
Tracking Information Across the Brain
As you watch a ballet dancer pirouette across the stage, your visual system rapidly parses and integrates the cascade of sensory information that makes up the scene — the color and texture of the dancer’s costume, the arched shape of her leg, the rotation of her spin — to generate a cohesive percept of her elegant twirls.
For most of neuroscience’s history, researchers have been limited to studying isolated aspects of this process, such as how the brain parses color or movement. But we know very little about a vital component: how different regions coordinate information. “The classic systems-neuroscience approach is to focus on one neuron in a particular brain region, but we know it’s really circuits that produce the dynamics that underlie cognition,” says Karel Svoboda, a neuroscientist at Janelia Research Campus and an investigator with the Simons Collaboration on the Global Brain (SCGB). “It’s not a neuron, not a brain region — it’s a connected set of neurons in brain regions that do the computation.”
Neuroscientists finally have the hardware to examine the volley of information across different regions: new probes and high-resolution imaging technologies capable of simultaneously recording electrical signals from multiple populations of neurons across the brain at the single-cell level. But with this power comes a major challenge. Parsing the code of tens or hundreds of cells in a single population is already a complex endeavor. Multiply that by 2 or 3 or 10 distinct but interacting populations, and you’ve got an epic computational problem.
Neuroscientists are now thinking about how to best tackle the datasets generated by these new technologies. “In terms of looking at the resolution of individual neurons and spike trains, we are only getting started,” says Byron Yu, a neuroscientist at Carnegie Mellon University and an investigator with the SCGB. “What are the statistical measures we have to try to analyze those data? What are new experiments to design to try to tease apart interactions among brain areas even more incisively than we are able to today?”
Tracking sharp wave ripples
Loren Frank, a neuroscientist at the University of California, San Francisco and an SCGB investigator, has long been interested in how the brain uses memories stored in the hippocampus to make decisions. When choosing where to eat dinner, for example, you might recall the last time you ate at your favorite restaurant, what you ate and where it’s located in relation to where you are. “Retrieving and evaluating memories involves complicated dynamics across brain areas that we don’t understand,” Frank explains. “To use them, we need to turn them back on and do something with them.”
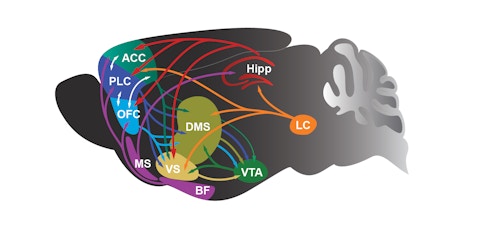
Frank’s team looks at how specific patterns of hippocampal activity propagate to other brain areas. They focus on a pattern called sharp-wave ripples, which appear to be an expression of memory in the brain. Sharp-wave ripples look like a sped-up version of activity that occurred during a specific event, such as when an animal is navigating a specific part of a maze.
To make decisions, the brain has to integrate memory information in the ripple with other data distributed across different systems. “When we see these events, what is going on in rest of brain?” Frank asks. “How do they go out and engage other parts of the brain?” Sharp-wave ripples’ punctate rather than continuous nature make them a good candidate for tracking across the brain; scientists can analyze neural activity in other regions during the precise time interval of each ripple. “With sharp-wave ripples, you can look at what’s going on around them,” Frank says.
“From a methodological standpoint, it’s hard to know how information flows in the brain by looking at connections,” Frank says. “We take advantage of a real event that we know is important and use it to define different networks and different patterns of information flow.”
Preliminary research suggests that sharp-wave ripples have a widespread effect on brain activity. “Everywhere we look in the brain, neurons outside the hippocampus can change what they are doing at the time of these sharp-wave ripple events.” For example, during a hippocampal sharp-wave ripple, ongoing activity in the prefrontal cortex is suppressed and replaced by a new pattern that reflects what was happening in the prefrontal cortex during the original event. “This lasts for a few hundred milliseconds, then switches back,” Frank says.
In research published in Neuron in December 2019, Frank’s team looked at how the dorsal and ventral hippocampus, which have different anatomical connections, route information. The team were surprised to find that sharp-wave ripples originating from dorsal and ventral regions seem to engage distinct groups of neurons in the nucleus accumbens, and did so at different times. “It looked like they are engaging two opposing networks,” Frank says.
Untangling feedforward and feedback connections
A major challenge in studying how brain areas communicate lies in the tangle of recurrent neural connections. It’s not always clear what regions are connected and in what direction information flows; in many cases, multiple areas interact via both feedforward and feedback connections. “Any two areas can be talking in both directions at the same time, so how do we know who is talking to whom and what are they saying?” Yu says.
Yu and collaborators are developing new statistical approaches to dissect this cacophony. Research published in Neuron last year in collaboration with Adam Kohn and Christian Machens, both SCGB PIs, and collaborators outlined a method to determine when population signals remain local or are shared between areas. The researchers adapted an existing statistical method, reduced rank regression, to examine when fluctuations in activity in one region are shared with another. They found that brain regions share select information through communication subspaces. “The findings imply that only a subset of information from V1 is transmitted downstream,” Yu says. “This suggests that a subspace could be a mechanism for routing information to different places.” (For more on this, see “Brain Areas May Use ‘Subspace’ Communication to Talk to One Another.”)
