
New Insight into the Hidden States of the Brain
Some nights when dinnertime rolls around, you find yourself craving takeout from your favorite neighborhood place. Other nights, you might feel more adventurous and decide to try a new fusion restaurant across town. The reason we react differently when confronted with the same situation often comes down to our internal state — feelings like hunger, fear or frustration that influence how we go from a situation, like dinnertime, to an action, like choosing a restaurant. These subtle differences can have a profound impact on behavior, pushing us to exploit what we know or to explore something new. “Internal states are what make you change your behavior based on your past experiences, history and motivations,” says Ann Kennedy, a neuroscientist at Northwestern University.
Even though internal states are a crucial ingredient in the pathway from experience to action, the way they influence behavior has often been overlooked, in large part because it can be difficult to identify the neurons that represent these states. Unlike with, say, decision-making, where researchers can look for neurons whose activity corresponds to an animal’s choice, determining when internal states begin and end requires a more creative approach. In some cases, scientists can induce a specific state, for example by scaring the animal, but these states can last for many minutes and shift at unpredictable times.
One approach is to observe a freely behaving animal and look for consistent shifts in behavior that can reveal its internal state. Detecting these patterns often requires many minutes or hours of observation. But once researchers identify behavioral patterns that reliably correlate with internal state, they can use them to isolate corresponding neural representations.
Even with behavior as a guide, determining which neurons are driving internal states can be challenging, requiring new tools that can simultaneously record from hundreds or thousands of neurons, along with analysis techniques that can make sense of these large datasets. “You need to see as many of the cells as you can,” says Jennifer Li, a neuroscientist at the Max Planck Institute for Biological Cybernetics. Once they’re identified, researchers can further probe how these neurons produce behavior, opening the door to a range of other experiments, including causal perturbations in which researchers directly manipulate the activity of particular neurons to confirm that they produce internal states.
Using these newly developed methods, neuroscientists are starting to understand how neurons coordinate their activity during various internal states to influence behavior. One common pattern that is emerging is persistent activity — sustained activity of groups of neurons over many seconds or minutes — which often occurs during long-lasting internal states. As neuroscientists expand their search to populations of neurons involved in internal states, they expect to see more complex and interrelated motifs emerge. “We are starting to uncover not just one … but many neurons that each carry distinct information, but in a way that seems somehow relational to the rest,” says Drew Robson, who co-leads a lab with Li. “Now we can ask much richer questions.”
A state of persistence
After a bad day at work, you might still be agitated when you get home despite having left the stress of the office. This long timescale extending past the stimulus that caused it is known as persistence. It’s a key feature that distinguishes behaviors driven by internal state from other behaviors, like reflexes. Understanding how states persist and what determines how long an animal stays in one is essential for deciphering how internal states influence behavior. Li and Robson addressed these questions by recording from zebrafish swimming around their tanks.
Li and Robson started with a simple premise: that changes in the internal state of an animal would manifest as consistent changes in the pattern of the animal’s behavior. After watching the zebrafish for hours, they noticed that the fish seemed to have periods when they swam around and periods when they would stop and hunt. “It seemed like there was this behavioral organization on the timescale of minutes,” says Li. “The behavior really just toggles between one state and another.” They mapped these behavioral patterns onto the already well-known concept of exploration and exploitation, with the zebrafish toggling between minutes-long periods of exploiting their environment by hunting and periods of exploring and looking for alternatives. The researchers formalized their observation by applying a hidden Markov model, commonly used to find hidden states, to quantify periods of exploration and exploitation. (For more on these kinds of models, see “In Mapping Mistakes, a Window to the Inner Mind” and “How Fruit Flies Woo a Mate.”)
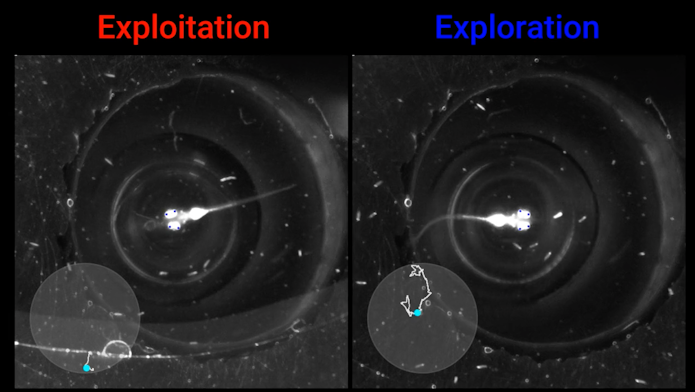
By clicking to watch this video, you agree to our privacy policy.
