
Why Neurophysiology Needs an MP3
Part 1 in the special report “The Data-Sharing Problem in Neuroscience”.
Kelson Shilling-Scrivo arrived at the Janelia Research Campus, in Reston, Virginia, last spring with almost 100 gigabytes of neuroscience data in tow — reams of raw and processed calcium imaging data recorded from the auditory cortex in mice. Shilling-Scrivo, a graduate student at the University of Maryland, had signed up to join a group of 40 neuroscientists and developers at a hackathon for Neurodata Without Borders (NWB), a new platform for standardizing how neuroscience data are stored.
Shilling-Scrivo’s lab already had a system for collecting, storing and analyzing data. But like many labs, it was a hodgepodge; as each user built on top of that system, it became more difficult to work with other lab members’ data. Shilling-Scrivo hoped the NWB platform would make it easier to share data among both his own lab members and collaborator labs. “The NWB format got me excited, because it enables data sharing right now,” he says.
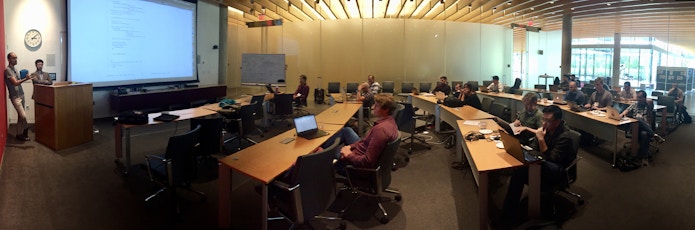
NWB is one attempt to address an enormous problem in systems neuroscience. New technologies for recording neural activity are generating huge volumes of data, which are rapidly becoming too large for any one lab to make sense of. But as datasets get larger and richer, they also become more difficult to share, analyze and store. “Deciphering other people’s data can be so hard, it’s easier to collect new data,” says Ben Dichter, a data scientist and NWB’s community liaison. “I think that’s a travesty.”
The potential benefits of a widespread data-sharing infrastructure are enormous. The MP3, a standardized format for encoding audio files, transformed the music industry. The $100 million Sloan Digital Sky Survey, which created 3D maps of the universe, reshaped astronomy. “Before that, you had to make a reservation at a telescope, wait six months, hope it’s not cloudy, get data, store it and study it,” says Joshua Vogelstein, a neuroscientist at Johns Hopkins University. “After the survey, scientists could look up data relevant to their hypothesis” before trying to confirm it, dramatically accelerating the pace of research.
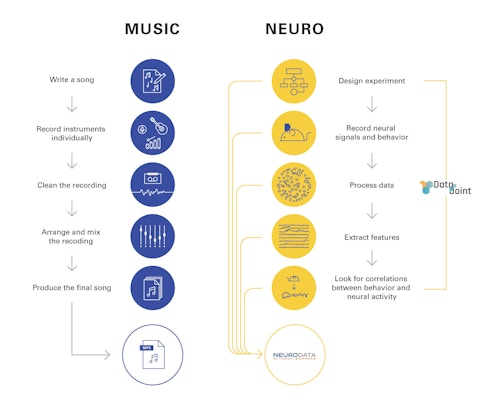
Neuroscience, particularly neurophysiology, has lagged behind other fields in developing an infrastructure for easily sharing data. Unlike astronomy or genomics, where data are collected with centralized or standardized commercial tools, both the hardware and software used to collect and analyze neurophysiology data are often customized to individual labs. Graduate students and postdocs develop their own idiosyncratic methods for storing and processing data, which then lie siloed in individual labs. “In electrophysiology, everyone builds their own scope and writes their own code,” says Lydia Ng, senior director of technology at the Allen Institute in Seattle.
These datasets likely harbor much richer information than can be gleaned by any individual scientist, but the effort required to access and analyze another group’s data is prohibitive. “In some sense, the way we share data now is an anachronism,” says Karel Svoboda a neuroscientist at Janelia Research Campus and an investigator with the Simons Collaboration on the Global Brain (SCGB). “We do studies that last five to six years, involve terabytes of data and very complex multidimensional datasets, including neurophysiology, imaging and behavior, and then we shoehorn these datasets into a research paper that represents only a highly biased small feature of the data.” Instead, Svoboda says, scientists should focus their efforts on sharing data, resources, protocols and software. “The paper should essentially be an advertisement of the data,” he says.
