Understanding How the Brain Works, One Model at a Time
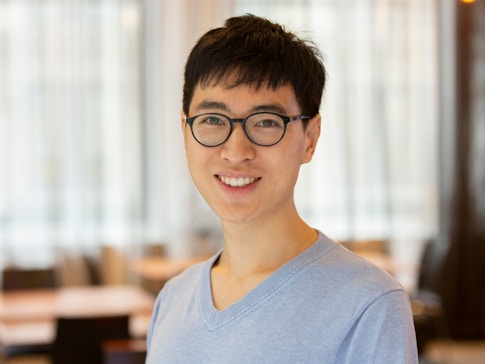
Even the most advanced computer is no match for the brain. Composed of 100 billion cells that together form trillions of connections, the human brain is perhaps the most complex object in the universe.
To make sense of its complexity, scientists are now developing mathematical models that go beyond what can be observed in the lab to guide a deeper understanding of the brain and its unmatched abilities. Guangyu Robert Yang, a junior fellow with the Simons Society of Fellows, creates these computational tools to help solve the mystery of what happens in our minds as we perceive the world.
Yang studied physics as an undergraduate at Peking University from 2008-2012. This gave him a solid mathematical foundation that he now uses as a theoretical neuroscientist. He earned a doctorate from New York University in 2018 and soon after began postdoctoral research at Columbia University’s Zuckerman Institute, under the mentorship of Larry Abbott and with support from the Simons Foundation. Now entering his final year at Columbia before becoming an assistant professor at the Massachusetts Institute of Technology, Yang recently spoke with me to discuss what he has uncovered so far and what he hopes to do next.
Our conversation has been edited for clarity.
You build something called neural networks. What are they and why are they useful?
Neural networks are mathematical models inspired by how information flows through the brain. Decades of work has revealed that an individual brain cell, or neuron, can only complete simple tasks on its own. Their true power lies in how they work together.
Neural networks are a way for scientists to represent this information flow abstractly. They help neuroscientists uncover patterns in brain activity that might not be apparent from the results of a single experiment. And it’s not just neuroscientists. Psychologists and people who study machine learning use neural networks too.
In essence, neural networks are like decoder rings, with infinite uses. That’s pretty cool! Besides building neural networks, what is the role of a theoretical neuroscientist like yourself?
Neuroscientists study how the brain and central nervous system work, usually in a lab. They can track the activity of individual neurons sending pulses of electricity from one neuron to the next — like data being sent along a telephone line. It’s from this collective activity that interesting perceptions emerge.
Let’s say a neuroscientist performs an fMRI scan to learn exactly what happens in someone’s brain when they see a giraffe, which seems simple enough. Those scans are likely to show greater neuronal activation in some parts of the brain than others, for example in the visual cortex. The neuroscientist could describe what happens in words. She might say that this part of the brain “lights up” when the giraffe appears. But using words to describe this process is ambiguous, because the same word means different things to different people. A complementary and arguably more powerful approach is to build mathematical or computer models that aim to precisely define the connection between what happens in the brain and observed behavior, thus removing some of this ambiguity. This is the work that the field of theoretical neuroscience — sometimes called computational neuroscience — does.
Experimental and theoretical neuroscience are two sides of the same coin. Without the hard work of experimental neuroscientists, theoretical neuroscientists like me would have no data to develop our models. And I like to think that our theoretical models help our experimental colleagues think of new questions to ask and test. It’s a true partnership.
Why are theoretical models so critical to the field of neuroscience?
Let’s say a psychiatrist is treating someone with schizophrenia, a complex disorder that — broadly speaking — occurs when certain neurons do not operate in a typical way. The psychiatrist may prescribe a drug to treat schizophrenia but doesn’t necessarily know how it works on a detailed level: how it changes neural activity in the person’s brain to alter behavior. A theoretical model could potentially help improve our understanding by making a formal prediction of what happens at every step along the way after someone takes a drug. We could then test these predictions — first in animals, and eventually in humans. Models offer a way to build, test and refine hypotheses. They give us a road map toward a better, deeper understanding of complex disorders such as schizophrenia.
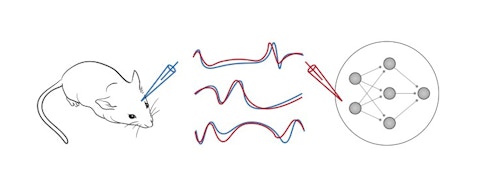
What projects have you worked on as a Simons junior fellow?
A significant area of focus has been developing a better understanding of the olfactory system, our sense of smell. We already know which neurons guide our sense of smell, but we don’t know why these neurons work the way they do.
We developed and trained a neural network to react to different smells as a way to begin to understand the evolution of the olfactory system. Over time the neural network we built became similar to the olfactory network in the fruit fly. We suspect we would see similar results in other animals, maybe even in humans. This gave us some ideas about why our sense of smell evolved as it did. What we’ve discovered so far is tantalizing, and something we continue to investigate.
Another area of focus and passion of mine is building a culture of openness and collaboration. Neuroscience involves designing clever tasks that animals or humans do, and then measuring brain activity during the task. The trouble is that most results of these tasks — the experimental records — live in silos, often on different people’s computers. This is a problem because many people design similar tasks; they could learn from each other if data existed in a centralized place.
Making research more open is a push in many scientific fields today.
Yes, exactly. The ideal case would be a central platform that housed every neural network and experimental result from neuroscientists around the world. With that in place, we could see if a neural network that worked for one experiment could also explain the results of another experiment. We could test our models at scale.
It will take a long time to reach this point, but it is something on which I plan to focus throughout my career.
That seems useful and ambitious! What else will you work on after leaving Columbia next year?
Next July I will become an assistant professor in the department of brain and cognitive sciences at MIT. While my focus will ultimately depend on funding and staff, I already have some ideas of where I want to start!
For example, I’m interested in expanding the scope of what our theoretical models can accomplish. Right now, it’s basically a one-to-one relationship: We develop a model that can inform a single experiment. But we should be building a model that explains the results of 20, 30, 50 experiments across many labs and many tasks. This could inspire a deeper understanding of human cognition, and it’s something I want to push toward.
I’m also interested in figuring out what it means for a neural network to ‘understand’ something. Today we can present a neural network with an English sentence, and it will produce another sentence in response. That seems like communication, but does the neural network truly understand what it’s doing? Or let’s take people. Someone could read a book and understand all of it, some of it, or perhaps nothing at all. What’s the mechanism in the brain behind these differences? Those questions are interesting to me, and they’ll keep me busy.