Using calcium imaging to simultaneously record the activity of neurons across the entire zebrafish brain as it swam, Robson and Li identified two groups of neurons in a brain region called the dorsal raphe nucleus that predicted the zebrafish’s transitions between exploitation and exploration states. The first subset of neurons was active when the fish was in an exploration state. The second subset rapidly increased its activity when the fish entered the exploitation state and then ramped down until the fish entered an exploration state again. The decaying activity of these neurons seemed to act like a clock, counting down the time the zebrafish spent in the exploitation state until it moved back to exploring.
Importantly, the amount of time spent in one state or the other was sensitive to the environment. Transitions could happen spontaneously, but they could also be triggered by aspects of the environment, like periods of light or dark. This flexibility allows the fish to toggle between exploration and exploitation, but also to favor one state over another when the environment indicates that it is advantageous.
Li and Robson’s finding is part of a growing body of work showing that slow, persistent decay of neural activity mediates the transitions between internal states. Kennedy, working with David Anderson, a neuroscientist at the California Institute of Technology and an investigator with the Simons Collaboration on the Global Brain, found a similar neural signature when recording from the hypothalamus of mice that became agitated after exposure to a rat. The frightened mice tended to run around the edges of their enclosure for several minutes after the rat was removed. “We could see that the neurons that were activated by the rat remained persistently active and decayed over many tens of seconds during the time the animal was running around the arena,” Anderson says.
When researchers blocked the activity of these neurons, the animals stopped running around the edges of the enclosure and returned to the center. “That says this is not just a correlation between persistence of behavior and persistence of an associated neural firing pattern, but that the neuronal firing pattern, the persistent activity, is required for the persistent behavior,” Anderson says. Persistent decay has also been observed in Caenorhabditis elegans moving between exploration and exploitation states, and in Drosophila moving between mating states, suggesting that this mechanism is a core part of how the brain controls internal states.
From single neurons to populations
Slow ramping up or down is a compelling feature of individual neurons, but a comprehensive picture of neural representations of internal states requires understanding how populations of hundreds or thousands of neurons in a given brain region encode information. Oftentimes, the diverse responses of single neurons don’t make sense until they’re put in the context of the larger population. One class of models, called dynamical systems, has been particularly useful in building this understanding, as they can reveal simplified representations in the neural data that evolve over time. In a recent study led by graduate student Aditya Nair, Kennedy and Anderson’s groups used these models to form a bridge between single-neuron signatures of internal state and population-level activity.
Kennedy and Anderson’s study began with a paradox. Male mice become aggressive when they encounter another male mouse. Optogenetically stimulating neurons in a region of the hypothalamus called the ventromedial hypothalamus (VMHvl) enhanced this aggressive behavior, but individual neurons were not selectively activated during attack behaviors. To resolve this paradox, the team used dynamical systems modeling to look beyond single neurons for patterns of activity across the population that could explain the animals’ behavior.
Dynamical system models identify small numbers of dimensions that describe how neural representations evolve over time. Each dimension is a weighted sum of the activity of all the recorded neurons. These signals are important because behavior requires the coordination of activity across several brain regions, and information is passed from one brain region to the next through weighted sums of the activity of many neurons. “So a dimension of neural activity is … a potential signal that [another] area could be using to generate behavior or do its own computations,” says Kennedy. The researchers identified one dimension whose activity gradually ramped up over time and aligned with the animal’s behavior.
