Finding Order in the Chaos of the Cosmos
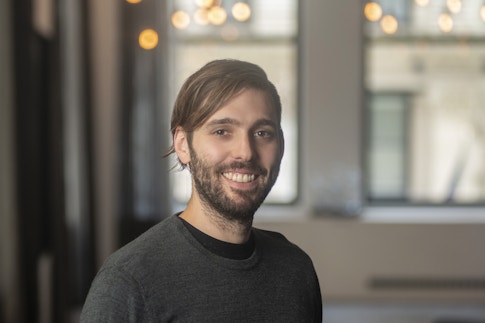
There are dark forces at play in the galaxy. Some so dark that they evade nearly all detection. But with innovative techniques at his disposal, astrophysicist Adrian Price-Whelan is beginning to uncover what’s lurking in the universe’s shadowy depths.
As a Flatiron Institute associate research scientist, Price-Whelan uses stars in the Milky Way to search for clues about dark matter. This mysterious substance, which astronomers first started cluing into in 1933, outweighs all the things we can see in the universe by a factor of 6 to 1. By mapping the motions of groups of stars, Price-Whelan can study how dark matter has shaped our galaxy. This work also helps astronomers understand the dynamics of distant galaxies that are too far away to study as closely as our own.
Price-Whelan joined the Flatiron Institute in 2019 as a member of the Galactic Dynamics Group at the Center for Computational Astrophysics (CCA). Prior to that he earned a doctorate in astronomy from Columbia University and was the Lyman J. Spitzer Jr. postdoctoral fellow at Princeton University.
He recently spoke with the Simons Foundation about his work and the mystery of dark matter. The conversation has been edited for clarity.
What is the focus of your research?
Along with a group of postdocs in CCA’s Galactic Dynamics Group, I study the Milky Way, our home galaxy. We are seeing how we can use information about the motion of its stars to draw general conclusions about the nature of dark matter and properties of galaxies throughout the universe. The Milky Way is the only galaxy we can study in great detail. By studying the Milky Way, we hope to use it as a Rosetta stone that we can then use to contextualize observations of other galaxies that are harder to see.
Historically, astronomers have run simulations and analyzed data based on necessary but simplified assumptions about galactic dynamics. A common one is assuming the Milky Way is in a steady state, meaning it always looked the way it looks now in terms of its broad properties and stellar motions. But there’s been a paradigm shift over the last 10 years or so because we’re now realizing that signatures of disequilibrium and disorder we see all over the place — such as a warp in the galaxy, spiral arms, and odd movements and large-scale motions of stars in the galaxy — are actually very significant. So now we’re trying to figure out what that disorder tells us about the Milky Way’s past.
What can that disorder reveal?
One of the things that disorder can tell us about is dark matter. Dark matter, as its name suggests, can’t be seen directly because it doesn’t interact with light. However, we infer its existence because we see irregularities in the movements of stars, which shows [that] there’s some large unseen mass. By studying these irregularities in movement, we can get a better understanding of what dark matter is, where it is and how much there might be.
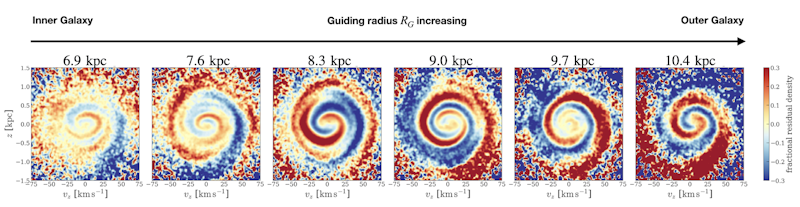
One of the ways I study dark matter is by looking at groups of stars that form stellar streams. The stars inside stellar streams used to be in bloblike star clusters, but over eons the gravitational tug of the galaxy has spread them out so that they form a long river of stars. Because the width of these stellar streams is very small compared to the sizes of galaxies, they’re sensitive to small-scale aspects of dark matter. By looking at different signatures in streams around the Milky Way, we can study properties of dark matter that aren’t reachable by any other method.
Another way I study dark matter is by using signatures of disorder in the nearer disk of the Milky Way. Recently, an observational survey of stars in the Milky Way called “Gaia” showed us that there are beautiful spiral patterns in the movements of stars in certain regions of the galaxy’s disk. These are fairly small compared with the whole galaxy — only a few thousand light years in typical size — but it was a surprising and interesting finding. I’ve been working on dynamical models to figure out how these features formed and what they are telling us about the local distribution of dark matter.
How do these features form?
We think spiral features like this form due to interactions between our galaxy and other smaller galaxies. For example, if a smaller galaxy falls into the Milky Way, it tugs at our galaxy and causes oscillations like you’d see if you looked closely at the head of a drum that had just been struck. This causes stars to oscillate up and down, which creates spiral patterns in the positions and motions of stars.
From our simulations, we know that these spirals get wound up tighter over time. This helps us figure out how long ago a galaxy might have passed through. We also know that the shape of the spiral is dependent on the amount of dark matter the galaxy has.
How do you study these features?
I work with huge catalogs of data that contain information like the properties of a star, where it is, how it’s moving and how bright it is. A lot of the features I’m looking at are quite subtle — they’re not the first thing you see if you look a group of stars. This means much of [the] work that I do is statistical modeling to remove the foreground stuff we don’t want, before we can study the details of what’s going on.