“An inability to share data effectively has held the field back,” says Anne Churchland, a neuroscientist at Cold Spring Harbor Laboratory and an SCGB investigator. “The infrastructure for large-scale sharing hasn’t existed.”
The Right Incentives
The now ubiquitous MP3 wasn’t always the dominant format for audio files. The 1990s saw a number of formats, including .au, .wav. and .snd. MP3’s rise to prominence was driven largely by the presence of Napster, a music sharing site that made huge volumes of music available in the MP3 format. “Before Napster, we had all these different file formats,” says Kenneth Harris, a neuroscientist at University College London and an investigator with the SCGB. “Within a year or two, everything collapsed onto MP3s, because there was a large corpus of available data.”
Astronomy and genomics both had their own Napster-like events — the Human Genome Project and the Sloan Digital Sky Survey. “Once the Human Genome Project became shared, everyone built tools to work on it,” Vogelstein says. “If you got new data, you would of course store it in format that tools already work on.” Harris, Vogelstein and others say that neuroscience needs an equivalent incentive, a huge and expensive shared dataset. “I think progress in neuroscience has been slow because we haven’t had large-scale projects to drive standardization,” says Harris, who has been involved in efforts to standardize neuroscience data for the last 20 years.
But that’s changing. The National Institutes of Health, the SCGB and other funders are increasingly supporting large-scale collaborations with data-sharing requirements baked in. Intensive data-sharing is a central component of the International Brain Lab, a project spanning 21 labs in multiple countries that will compile data from different groups to better understand the brain-wide process of decision-making. IBL researchers have developed methods for sharing data among its member labs, and the project as a whole has committed to publicly release data within a year of collection or when accepted for publication, whichever comes first. The MICRONS project, an effort to map part of the mouse cortex, will release large amounts of calcium imaging and connectivity data. Vogelstein thinks it will take datasets like these — which consider data-sharing from the get-go — to encourage the field to coalesce around a set of standards and develop tools for working with these resources. “To make something like that happen, you need test cases,” says Loren Frank, a neuroscientist at the University of California, San Francisco, and an investigator with the SCGB. “You need groups for whom it’s relevant and for whom it’s required to make their project work.”
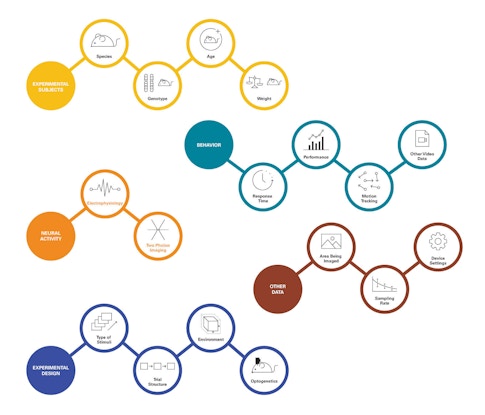
A major challenge in developing new tools for sharing and standardization is the diversity of neuroscience data, which span a much greater range of properties than audio files. Neuroscientists can collect hundreds of types of data, such as where in the brain activity was recorded, the type of electrodes or sensors that were used, and what the animal was doing during the experiment. Data types are also constantly changing, as scientists develop new tools for tracking and manipulating the brain. Characterizing behavior is particularly challenging. “There isn’t any way to standardize the description of a behavioral task,” Harris says.
A Test Case in Data-Sharing
The IBL, launched in 2017 and co-funded by the SCGB and the Wellcome Trust, has had to tackle this challenge head-on. The project aims to better understand how the brain makes decisions, a computation that is distributed across the brain. To do that, collaborators are running the same decision-making task across multiple labs, all recording from different parts of the brain. They’ll then synthesize data from different groups, which requires a standardized way to collect, store and share data. One of the biggest difficulties in accomplishing this has been organizing the meta-data that accompanies each experiment. “The time of day each experiment was conducted, the age, weight, sex and genotype of each experimental subject and the time since they last ate: all these things might make a difference,” Harris says. “It’s not even a computing challenge, it’s a social engineering issue, getting that information from each institution’s animal facility.”
The IBL developed a computerized colony management system now in use by its member labs that collects metadata and uploads this information to IBL servers. To more easily access and search data among member labs, researchers developed a tool called Open Neurophysiology Environment, inspired by the Open Microscopy Environment, a consortium of public and private labs creating open source software and standards for microscopy data. It lacks the comprehensiveness of NWB but is simpler to learn and allows random access, Harris says. “It is a way for a user to search for experiments they are interested in, and download specific data items from these experiments straight into computer memory,” Harris says. They eventually plan to make IBL data available to the broader community via NWB.
