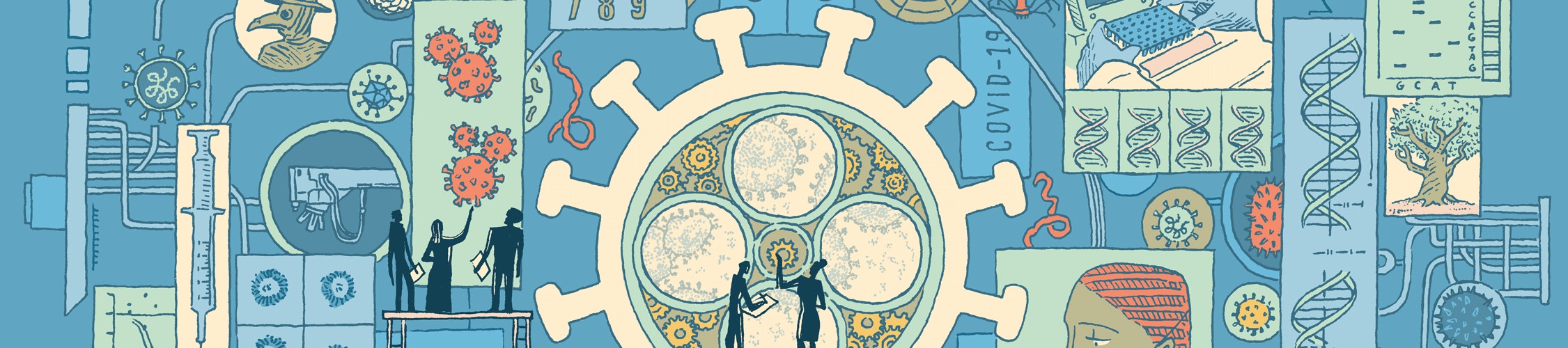
How Speeding up Science Aided the Fight Against COVID-19
As an influenza epidemic raged through Hungary in 1951, Gyula Takátsy, MD, had a problem. He was running out of critical influenza virus testing supplies, such as test tubes and pipettes. His solution to the shortage? The physician hand-machined a block of plastic with 6 rows of 12 indentations, each roughly the diameter of a pencil. Each indentation, or well, could be the locus for a chemical reaction formerly done in a test tube. Rather than needing 72 individual test tubes, he now had space for 72 reactions in a block of plastic little bigger than his hand. Called a microplate, this invention is one of the earliest examples of ‘high-throughput technologies’—a methodology central to the fight against COVID-19.
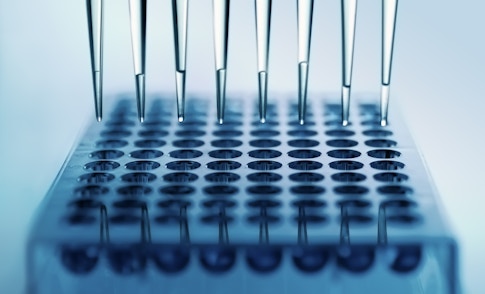
Speed is central to a technology being considered high throughput. Scientists and engineers take a task that is performed by hand—say, for example, transferring liquid from one test tube to another—and alter it so that in the time it takes a researcher to perform one action, now hundreds or thousands of such actions can be done.
Robotics and automation are key aspects of such technologies, says Deirdre Meldrum, PhD, director of the Biosignatures Initiative at Arizona State University and a founding editor of the journal IEEE Transactions on Automation Science and Engineering. When tedious and repetitive laboratory tasks can be performed with minimal human intervention, that frees up scientists for more thought-intensive work. Such technologies have further benefits as well. “Robotics are needed during research and development, when you’re optimizing some process or you need a robot to perform multiple functions,” explains Meldrum. “Automation is needed when you know your process and you want to harden it, so you have reproducibility, efficiency, and accuracy. You want to take out the human factor—variation.”
Refining the concept
Performing multiple tasks at once—a process known as parallel processing—is another factor that lies at the heart of high-throughput technologies. An early adoption of parallel processing in the life sciences can also be traced back to the innovative Hungarian physician. To batch-transfer liquid in and out of the wells he’d drilled, Takátsy designed spiral loops—similar to today’s inoculation loops (tools made of wire with a tiny loop at their tip)—that could reach into the wells and through capillary action absorb calibrated amounts of liquid, the way a pipette does. Whereas Takátsy simply held six or eight loops in one hand, today a robotic arm does the same thing, transferring precise amounts of liquid out of a microplate’s wells, multiple samples at a time. To match the number of loops he could hold in his hands, Takátsky further modified his plate to contain wells in an 8-by-12 format, inventing the first 96-well microplate, a format that is standard even today. Also called a microtiter plate, the microplate grew to replace test tubes in ever more numerous applications over the next several decades.
Robots that could transfer precise amounts of liquid into microplates arrived on the scene in the late 1970s. Their operation relied on a breakthrough in basic computing research: the invention of the silicon-gate transistor, which led to the first microprocessor. The microprocessor took the electronics formerly on multiple circuit boards and localized them onto a single tiny chip.
Invented in 1968 by a 26-year-old Italian physicist, Federico Faggin, PhD, who had immigrated to the U.S. to work for Fairchild Semiconductor in Palo Alto, the silicon-grate transistor revolutionized computing with speed and reliability. When the head of Fairchild, Robert Noyce, PhD, and others—including Faggin and Gordon Moore, PhD (of Moore’s Law, which holds that the number of transistors per chip doubles every two years)—left the company to form Intel, this technology went with them. Intel released the first commercially available single-chip microprocessor in 1971. Soon after, scientists in immunology labs were starting to use microplates to detect proteins; they were also the first adopters of the new robotic liquid handlers equipped with microprocessors.
