
In Foraging Tasks, New Opportunities to Study the Brain’s Complexity
In 2015, neuroscientist Dora Angelaki had a midlife crisis. She had spent the past 15 years or so conducting cutting-edge research on multisensory decision-making, but she had a nagging feeling that her experiments weren’t addressing the core questions she cared about.
Angelaki, like many others in systems neuroscience, had been using behavioral tasks with very limited outcomes: sink or swim, left or right, go or no-go. While these frameworks have been useful for describing decision-making and measuring behavior, they are limited in what they can tell us about complex cognition and the brain’s ability to adapt to new challenges.
The monkeys trained in Angelaki’s lab, for example, were good at performing the task they had been taught, but they failed to generalize their skills. As soon as researchers changed the details of the stimulus or any other small aspect of the task, the animals had to learn anew. “What I think is most fascinating about the brain is that we don’t have the same experience twice — we can generalize, we are flexible, we can use all the internal models we’ve learned from previous experiences to interpolate and do new stuff,” Angelaki says. “But we weren’t studying those behaviors.”
Angelaki, now at New York University and an investigator with the Simons Collaboration on the Global Brain, is not alone in her frustration. Many in the field of systems neuroscience worry about how applicable this type of highly controlled research is to other tasks or species, or to neurological conditions in humans. As a result, many researchers are turning to more complex, naturalistic tasks that fall under the umbrella of ‘foraging,’ in which an animal needs to get to know its environment and make choices about which kinds of behaviors are worth its while. Such tasks are quickly becoming ubiquitous in systems neuroscience research, as many scientists start to appreciate that they strike a manageable balance between controlled and naturalistic. Because almost every creature from a fly to a primate needs to search for resources, foraging tasks can be implemented across a range of model systems.
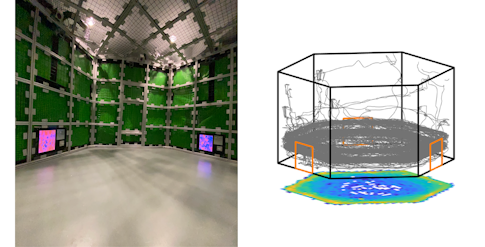
Over the last few years, Angelaki has developed a new range of naturalistic and dynamic experiments that can better capture cognitive sophistication by letting monkeys forage in a freely moving arena or in virtual reality while she records from multiple brain areas.
Efforts like Angelaki’s are made possible in part by recording devices and tracking algorithms that are expanding our capacity to listen to neurons simultaneously and automatically categorize animal behavior. And with tools to record from hundreds or even thousands of neurons during foraging, researchers can, for the first time, map these complicated behaviors onto brain activity.
The data is complex and will require development of novel analysis pipelines and computational models. But these efforts are already leading to surprising observations about how the brain works — for example, some experiments have shown that hippocampal neurons may encode more features of the task than originally thought. “The real benefit of doing the naturalistic task is that it pushes you into completely new mental directions, completely new ideas that you didn’t have before, completely new questions you weren’t even able to ask before,” says Benjamin Hayden, a neuroscientist at the University of Minnesota in Minneapolis.
A ripe time for foraging
Each of us has foraged to some degree — looking through an open cupboard for snacks, searching for the nearest trash can, remembering that the conference room down the hall often has leftover pizza. Almost every creature forages in one way or another, most often for food but sometimes for shelter or mates. Foraging involves both a reward and a cost, such as the energy spent searching for food or the risk of encountering a predator.
The complexity of the foraging repertoire contrasts sharply with the simple, highly structured tasks typically used in neuroscience. Those tasks have been constrained in part by technical limitations — because neuroscientists could record from only a few neurons at a time, they had to synthesize data from the same repeated task to draw conclusions. The need for repetition has dwindled with the advent of large-scale techniques capable of recording from many neurons simultaneously and making it feasible to study the neural basis of complex, naturalistic behaviors such as foraging. “A lot of traditional neuroscience has tried to simplify the task as much as possible to get the most constraints on the behavioral data and the neural data,” says Xaq Pitkow, a researcher at Rice University. “That makes a lot of sense when you’re really limited in how many recordings you can get, but I think that the time is ripe to move on beyond that.”
The ability to simultaneously record neurons not only enables the study of more complex behaviors, but may actually require it in order to accurately explain the neural activity. If the complexity of your neural recording is much greater than the complexity of your task, you will enable a sort of curse of dimensionality. If you are asking whether specific neurons encode ‘lick left’ or ‘lick right,’ you will inevitably find lots of neurons in all sorts of brain regions that seem to correlate with left more than right. “If you’ve got tons of neurons and brain regions, you’ll see neural correlates of your low-dimensional behavior in many brain regions,” says Karel Svoboda, head of the new Allen Institute for Neural Dynamics, which will use foraging tasks in mice during optical imaging and manipulation. The institute’s forthcoming experiments will include a whole battery of behavioral variables that can be used in regression models as a way of explaining neural activity. “The idea is that if you look at more, higher-dimensional behavior, you’ll have more to regress against,” Svoboda says. Richer behavior gives researchers more and better ways to explain the neural data they’re seeing.