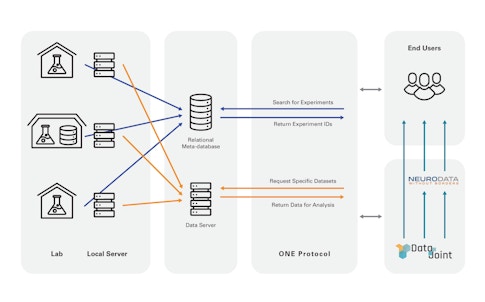
They are also developing ways to process the reams of data these experiments produce — terabyte-scale records of neural activity, behavior and other factors — so that data can be more easily shared. This is especially important because half of the IBL’s PIs are theorists and computational neuroscientists, who need ready access to the data. Researchers hope that some of the tools and infrastructure they develop for the IBL will eventually be useful to the community more broadly, perhaps providing a model for how to work closely with many labs. (Information on tools the IBL has developed is available here.)
A number of large collaborative projects are now emerging through the NIH BRAIN (Brain Research through Advancing Innovative Neurotechnologies) Initiative–funded U19 grant program, which brings together teams with different expertise and approaches, working at different scales and even in different species to tackle the same question. Each U19 includes a proposed data science plan outlining how the team will standardize data. “It’s sort of a sandbox approach to get them to try out different things — we want the science to drive data sharing,” says Grace Peng, director of mathematical modeling, simulation and analysis at the National Institute of Biomedical Imaging and Bioengineering. “We really need the community to buy into it and let them take charge of how they want to do data-sharing.”
NIH is also taking a more top-down approach by funding development of data archives where awardees can put their data, as well as software to integrate and analyze data. Part of the value of getting the U19 data sharing groups together, Peng says, is to help determine if another resource, such as a different type of archive, is needed. The government-funded BRAIN Initiative put forth new data sharing requirements in January, requiring future awardees to deposit their data in BRAIN data archives, and to include data-sharing plans and costs in grant applications. The new requirements just went into effect, so it’s not clear yet how well they will work or what kind of onus they put on PIs.
Many of the challenges in data-sharing are technological. But the field’s culture and sociology present their own hurdles, which are especially apparent to those coming from outside the field. “Neuroscience has an interesting tic, people who do data collection feel they own the data, even when it’s generated under publicly funded grants,” says Ariel Rokem, a data scientist at the University of Washington who is developing new data-sharing tools for a U19 project. “Creating valuable large datasets and making it available is not currently rewarded for reasons I don’t entirely understand.”
This is in stark contrast to other fields, such as genomics. John Marioni, a computational biologist at the European Molecular Biology Institute, who serves on the scientific advisory board for the International Brain Lab, says neuroscience resembles genetics 20 years ago. “As an outsider, I was surprised: In genomics, data have to be public, to be stored in an open-access or managed-access repository and available to the wider scientific community,” he says. Data sharing in genomics is so entrenched that “the thought of not publishing your data is fairly staggering,” Marioni says. “Journals wouldn’t accept your manuscript. My peers wouldn’t accept me.” In some neuroscience communities, in contrast, “it seems like publishing data is the exception rather than the rule.”
In genomics, the human genome project and the efforts that followed — such as the 1,000 Genomes Project, an effort to better catalogue human genetic diversity — were instrumental in getting the field to adopt specific standards. “It was an incredibly important byproduct of that project,” Marioni says. “The people who led it deserve enormous credit for that foresight, that the data itself isn’t just important but how to share it.” Others in the field adopted those formats and developed tools around them because they wanted to be able interact with the reference data. For Marioni, it would be inconceivable to ask lab members to develop their own storage systems, something routinely done in neuroscience.
Fortunately, the sociology of data sharing in neuroscience is starting to change, driven by both necessity and the recognition that sharing data will help the field as a whole. “Datasets are getting so big and rich — within my single lab, we couldn’t reap all the benefits of the dataset in 20 years,” says Beth Buffalo, a neuroscientist at the University of Washington, in Seattle, and an SCGB investigator, who is leading a U19 project. “Making more use of the dataset that was expensive and time-consuming to gather is the right thing to do.” (Find more on how Buffalo and her U19 collaborators approach data sharing in Part 4: Standardizing Data Across Species: A Case Study)
Part 2: Driven by the growing number of large-scale collaborations, researchers are developing new ways to standardize both data and analysis pipelines.