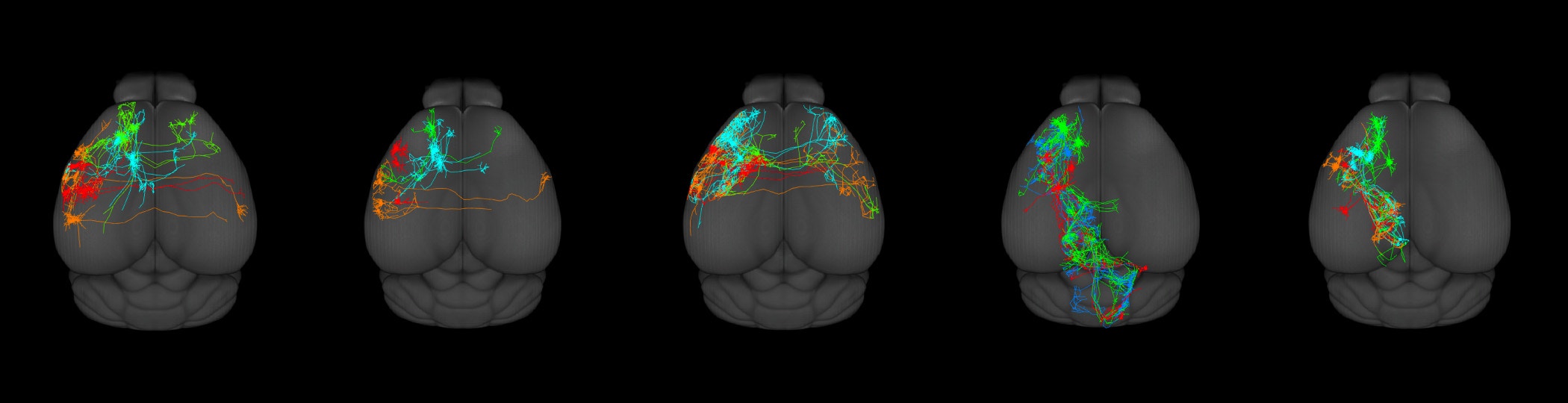
Crossing the Divide: Defining Neurons by Both Genes and Function
There are about 86 billion neurons in your brain, but they are far from identical.
Some have cell bodies shaped like a marble, others emulate pyramids. Some start in the cortex and reach deep into the brain, others sit in the brainstem and tick away on their own, largely unaware of other neural happenings. Some neurons are excitatory, compelling other cells to go, whereas other neurons are inhibitory, acting to prevent overexcitation.
Neuroscientists have long dreamed of a complete glossary of cell types in the mammalian nervous system, akin to the periodic table of elements in chemistry. But the ideal features to use as the basis for such a taxonomy are not immediately clear. Cell morphology was one of the earliest means of classifying cells and gleaning insight into their function. More recently, researchers have employed gene expression and bioinformatics tools to build hierarchies of cell types based on the similarity of their gene expression patterns. Alternatively, cells can be categorized based on developmental trajectory or functional categories, such as what circuit computations or behaviors they seem to be involved in.
An ideal glossary would consider each of these features. But historically, the study of the genetic or molecular makeup of neurons has unfolded largely separately from the study of their function. The earliest studies of neural function — which consisted of randomly poking cells and looking for responses that aligned with a stimulus or a behavior — were almost entirely devoid of any understanding of the neuron’s gene expression.
But that’s now changing. The development of a new arsenal of tools for measuring gene expression and for targeting cell types by both function and gene expression means that systems neuroscientists can finally draw direct connections between genes and function. Labs that never peeked at the gene expression of their cells are now running full single-cell sequencing experiments to understand their transcriptomic profile.
“Our ability to understand cell types has been dramatically accelerated by new technologies, especially single-cell technologies that are rapidly revolutionizing the field,” says Hongkui Zeng, director of the Allen Institute for Brain Science. “These techniques allow us to profile many individual cells in a comprehensive and unbiased manner and in a highly scalable way.”