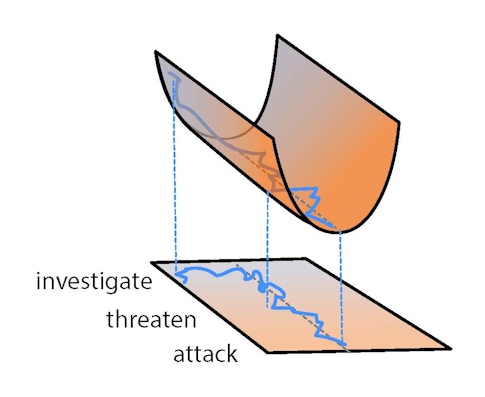
They noticed that the ramping activity in this dimension gave rise to a dynamical system feature called a line attractor. Once line attractor dynamics begin, neural activity persists in a stable state. To understand how this line attractor behaves, Anderson likes to think of the neural activity, which he refers to as a population vector, as entering a canyon. “The population vector dives into that canyon and moves along the floor of the canyon as aggression is escalating,” he says. No matter where the neural activity starts, it’s pulled into the canyon, launching a specific sequence of activity. In this case, movement along the canyon floor reflects a gradual increase in neural activity over time. This activity may represent an escalating aggressive internal state.
Activity along this dimension also predicted individual differences in mouse aggressiveness. The more aggressive mice showed VMHvl activity that was more persistent, spending more time in the furthest part of the canyon. In less aggressive mice, VMHvl activity would quickly progress along the canyon, but then return to the start. “It took not just finding a signal that looked like a ramp, but also finding this correlation between time constant and level of aggression that helped me believe that this was a real thing,” Kennedy says. The link between neural activity and behavior suggests that this low-dimensional representation could be the signal readout from the hypothalamus that produces behavior.
Intuitions about the dimensions of dynamical system models can also work in the opposite direction. By looking at what neurons contributed most to the line attractor, the researchers identified a subset of neurons that also showed ramping activity, similar to the kind of activity Li and Robson observed in their experiments. They hadn’t noticed these neurons before because they didn’t know to look for them until the model had identified this dimension. “I think there’s a lot of worry and skepticism that if you need a super fancy dynamical systems model to find a pattern, then maybe you’re squeezing that pattern out of the data,” Kennedy says. “But it’s a good way to get intuition for what a neural population is doing … and it’s reassuring to also be able to go back and reconcile that with the activity of individual neurons.”
For Anderson, finding a line attractor in the hypothalamus was an exciting step forward in applying these models to novel brain regions. Structures like line attractors are typically associated with functions attributed to the cortex and hippocampus, such as decision-making. “So the finding of a line attractor in a particular region of the hypothalamus that correlates strongly with a specific behavior … is extremely important and novel,” he says.
Beyond persistence
Persistent neural activity is an emerging hallmark of internal states, but it’s not new to neuroscientists. Persistent neural activity is a signature of evidence accumulation in brain regions involved in decision-making, as well as a means of holding items in working memory through neural activity in the prefrontal cortex. Kennedy sees these as examples of how fundamental persistence is as a neural computation across many domains. “This is just something you have to do to make sense of your world and survive,” she says. “You have to keep a running record of what you’ve encountered in the past and be able to integrate signals over time instead of just perceiving the world moment by moment.”
Persistent activity is likely just one of several neural motifs that work together to produce an internal state. Neuroscientists are working to uncover additional motifs by recording for longer periods of time across a wide range of behavior. As new components emerge, one of the next challenges will be to fit them together. “Now that we’re starting to find neural substrates for individual pieces of internal states, it’s like we have a partial parts list,” says Robson. “Now we’re starting to learn how it all fits together.”
Neuroscientists are also eager to understand how different states interact. Neural signatures of states like fear, aggressiveness or hunger are associated with activity in particular brain regions, but understanding how these states interact requires looking beyond individual brain regions toward how they relate to one another. “That’s a big question for theory and modeling,” says Kennedy. “How can these regions interact to shape behavior such that behavior is adaptive and flexible — so that you’re not ignoring a predator just because you’re hungry?”
Li and Robson are examining how states interact by extending their recordings from two to 24 hours. “You start to see a lot of interesting complexity that emerges,” says Li. “You can see … the organization of how these states interact and transition and also change over a longer timescale.” Longer recording times paired with whole-brain imaging allows them to observe how all brain regions interact across many states — something that isn’t currently possible in larger model organisms like mice. “There are a lot of very exciting things to do just to understand the organization of complicated and intertwined internal-state spaces,” says Robson. “There’s certainly enough to fill a decade of very rich and exciting work.”