Foraging studies are already broadening our insight into the brain. In a project for the SCGB, Angelaki and Pitkow developed a task in which monkeys ‘catch’ fireflies in a virtual open field. As the animals hunt, researchers record from multiple brain regions simultaneously, including the frontal and parietal cortex and an area called MST, a sensory area responsible for encoding visual motion. As described in a preprint posted earlier this year, the researchers found signals related to the task in a variety of areas, including MST. “When you use naturalistic tasks with action-perception loops, the sensory areas are not isolated from the rest of the brain, and they are not feedforward only,” Angelaki explains. The findings echo work using other tasks in mice, in which areas classically considered sensory seem to encode task-related variables. (For more, see “Thinking on the Go: Why Does the Whole Brain Light Up for Just the Smallest Movements?”)
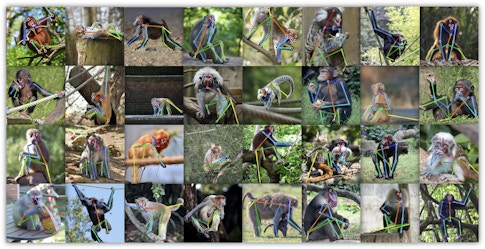
Foraging approaches may capture different aspects of behavior than more constrained tasks. Hayden studies monkeys freely foraging in an open area while he automatically tracks their movement and wirelessly records from the brain using a miniaturized recording system with its own onboard SIM card. It’s a welcome change from the classic gambling experiments of his grad student and postdoc days, he says. In many economics-inspired tasks, the monkeys’ behavior is impulsive and risk-seeking. But Hayden suspected that this behavior was a product of the task rather than a reflection of the animals’ natural behavior. As technology has advanced to enable freely moving behaviors during recording, Hayden has been able to show that monkeys are less impulsive when they’re faced with more realistic challenges.
New insight into memory circuits
Frameworks that mimic foraging have also breathed new life into classic hippocampal memory tasks. In the wild, flies, rodents and monkeys all need to search their environment for food and to remember where they have previously found a stash. These searches can be ‘goal-directed’ — seeking a previously identified location — or more open-ended, with the animal seeking to learn about new locations.
“We know that many ethologically relevant navigational strategies involve goal-directed navigation — back to a remembered food source or home location — which inspired us to incorporate this element into an open-field arena,” says memory researcher Lisa Giocomo of Stanford University. To elucidate how the hippocampal formation encodes memories related to foraging, her lab developed a task in which rats needed to forage in an open arena with an uneven distribution of food. In a 2019 paper, they showed that spatial maps in the entorhinal cortex could adapt to new reward locations, and that this improved the animal’s ability to discern its position in those locations.
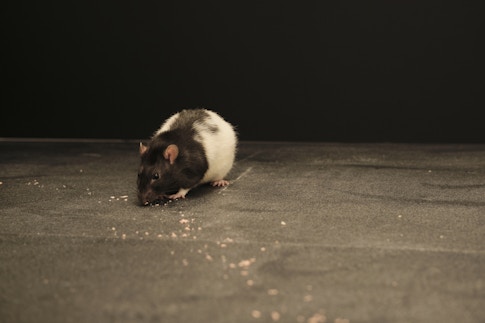
These experiments inspired Elizabeth Buffalo, a learning and memory researcher at the University of Washington, to develop a virtual foraging task in monkeys that could probe learning and memory without relying on overtrained behaviors. “We’re interested in episodic memory formation that’s different from overtrained tasks,” Buffalo says. “Our hope is that in VR we can make things naturalistic enough to then be able to capture experiential learning built on a framework that we can control.”
Buffalo was specifically interested in understanding whether the hippocampus encodes memories the same way in primates as it does in rodents. What her team found surprised her. In a large 3D foraging environment, she couldn’t easily identify the place cells characteristic of the rodent brain. Instead, the cells in the hippocampus seemed to encode the different stages of the task that the animals were performing. That insight was made possible by the combination of large-scale recordings during a naturalistic task.
“Having the large-scale recordings was really critical for seeing this kind of representation of the tasks across the population,” Buffalo says. “If you were just looking at the data neuron by neuron, you might say that this structure has a really complicated mixture of responses: place, gaze, reward, delay, etc. But if you instead take a look at it at the response of the population, what you see is that the population activity really carves out behaviorally relevant epochs in the task.”
