A Tale of Two Brain Regions: Population-level Analysis Reveals Differences Not Evident in Single Cells
Someone who suffers a stroke in the motor cortex, a brain area essential for movement, often ends up partially paralyzed. But damage to a neighboring region called the supplementary motor area, also involved in movement, can have more complex and mysterious outcomes: as one example, people may be able to move their bodies yet report that they just don’t want to.
Those observations suggest the two regions play different roles in generating movement. Scientists speculate that the supplementary motor area is involved in self-initiated movements, such as when someone decides to get up from a chair rather than being asked to do so. The motor cortex is thought to play a more mechanical role: creating the patterns of neural activity that will be conveyed to the muscles and actually generate the movement. That role would be the same regardless of why someone chooses to make a movement.
But neuroscientists have struggled to find further experimental evidence for this division of labor. They found, for instance, that the neurons in the supplementary motor area aren’t more active when an animal decides on its own to reach for an object. And activity of individual neurons in both brain areas looks broadly similar during reaching. “The supplementary motor area is active regardless of whether [an animal] is reaching in response to a visual cue or of their own volition,” says Mark Churchland, a neuroscientist at Columbia University and an investigator with the Simons Collaboration on the Global Brain (SCGB).
To resolve this conundrum, Churchland and his collaborator John Cunningham, also an SCGB investigator at Columbia, decided to compare the activity of populations of cells in the two regions. “If we believe that groups of neurons compute things by way of population dynamics, then different areas that have quite different jobs would exhibit quite different population dynamics,” Churchland says.
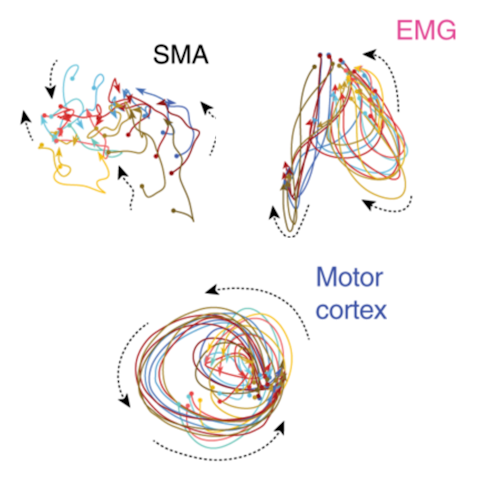
Their findings, published in Nature Communications in July, suggest this is indeed the case. “The dynamics you see in the primary motor cortex are not as prevalent in the supplementary cortex, which means the two areas have a fundamentally different role in motor control,” says Aaron Batista, a neuroscientist at the University of Pittsburgh, who was not involved in the study.
The findings highlight how analyzing populations of neurons can identify important differences not apparent in single-neuron activity.
Complex patterns
Populations of neurons produce complex patterns, and figuring out how to effectively analyze these patterns is a challenging endeavor. To study the dynamics of a collection of 100 neurons, for example, researchers map the activity of each neuron in a 100-dimensional space. Interpreting the resulting high-dimensional structure is difficult, so scientists start by stripping away some of that complexity. This downsizing involves identifying components of the structure that represent certain aspects of the population’s computational function, a general approach known as ‘dimensionality reduction.’
The most common method of dimensionality reduction, called ‘principle component analysis,’ picks out the most prominent features in the data, glossing over the rest. It might isolate a pattern of activity shared by 80 of the 100 neurons, ignoring activity patterns that are idiosyncratic to individual cells.
In 2012, Churchland, Cunningham and their collaborators, including SCGB investigator Krishna Shenoy of Stanford University, used this type of approach to analyze population dynamics in the motor cortex. They found that when a monkey reaches, population activity in this region exhibits a rhythmic, rotating pattern. The idea that a non-rhythmic movement — reaching — would be generated by rhythmic dynamics seems surprising, but Churchland and his collaborators had predicted this feature. They theorized that the motor cortex generates complex movements by essentially summing together rhythmic patterns.
Others in the field were skeptical, however, that the rhythmic dynamics were significant or unique to the motor cortex. Critics proposed that the rotational pattern might simply reflect the dynamics of muscles themselves or be a general property of neural circuits, rather than instrumental in driving movement.
Addressing this criticism has been no easy feat, in part because the field has yet to standardize methods for analyzing neural dynamics or settle on an optimal approach. Scientists use different techniques depending on what features of the data they are most interested in or what they think that region is doing, which makes comparing results with different analyses challenging. “There isn’t a one-size-fits-all data-analysis approach,” Churchland says.
Comparing methods
In a paper published in 2015, Cunningham and his collaborator, Zoubin Ghahramani of the University of Cambridge, outlined an approach to relate the different methods used to analyze population activity, such as dimensionality reduction or linear dynamical systems, by framing them as an optimization of a cost function. “All of these models have parameters, and you want to minimize some cost over the parameters,” Cunningham says. For principal component analysis, for example, the cost function acts to isolate the biggest contributors or response features in the data.
In the new paper, researchers used this general approach to produce a specific method to compare population structure in the motor cortex with that in the supplementary motor area. “We had a specific hypothesis about what kind of dynamics might be present and could substantiate that hypothesis in a cost function,” Churchland says.
The analysis confirmed that rotational dynamics are indeed present in the motor cortex and largely absent from the supplementary motor area. “It’s very clear that motor cortex dynamics are not shared with the supplementary motor area,” Churchland says. “That argues strongly that the two areas are performing quite different computations.” He notes that the fact that the supplementary motor area does not contain rotational dynamics acts as a control for the method, confirming that it doesn’t find this pattern indiscriminately.
“I think this paper is a crucial control that helps to shore up the idea that the motor cortex can build its own dynamics,” Batista says. “The dynamics in [the motor cortex] are not trivially explained by the behavior the animal is engaged in or by general cortical mechanisms but are specific to how the motor cortex does its job.”
Although the approach clearly shows that population activity in the two regions differs, the analysis doesn’t reveal what the supplementary motor area is doing. “We think the supplementary motor area is playing a high-level role, but we can’t tell what it is from the data,” Churchland says. He and Cunningham, along with Larry Abbott and Liam Paninski, also at Columbia, will explore this question as part of their new project with the SCGB.
Researchers are analyzing data from a different task, which they hope will provide greater insight into computations performed by the supplementary motor area. Their recent results provide some cues. Neural dynamics in the motor cortex are the same for the same movement, regardless of intent — if the animal decides to reach for something on its own or does so in response to a cue. But neural dynamics in the supplementary motor area are different under these two conditions. “Whatever it’s doing, it’s something higher level, which agrees with a host of prior findings,” Churchland says.
Cunningham is also developing new ways to more rigorously assess different methods for analyzing population level neural activity. In a paper published in 2017, he outlined an approach to determine whether population structure is an artifact of the method used to analyze the data or some other feature of the data rather than a true feature of the data. “In picking three dimensions of data that we think will show dynamical structure, it’s reasonable to be concerned that I have a powerful algorithm that invents structure not actually in data,” Cunningham says. “We need to be able to make a careful quantitative statement that says this structure did not happen by chance, that it’s not an artifact of the algorithm.”
Cunningham is working on these efforts with a number of collaborators, including many SCGB investigators. “We want to make sure the claims we are making are on solid footing,” he says. “This is an important consideration, especially as we do more and more population analysis.”