Mapping function and connectivity onto neurons’ genetic profiles is opening a new window into the inner workings of the brain, revealing, for example, that genetically defined cells can have different coding properties and that groups of genetically defined cells have distinct patterns of neural dynamics. These efforts are also raising new questions, such as what facets of a neuron’s identity are best used to define it.
Targeting cells
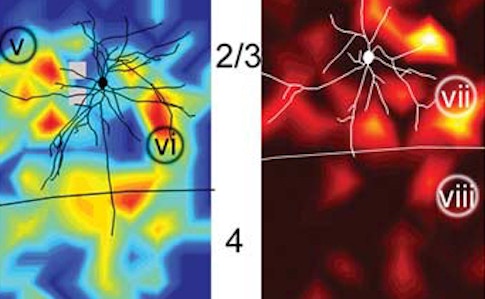
In systems neuroscience, the study of specific cell types in the cortex began with one major division: excitatory versus inhibitory cells. Ed Callaway, a neuroscientist at the Salk Institute, has been studying these classes of cells for the past three decades. His early publications reveal electrical recordings of neural activity alongside gorgeous reconstructions of various types of neurons — from excitatory cells shaped like pyramids with the ability to depolarize distant cells to smaller, inhibitory cells with quick action potentials sent only to nearby neurons. For decades, such electrophysiology and morphology data dominated the field of systems neuroscience and helped us build a starting glossary of cell types in the cortex. Callaway and others also started documenting differences between inhibitory neurons, finding that a few subtypes, such as parvalbumin-expressing ‘basket’ cells, could be defined by both the expression of surface proteins and their shape.
In the early 2010s, researchers gained the ability to genetically target specific populations of excitatory and inhibitory neurons for experiments. Several research groups, as well as the Allen Institute, unleashed a Noah’s ark of different mouse lines with genetically engineered cells, enabling researchers to study specific types of inhibitory neurons. In parallel, researchers could use viral approaches or reporter mouse lines to study the brain when these neurons were inhibited, activated, or responding to a stimulus or task. A large body of research then dug further into the subtypes of inhibitory neurons, integrating these newer techniques to observe and manipulate populations of them at work. This research boom shed light on the computations happening within a 1-millimeter column of the cortex, showing, for example, that parvalbumin neurons play a key role in controlling the gain of neurons in the mouse cortex.
More recently, researchers have developed even more precise ways to target even more specific types of neurons based on their gene expression. “Now we’re starting to have access to target and manipulate not just classes of inhibitory neurons, but also classes of excitatory neurons,” says Anne Churchland, a Simons Investigator and neuroscientist at the University of California, Los Angeles. In contrast to earlier tools, which provided a window into local microcircuits, “that gives us insight into much larger-scale neural circuits.” Churchland’s lab has been using these tools to understand the involvement of excitatory cell types with different projections in decision-making tasks.
Other efforts to categorize cells focus on their expression of certain transcription factors, which regulate when and to what extent a part of the genome is expressed. One of these factors is FOS — it is induced when neurons are active and in turn regulates genes that control key features of synaptic and circuit activity. Many researchers use FOS expression to identify active neurons, but little research has been done on what it is actually doing in vivo and how FOS expression relates to a neuron’s function.
To address these questions, Harvard neuroscientist Chris Harvey teamed up with transcription researcher Michael Greenberg, also at Harvard, to study the role of these cells in the hippocampus, one of the brain regions responsible for our ability to form memories. The hippocampus has two functionally defined cell types that have long been of interest to neuroscientists: cells that form engrams, small circuits of neurons involved in storing memories, and place cells which encode spatial maps of our environment. Typically, engrams are identified by the expression of FOS. But it was not clear whether cells for spatial navigation were also FOS-induced.
Using a genetically engineered mouse line developed in 2007, Harvey and Greenberg showed that FOS-expressing cells can form reliable and long-lasting maps of their environment, according to research published in Nature in August. Furthermore, FOS-induced cells are also highly correlated with other FOS-induced cells that encode different locations in space, which may be how the brain unites the encoding of space with our memories. By choosing to focus on cells that show induction of a particular transcription factor, Harvey’s group linked research on memory engrams to cognitive map theories of hippocampal function.
