
Standardizing Data Across Species: A Case Study
Part 4 in the special report “The Data-Sharing Problem in Neuroscience.” Part 1 lays out the problems; part 2 describes some solutions; part 3 is a case study of a successful multi-lab meta-analysis.
Beth Buffalo and collaborators at the University of Washington in Seattle are part of a multi-institute collaboration, part of the NIH’s U19 program, that aims to better understand rapid learning. The project brings together researchers studying both non-human primates and humans, and researchers want to directly compare brain activity in both species. “As they learn a task, do we see similar patterns of brain activity evolving in primates and humans, both within regions and across regions?” asks Ariel Rokem, a data scientist working on the project.
Buffalo says figuring out how to best share data within their U19 collaboration, even before publication and public sharing, has been challenging. Datasets have grown so large — her lab generates about 15 terabytes of data a month — that local computing is no longer feasible. “It’s really about getting data in the cloud and bringing the computation to the cloud,” she says. That requires a different approach to formatting data and writing code to analyze it.
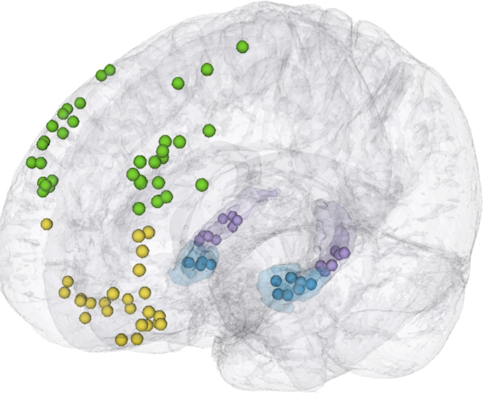
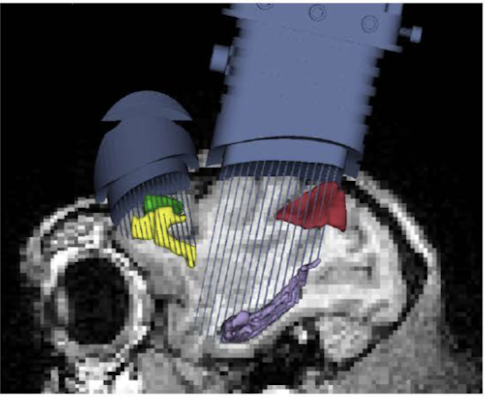
Rokem is building a system inspired by a geosciences initiative called Pangeo, a project funded by NASA and NSF to build systems to analyze datasets that are too big to move. Pangeo and many of the data-sharing tools emerging in neuroscience are open source, which Rokem says is critical for building long-lasting and robust systems. “Lines of code are not as important as the intellectual infrastructure, the collaborative networks of people who write and maintain the code,” Rokem says.
A central part of the project will be running the same types of analyses on data collected using different recording methods in different species. For this issue, Rokem and collaborators took inspiration from a neuroimaging project called the Brain Imaging Data Structure (BIDS). Essentially, collaborators agree on how to save and name data files so that data can be easily shared and analyzed the same way. “We are slowly defining a data specification of how files are organized and named and what metadata is stored,” Rokem says. “That makes eventual sharing of data in larger community easier.”
One of the biggest challenges Buffalo, Rokem and others have come up against when making data public is social. Electrophysiology data are hard won — it can take years to collect and analyze, particularly in primates — and researchers want to maximize their publications from that data. “When is the right time to share data within a collaboration, so that individuals don’t feel like they are competing with their collaborators?” Rokem says. “The social structure impedes sharing of data, even among collaborators.” The solution, he says, is to communicate extensively and to be very explicit.
Buffalo and collaborators also have to figure out who supports data storage once the grant cycle is over. Data collection is outpacing their expectations, and storage costs will soon surpass what they had budgeted in their grant. “Where is data going to sit, who will pay for storage in a way that’s accessible — the more accessible the type of storage, the more expensive,” Buffalo says.
Despite these challenges, early efforts at cross species analyses have been successful. In June, trainees from different U19 labs got together for a ‘data science sprint.’ “They started coding together and made decisions about what analyses to do across the cloud,” Buffalo says. “I’m confident this kind of story will come, that’s the whole point of the U19.”
How BrainCogs Learned to Share: A Case Study
Members of the multi-lab BrainCogs collaboration are using DataJoint to more easily access each other’s data.