic or a school of fish,” he says.