Yu and collaborators are now delving deeper into signals that are relayed among areas, developing new methods to more incisively tease apart feedforward and feedback interactions. That can be particularly challenging because “communication is bidirectional, asymmetric and likely occurring at multiple time delays,” said Evren Gokcen, a graduate student in Yu’s lab, presenting the work at the Computational and Systems Neuroscience (Cosyne) conference in Denver in February 2020.
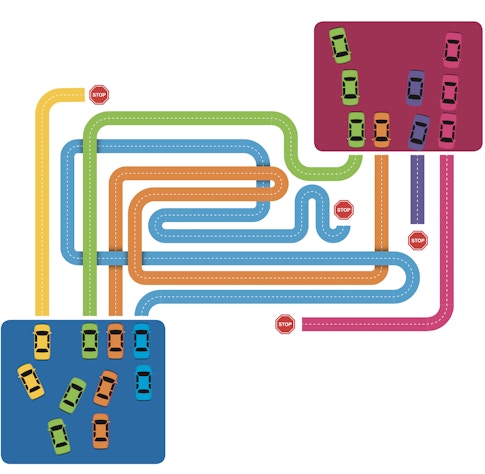
To tease apart those exchanges, the researchers examine how interactions among regions vary with different time delays, which act as a proxy for feedforward or feedback interactions. If A talks to B, the information A relays to B will appear after a delay, Yu says. “We are trying to leverage the idea of a time delay to try to understand these interactions.”
They developed a new approach called DLAG (Delayed Latents Across Groups) that adapts dimensionality reduction for multiple areas. When doing dimensionality reduction in a single population, each latent variable represents a prominent activity pattern shared among neurons. The new method uses the same idea, except each latent variable represents a pattern of activity in area A and a pattern in area B that occur together with some time delay — for example, if a certain pattern in area B always follows 50 milliseconds after a pattern in A. “We can then assign some notion of directionality to these across area signals,” Gokcen said at the conference.
Researchers applied the method to interaction between two areas in the visual system, V1 and V2, with strong reciprocal connections. Preliminary research presented at the conference suggests that feedforward interactions between V1 and V2 tend to carry more stimulus-driven signals than do feedback connections; for a sinusoidal grating stimulus, feedforward interactions from V1 to V2 look sinusoidal but feedback interactions do not. “All of this fits with intuition, but no one has been able to pull this out of the data in an unsupervised way,” Yu says. The method can be applied to any distinct populations and recording types.
Yu and collaborators are also examining how interactions among brain areas change with behavior, such as when animals either perform accurately or make mistakes on a perceptual decision-making task. Do mistakes occur because of a breakdown in communication between areas?
Internal state and communication
Nick Steinmetz, a neuroscientist at the University of Washington, in Seattle, and an SCGB PI, and collaborators are taking a similar approach, using canonical correlation analysis to characterize the communication structure across two multidimensional populations to examine how related those populations are. Researchers look at the structure of population activity in different brain areas tied to a specific event, such as when a mouse sees a visual stimulus or presses a lever. (This approach has some of the same advantages as looking at activity tied to sharp-wave ripples.) To analyze the data, Steinmetz and collaborators use a multidimensional generalization of an existing method for looking at two neurons, Joint Peri Stimulus Time Histogram (JPSTH), joint distribution of activity of two neurons over time tied to an event.
“Going forward, we will be able to answer all kinds of questions about how dimensionality changes as a function of time, internal state and behavioral condition,” Steinmetz says. “I think we will start to understand more about the nature of that communication. When you start thinking about communication not just as a single number correlation between A and B but as a multidimensional structure, you can look at all different kinds of changes,” he says, “such as changes in the size of the communication space or changes in rotation or dimensionality.”
Steinmetz is particularly interested in examining how correlation structure changes as a function of internal states, such as whether an animal is engaged or not. For example, Steinmetz and collaborators have found that dimensionality of the correlation structure changes based on engagement. “You see a shift in state in the cortex, thalamus and striatum, from desynchronized in the aroused or alert state to synchronized in the disengaged state,” Steinmetz says. “In the unaroused state, you see huge correlated fluctuations.”
His team is now applying this approach to data collected as part of the International Brain Laboratory (IBL), a large-scale collaboration among 21 labs that seeks to decipher how decisions are made across the brain. “With IBL data, we will be able to look at how the internal state is modifying connectivity among brain regions,” Steinmetz says. “I think that’s an exciting question because it’s different than how people looked at internal state in the past.” Previous efforts focused on how activity of individual neurons or local circuits change with the internal state, rather than how communication among brain regions changes.
When to share?
Yu’s work shows that individual brain regions sometimes routes information to other areas and sometimes keeps it private. How do neural circuits mediate that gating process? “Given that almost everything in the brain is connected to everything else, how do brain regions connect and disconnect?” Svoboda asks. “What are the mechanisms, and how do they enable flexible computation?”