Prediction errors
Over the past decade, the process of tracking transcription factors has evolved dramatically, from sampling just a few genes or transcription factors at a time to measuring the entire transcriptomes of thousands of single cells in one experiment. Zeng helped usher in these advances at the Allen Institute. Seeing the power of using even limited sets of transcription factors to differentiate neurons, she knew that single-cell sequencing was the way to move forward, and her team began profiling the gene expression of single neurons. In 2018, her team published a landmark paper in Nature, along with an expansive, publicly available dataset containing single-cell transcriptomes of about 25,000 cells from two areas of the mouse cortex, concluding that there are 133 transcriptomic cell types. This publication has been cited almost 1,000 times in just four years, not only because it establishes a taxonomy of mouse cortical cell types, but also because it provides an essential reference dataset for integrating gene expression data with other data.
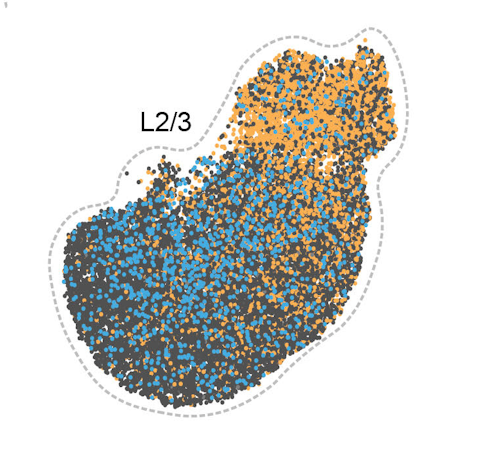
Georg Keller, a neuroscientist at the Friedrich Miescher Institute for Biomedical Research in Basel, Switzerland, is using the Allen Institute resources to better understand the role of different neurons in predictive coding, the ability of the brain to detect mismatches between what it expects to happen and what actually happens. Previously, his group and others have shown that there are different sets of cells that seem to encode positive or negative prediction errors — signals that a stimulus was stronger or weaker than expected — but they have not had a clear way to target these cells for investigation. (For more, see “The Challenges of Proving Predictive Coding.”)
To dig into the cell types that encode different types of prediction errors, Keller adapted a technique demonstrated by Gina Turrigiano’s group where researchers isolate neurons by their function using a fluorescent tag, sequence the RNA of those neurons, and then design Adeno-associated virus vectors to target those neurons specifically. In essence, this can allow researchers to close the loop between gene expression and function.
Using this technique, Keller’s group showed that the positive and negative prediction neurons were also separable based on their genetic identity. They’re not completely different — there are some genes that are co-expressed — but they are different enough that the researchers could use previously identified differences in RNA expression levels to separate them. Now, using these tools, Keller and other researchers can study these cells selectively to fully understand their role in predictive coding.
Mapping the habenula
Deep in the brain lies the habenula, an evolutionarily old brain structure that is involved in a variety of emotional tasks, such as avoiding stress and making decisions. The habenula has two well-studied and mutually exclusive cell types: those that express TAC1 and release neuropeptides, and those that express CHAT and release acetylcholine. But for years, the field has been grappling with observations that there are many more than two cell types in the habenula. Mapping the multitude of tasks that habenula neurons tackle onto clear molecularly defined cell types hasn’t been possible — until now.
In research published in Cell in September, a team of researchers in Karl Deisseroth’s lab set out to more precisely define the cell types in the habenula using a combination of molecular and computational approaches. They used a technique called STARmap, developed in the Deisseroth lab, to read the transcriptomes of the neurons in intact brain tissue. STARmap is part of a family of techniques known as spatial transcriptomics, which enable researchers to obtain gene expression profiles of cells while preserving their spatial arrangement.

