Brain Areas May Use “Subspace” Communication to Talk to One Another
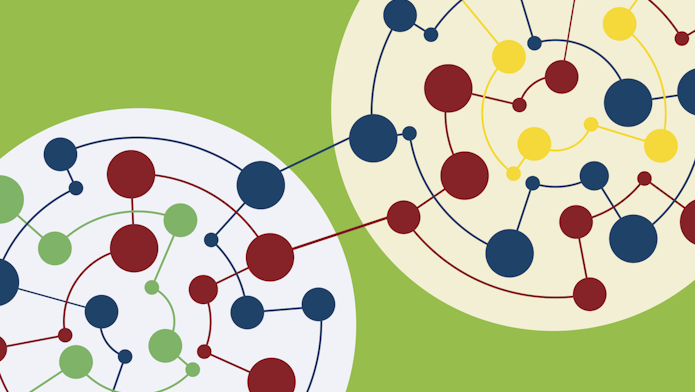
If you want to understand air traffic patterns around the country, but you only focus on flights coming into and leaving Kennedy or O’Hare airport, you’ll end up with a limited picture. Yet that’s exactly what much of modern neuroscience does. New technologies have enabled researchers to analyze activity in hundreds of neurons simultaneously, opening a window into the computational language of the brain. But to date, most studies focus on how single brain regions function rather than on how different parts of the brain work together. This approach sidesteps a central question in the field — “Why do we have different populations of neurons and what transformations are going on from one brain area to another?” says Marlene Cohen, a neuroscientist at the University of Pittsburgh and an investigator with the Simons Collaboration on the Global Brain.
Over the last five years, scientists have begun using the new technologies to study multiple brain areas, opening a new set of questions. “How do different parts of the brain communicate with each other, and how does that change moment-to-moment based on what we’re trying to accomplish?” asks Adam Kohn, a neuroscientist at Albert Einstein College of Medicine and an SCGB investigator. Visual information, for example, passes through different parts of the cortex. But we know very little about how all the steps come together to produce a cohesive visual perception of the world.
In a new study, published in Neuron in April, Kohn and collaborators lay out an approach for analyzing neural activity in groups of neurons in connected brain regions. They found that different areas seem to exchange information by means of computationally defined channels, dubbed communication subspaces, which act like sieves, permitting only some data to pass from one area to another. “Signals can be shared downstream or kept private,” Kohn says. “For me, this opened up a new way of thinking about the area to area communication problem.”
The new research differs from previous efforts in both resolution and scale. Older studies examining how brain regions interact have employed coarse-grained methods, such as fMRI, which indirectly monitors activity in large swaths of brain tissue. Studies at the resolution of single cells have focused on small numbers of cells, looking at how activity of pairs of neurons inhabiting two different regions fluctuate with respect to each other, for example.
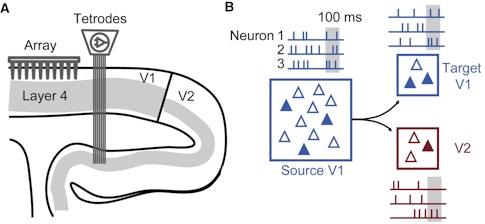
A Communication Channel
The new study focused on two visual areas, V1 and V2, known from anatomical analysis to be highly connected. To understand how population activity in V1 influences V2, João Semedo, a postdoctoral researcher co-advised by Kohn and SCGB investigators Byron Yu at Carnegie Mellon University, and Christian Machens at Champalimaud Foundation, took advantage of trial-to-trial fluctuations, variations in patterns of neural activity that occur even though the animal is looking at the same image over and over again. “We asked if V2 gets to see all the activity fluctuations in V1 or if it only gets to see some,” says Yu, who co-led the study with Kohn and Machens.
Few well-established methods exist for studying multiple populations of neurons in different parts of the brain, so the researchers’ first step was to develop some. “We needed to figure out the right conceptual framework and the right analysis methods to look at these data,” Yu says.
The team used a general approach called dimensionality reduction, in which the activity of each neuron in a population is first mapped in a multidimensional space. Interpreting the resulting high-dimensional structure is difficult, so scientists use computational methods to search for a lower-dimensional structure that represents the data’s most prominent components. These components are thought to represent certain aspects of the population’s computational function.
In the new study, researchers used a variation of dimensionality reduction called reduced-rank regression, a statistical method for estimating the relationships among multiple variables. Using this approach, they searched for a low-dimensional space within the higher-dimensional V1 activity space that best predicts activity in V2.
“Using dimensionality reduction to understand something about the complex patterns of interactions is a creative and clear-eyed way of getting a handle on what looks like very complicated patterns of activity,” says Cohen, who was not directly involved in the study but is now collaborating with the authors. “You can use these methods to understand variability among two groups and then start to understand the nature of cognitive, sensory or behavioral information shared among any two areas.”