Svoboda and collaborators are trying to determine when and how information flow is shut off. They focus on short-term memory, which can become resistant to further sensory information. If you’re holding someone’s phone number in your short-term memory, for example, you can’t remember another one.
In research presented at Cosyne, scientists trained animals to respond to an optogenetic stimulus delivered to the somatosensory cortex — licking the right side after a delay when they detected the stimulus or the left when the stimulus was absent. Because of the delay component, the task requires short-term memory. The researchers recorded activity in the somatosensory cortex and anterior lateral motor (ALM) cortex, which is involved in decision-making. Choice-related neural activity was concentrated in the ALM and increased during the delay.
To understand the flow of sensory information during motor planning, researchers gave mice distractor stimuli — optogenetic stimulation delivered during the delay that mimicked the target stimulation but indicated the opposite choice. The distractors, which caused the animal to change its choice, worked best if given early in the delay, and had little effect if delivered late in the delay period. That suggests that choice-related neural activity in the ALM is resistant to interference in a time-dependent way.
One possible explanation for this time-dependency would be a sort of gate closing during the delay period, blocking distractor information from reaching the ALM. But that wasn’t the case. Researchers found that distractor information does indeed reach the ALM late in the delay period, but decision-related activity in the ALM becomes more resistant to interference over time.
Connecting multiple recurrent networks
How does the flow of information change based on an animal’s state of mind? Kanaka Rajan, a neuroscientist at the Icahn School of Medicine at Mount Sinai, in New York, and collaborators are exploring this question by studying frustrated fish. The animals are given a mild electric shock that they can’t avoid by swimming away. Recognizing that their efforts are futile, the fish eventually give up, a state known as learned passivity. Scientists want to know what triggers the behavioral change from active to passive.
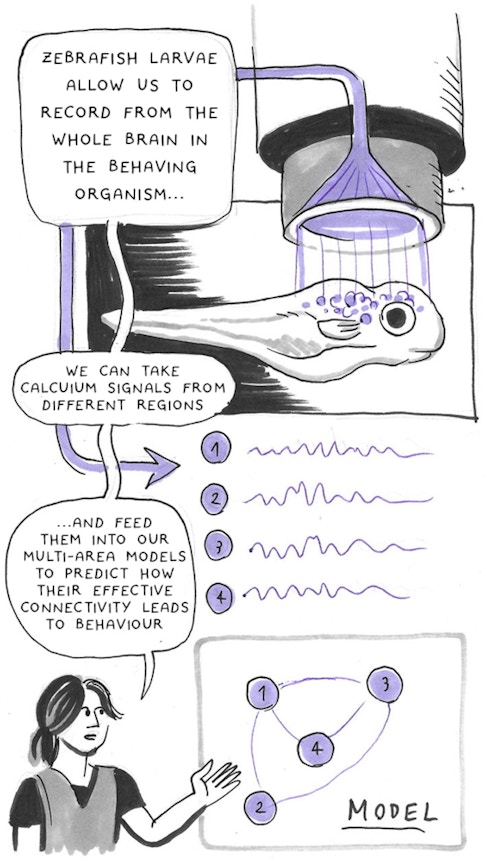
Transparent zebra fish larvae offer a clear advantage when studying communication among brain regions: Scientists can capture calcium imaging of the whole brain simultaneously as the animals swim. Rajan’s team is collaborating with Karl Deisseroth’s lab at Stanford, analyzing calcium imaging data recorded from 10,000 to 40,000 neurons across the entire brain in swimming fish.
To better understand the neural activity changes that occur as animals learn to give up, Rajan and collaborators create a collection of connected recurrent neural networks (RNNs), with each network representing an individual brain area. Each network is trained to mimic neural activity recorded from an individual population and contains as many units as there are neurons in the population. Because networks are trained when connected to other networks, they reveal information about how information flows among regions. “By constraining the RNNs to match the entire neural population’s dynamics simultaneously, we can account for common inputs, and assign both magnitude and directionality to all the interactions responsible for the observed dynamics,” Rajan says.
Researchers then dissect the network of networks to analyze how connectivity both within and between networks changes as animals learn to give up. “That can tell us how brain-wide interactions change from one condition to another or through experiential state,” Rajan says.
In research published in Cell in 2019, Rajan, Deisseroth and collaborators showed that passive coping is tied to progressive activation of neurons in a brain area called the habenula, with an accompanying decrease in activity in downstream neurons in a region called the raphe nucleus. RNNs modeled on these two areas show that as the animal stops moving in response to inescapable adversity, habenular interactions strengthen, boosting currents within the habenula. Currents flowing from the raphe to the habenula also strengthen during this period, driven by brain-wide plasticity mechanisms that alter interactions across the habenula and raphe. The findings hold in multiregion networks built from different fish.
Researchers are now scaling up their approach to include additional regions, including the telencephalon and thalamus. Rajan says the data-inspired RNN approach provides an advantage over methods based on correlations, which are difficult to scale beyond two regions. “They are unable to assign directionality to the interactions uncovered and cannot account for common inputs driving both regions simultaneously,” she says. “Studying interactions between brain regions becomes problematic because you don’t know if covariation is because of connections, common inputs or information exchange.”