This approach identified four candidate genes that defined transcriptionally and spatially distinct populations of cells: TAC1, CHAT, TH and CALB1. Using genetically modified mice to target cells that expressed these four different genes, the team also found that they project to different regions in a downstream brain area, the interpeduncular nucleus, and that they play different roles in a decision-making task. Finally, they developed an imaging-friendly version of a computational technique called LFADS, designed to infer single-trial neural population dynamics, to show cell-type-specific population neural activity for TAC1+ and TH+ neurons. (For more on LFADS, see “Noise-Cancelling Method Enhances Ability to Predict Behavior From Brain Activity.”) While TAC1+ neurons increased their population activity across trials and exhibited line attractor dynamics, TH+ neurons did not change their activity, instead exhibiting point attractor dynamics.
There was no guarantee that these genetic markers would map onto the function of these cells in vivo. “We were excited that the molecularly defined cell types could be nicely mapped to distinct population activity dynamics and representations,” says graduate student and co-lead author YoungJu Jo. “Our study shows that cell typology [the transcriptomic profile] can aid in functional dissection even in a tiny nucleus.”
Although the transcriptomic profiles of neurons clustered reasonably well in the Deisseroth paper, this isn’t always the case. Callaway published a study in 2020 in which the team conducted single-cell sequencing in two populations of neurons with different visual response properties and projections to different visual areas. “We wanted to know whether these cell types are genetically different, and if we’d be able to target them based on such differences,” Callaway says. But unfortunately, those two populations of cells did not fall into distinguishable transcriptomic clusters.
Clear separation based on gene expression may not be possible for all populations of cells — it may be that it is easier to find transcriptomic differences between cells in more evolutionarily conserved areas deep in the brain than it is in the cortex. “A lot of the developmental mechanisms that specify those circuits are maybe more hard-wired,” Callaway hypothesizes.
In considering how best to merge transcriptomics with other cellular data, Callaway also points to the fact that most transcriptomic clusters have some overlap. When those clusters of cells are involved in different functions, researchers can make a clear case that they are different cell types. “If two populations of cells look the same, function the same and are connected the same, then your working hypothesis should be that they’re the same,” Callaway says. “Even if the gene expression data created clusters, they might not be different cell types.”
Merging data
Even with this array of new approaches, defining a cell type is a tricky thing. Neuroscientists are now collecting different kinds of data about their cells, and the ultimate goal is to pull it all together into a cohesive framework. What kind of data should dominate that framework, however, is still under debate.
“I would argue that what matters probably most is the computation that it performs — how does it contribute to the circuit?” Keller says. Harvey agrees but also notes the importance of gene expression. “I often think of cell type definitions in a genetic sense, with the hope that the function and the genetics map onto each other to some degree,” Harvey says. “I think that’s ultimately our goal: to try to map those two together in a bidirectional way, toward understanding how gene expression influences function and how a cell’s function influences its gene expression.”
Callaway is reluctant to name any single feature that should define a cell type. “I think you have to have different definitions for different questions,” he says. Still, he notes that gene expression isn’t everything. “There are many different combinations of the same genes that can give rise to the same function,” he says, referring to classic work by Eve Marder showing that a variety of ion channels and circuit configurations in the crab digestive system can result in the same functional outputs.
For Zeng, a multimodal approach is necessary, but it will almost definitely involve some form of transcriptomics. Circuit functions, for example, are not always stable. As Zeng points out in a recent review in Cell, physiological properties can change rapidly under different conditions, much more than a cell’s gene expression can. Zeng makes a distinction between cell type, which is more stable, and cell state, which may change because of network interactions or global brain activity. What’s more, scaling up in vivo studies of function is more challenging than scaling up gene expression analyses. She proposes using transcriptomics to define different transcriptomic cell types and then following up with studies of their function.
Zeng is especially excited about spatial transcriptomics and its ability to bring different types of data together. In an intact brain, researchers can watch cells in action and then use spatial transcriptomics to understand whether their function maps onto gene expression. “For us to understand cell type diversity, you have to be able to localize the cells precisely in space and see how they interact and how they fit into the circuit,” Zeng says. “Spatial transcriptomics is absolutely the next frontier for us to understand the cell type diversity and function better.”