The analysis revealed that such a low-dimensional space indeed exists. The researchers dubbed this a communication subspace — the specific subspace of activity in V1 that is important for activity in V2. V1 activity within the subspace is correlated with activity in V2, whereas V1 activity outside the subspace is not. No such subspace exists for filtering communication between different populations within V1 — groups of neurons within the same area share all information with each other — suggesting that this isn’t a ubiquitous property of groups of neurons or an artifact of the analysis methods. “This is the most direct path I’ve seen from neurons to actual neural computation,” Cohen says.
The findings imply that only a subset of information from V1 is transmitted downstream. This suggests that a subspace could be a mechanism for routing information to different places. Brain regions that contain several types of information, such as the color of an object and its movement, may need to send the color information to one part of the brain and the movement information to another. “One possible way to do that is to have a different communication subspace for each downstream area,” Yu says. “Only the appropriate patterns make it to the other area.”
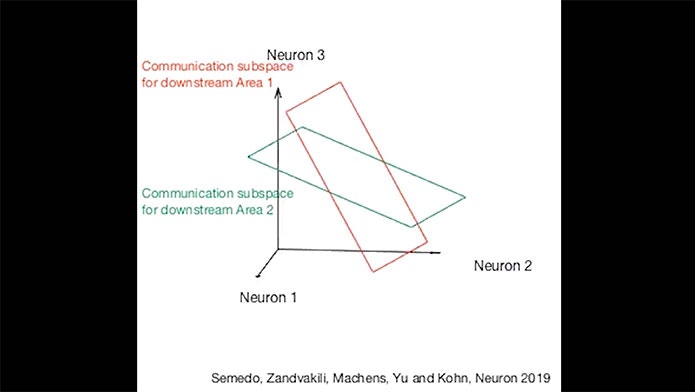
By clicking to watch this video, you agree to our privacy policy.
Communication subspaces appear to be selective — the most dominant patterns of activity in V1 are not necessarily the ones that drive activity in V2, for example. Nor does a specific subset of neurons in V1 drive V2. “You can have a communication subspace regardless of whether all neurons project between areas,” Yu says. “The idea of the communication subspace is all about how the weights of the connections are configured.”
Though it’s unclear exactly how the brain generates such subspaces, Yu points out that the setup is fairly straightforward from a mathematical perspective. “The activity of a neuron in the downstream area is a weighted linear combination of the activity of neurons in the upstream area,” he says. “If those weights are configured in a consistent way across all downstream neurons, you can obtain this communication subspace.”
Dynamic Communications
The new findings emerged from Kohn and Yu’s original SCGB project, “Corticocortical Signaling Between Populations of Neurons”, and formed the inspiration for their new project, “Communication between neural populations: circuits, coding, and behavior”. An expanded team, including Cohen, Machens, Brent Doiron, Ken Miller and Alex Pouget, is exploring how different tasks alter the communication subspaces, whether communication subspaces provide a way to dynamically modulate communication among different areas, and whether feedforward and feedback connections involve similar activity patterns.
Previous work has shown that patterns of population activity are malleable. “If you attend, learn or adapt, all of those processes are evident in the relative activity among neurons,” Kohn says. Cohen has shown, for example, that paying attention alters shared variability — how often neurons fire together in response to the same visual stimulus — suggesting that attention modulates coupling between two areas. Researchers can further test this idea by looking at whether neural activity is better aligned with a communication subspace when an animal is paying attention.
“Once you have a subspace, you can couple the two regions more or less strongly by generating more or less of the subspace patterns,” Kohn says. For example, if an animal wants to weight visual over auditory information to make a decision, it can modify activity in a communication subspace corresponding to a particular downstream area, presumably more quickly and fluidly than it can adjust synapses. “It may be a way of selectively and dynamically routing signals among different parts of the brain based on the task,” Kohn says.
The Neuron study focused on fluctuations rather than activity tied to the stimulus or task itself. Researchers are now looking at the content of the information being transmitted in the subspace. For example, if animals are trained to pay attention to different features of the same visual stimulus — say, the orientation or frequency of a striped grating — researchers can examine how subspaces change to communicate the relevant information downstream. They can also look at subspaces on trials where an animal makes a mistake. “On error trials, does activity not get transmitted from one area to another because it’s not aligned well with the communication subspace?” Kohn says.
The same methods scientists use to study subspaces in brain activity can also be applied to models that scientists develop to explain this activity. “These observations about subsets of activity that are and aren’t shared will place strong constraints on models,” Cohen says. “This will be a way to put experimental data and models on common ground so they can be compared.”