With more complex tasks and plentiful neurons, researchers are also able to identify differences between individual animals. Like humans, laboratory animals have different personalities — one animal might be risk averse, while others might be prone to taking chances. With enough data from each individual, researchers can examine the neural mechanisms underlying individual differences in behavior. “There’s a big opportunity to start going after individual-difference neuroscience, which is a big and important thing,” Hayden says. “This is just an example of the kind of thing that gets uncovered when you make things more naturalistic, and you move away from these highly controlled, simplistic tasks.” Hayden’s parallel work recording neural activity in humans is starting to demonstrate that different brains may compute tasks differently, a finding that has major implications for the way we approach neurological disorders.
Tackling the complexity of rich naturalistic datasets
Researchers analyzing stereotyped tasks, where an animal chooses between two or three options, have an established toolbox to draw on. They can align their neural data by specific events that occur across trials, such as the animal’s lever press or the delivery of a reward, and average the data to derive an event-evoked neural signal. But without the familiar trial structure of a two-choice task, the analysis landscape for naturalistic tasks looks a little bit like the wild west. Researchers have applied behavioral economics and ecological frameworks to foraging, but many of these models do not generalize well to more complicated foraging tasks. Moreover, researchers are collecting data from more and more neurons simultaneously, adding another layer of complexity to the task of understanding the experiment as a whole. As a result, many researchers are turning to both old and new theoretical frameworks to make sense of the data.
“The most challenging thing in these experiments is actually not the [recording] technology, but the data analysis,” Angelaki says. “We cannot take off-the-shelf analyses. I need to collaborate with data scientists because we really need to develop our own algorithms.” Her lab, in collaboration with Cristina Savin at NYU, is adapting algorithms such as canonical correlation analysis and demixed principal component analysis to use with their neural foraging data.
Angelaki and other experimentalists are also reaching out to theorists who can use computational modeling to make sense of the data. Buffalo is teaming up with Adrienne Fairhall and Xiao-Jing Wang, both SCGB investigators, while Angelaki is collaborating with Pitkow, Savin and Paul Schrater of the University of Minnesota to test reinforcement learning frameworks. Reinforcement learning models, in combination with deep learning, can be used to understand the kind of mental models that animals are developing while doing the task, and can in turn help us understand the brain.
During a task, experimenters have access to external variables, such as the animal’s movement and choices. However, they do not have access to the animal’s thought processes or its evolving understanding of its environment. Behavioral modeling helps researchers identify these hidden, or latent, variables and use them to explain the neural activity — Pitkow dubs this a sort of “mathematical mind reading.”
A good mental model for the animal is helpful because then we can impute these latent variables — an estimate of the animal’s internal beliefs — as a function of time, Pitkow says. We can then check if the latent variables calculated from the behavioral model match what’s going on in the brain, and specifically whether the evolution of neural activity is consistent with the evolution of the latent variables, he says. Using foraging tasks lends the ability to build a much richer model of the behavior and ultimately distill latent variables that may not even be present in a simple alternative forced-choice task.
Hayden, Angelaki, Pitkow and others are also digging out old engineering textbooks and thinking about their data in terms of control theory, a dynamical systems model that situates the brain as a ‘controller’ and can help quantify the importance of feedback loops and latent variables.
Other researchers, like Sue Ann Koay, who started her lab last year at the Howard Hughes Medical Institute’s Janelia Research Campus in Virginia, are thinking up radical new ways to devise hypotheses about brain function. In Koay’s randomly generated virtual environments, mice do not know the configuration or even the extent of the world they are in, so there is not a well-defined way to complete the task. Mice can find food in unexpected places in a world that may never end and may sometimes even distort the normal geometry of space, as in a science fiction movie.
Many ecological models optimize for an efficient solution to foraging tasks by balancing time and resource trade-offs. Koay wants to explore models that are more biological in nature. She plans to use these models to inspire hypotheses about the ways brains can work, employing ideas from evolution to constrain her models. “I would like to have some method of exploring brain-like solutions that is not tied to a human-formulated list of tasks that they should solve,” she says. “The only thing I could think of that is more general is evolution.”
Koay is part of a broader community of young researchers who are thinking about foraging in new ways, developing novel methods to generate hypotheses about how the brain facilitates foraging. Other early-career researchers are working on ways to bring ethology back into the conversation, hoping that neuroscientists can learn from their wealth of knowledge.
Last year, a team of trainees from both Janelia and the Champalimaud Centre for the Unknown in Lisbon kicked off the Future of Foraging seminar series, which features neuroscientists like Hayden as well as ecologists and ethologists who have been thinking about the foraging brain since long before we were able to record thousands of neurons simultaneously. Laura Grima, one of the co-founders of the series and a postdoctoral fellow at Janelia, is hopeful that a bridge between ethology and cutting-edge neuroscience is possible. “It is an overwhelming amount of data, even when you scale up some of these aspects just a bit, even if you don’t go the whole hog and just have mice running around in the wild,” Grima says. “I just hope as a field, we start to not be so scared of complexity and instead start to embrace it.”