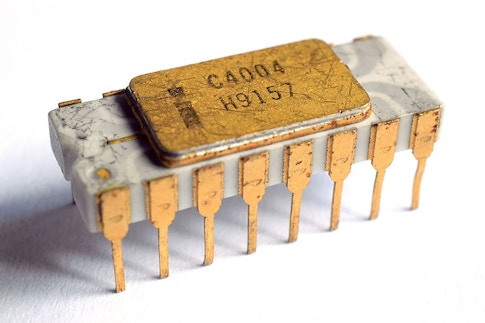
A shift in how scientists thought about drug discovery led to high-throughput methods migrating beyond immunology into drug discovery. At Pfizer, two scientists John Williams, PhD, and Dennis Pereira, PhD, developed the concept of high throughput drug screening and implemented it beginning in the mid-1980s. Their group wanted to create novel antibiotics. Their basic research challenge was as follows: How could they identify a single antibiotic-producing bacterium within a library containing 100,000 bacteria? To do so, they would need to scale up the pace of discovery from a few hundred a week to at least 10,000. Says Williams, “The key was the shift from test tubes to a fixed format, the microtiter plates.” Williams recalls the skepticism he and his team faced from other scientists: Would the chemical reactions work at smaller volumes? “Molecules are really small,” he says. “You just need the right concentrations and conditions; the volume is immaterial. The comfort was, having worked in recombinant DNA with reactions in 10 microliters, our labs knew they worked perfectly.”
The shift to a miniaturized well-plate format was just the beginning of ramping up lab productivity. “In those days, you could use an 8- or 12-channel pipette, which was leverage,” says Williams, referring to the ability to transfer liquid into or out of 8 or 12 wells at a time. “We decided it would be wonderful if we could do 96 at once, but the instrumentation was not readily available.” But by chance, as he was browsing through a trade journal, Williams noticed the Soken SigmaPet 96, a 96-well liquid transfer device. He bought eight but was told no one else wanted them. “Some people said, ‘We’re not sure if those reactions will work if you do them with a robot.’ I said, ‘Let’s set it up and compare the variance in dispensing. The robot will win every time.’”
By the late 1980s, there was a shift in the pharmaceutical industry toward high-throughput biology. By 1998, microplates had proliferated, but with enough variation to make the development of additional robotics for liquid-handling difficult to design for wide adoption. In January 1998, the Society of BioMolecular Screening worked on standard dimensions for the microplate, and “all the companies started building to this standard,” says Williams. In an article for the December 2000 issue of the Journal of the Association for Laboratory Automation, engineer Thomas Astle wrote, “This one change has been an important factor. . . . Today the offspring of the 96 well plate . . . are meeting the industry’s requirements.”
From genomics to “omics”
The shift to high-throughput research in biology was compounded by the promise of sequencing an entire genome, accomplished in humans by the Human Genome Project between 1990 and 2003. Hans Lehrach, PhD, the director emeritus of Germany’s Max Planck Institute for Molecular Genetics, says the shift from hypothesis-based research—in which a scientist seeks to answer a specific question—to discovery-based research was a crucial step. “We wanted to be able to do everything, the whole genome, and say, ‘Look at what we have!’” This shift to high-throughput technologies was logically obvious, Lehrach adds—an engineering development driven by scientists’ need for more genetic information as they sought to make new discoveries from vast datasets. “If we had continued to sequence the genome [in the Human Genome Project] in a hypothesis-driven fashion, we would still have not finished it and have spent a hundred times as much,” he says.
In fact, the Human Genome Project was made possible by tremendous advances in the automation of DNA sequencing technology. In the late 1970s, scientists painstakingly constructed the first genome of a virus by hand, using chemical reactions that could reveal the identity of a sequence, one DNA nucleotide at a time. Leroy Hood, MD, PhD, cofounder of the Institute for Systems Biology in Seattle, invented the first automated DNA sequencer; a commercial one was available in 1986. Hood secured funding for the effort from philanthropist Sol Price, the founder of Costco and Sam’s Club, after his idea was rejected by the National Institutes of Health as “impossible.” From there, a host of new high-throughput sequencing technologies, now referred to as “next-generation sequencing,” exploded. Such processes take place at unparalleled speed. While the sequencing of the first human genome took 13 years to complete, some of today’s fastest sequencers can complete 60 genomes in a day.

DNA sequencing “is a prime example of how advances in the life sciences enable new approaches in robotics and automation, and vice versa,” says Meldrum. Next-generation sequencing helped usher in a suite of new approaches known as “omics,” such as genomics and proteomics, in which scientists push to understand all the genes or all the proteins in a living system. Scientists invented tiny chips that could identify whether or not genes were turned on, thousands of genes at a time, plus specialized sorters that could separate individual cells from each other for single-cell analyses. Examining the inner workings of cells, in a comprehensive omics strategy, one cell at a time, lets scientists discover the heterogeneity in a given cell population—a factor that is especially important in samples like tumor cells or drug-resistant bacteria. Such single-cell analyses would not be possible without automation, says Meldrum.
Applying automation to COVID-19
In early 2020, these high-throughput technologies, refined over the course of many decades, suddenly became an important anchor in testing for COVID-19. “We had experience in outbreak-related DNA sequencing,” says Emily Crawford, PhD, a group leader at the Chan Zuckerberg Biohub (CZ Biohub), a research collaboration among the University of California, Berkeley; the University of California, San Francisco; and Stanford. “COVID-19 testing relies on RNA extraction and PCR, the bread and butter of biology,” Crawford adds.
Over the span of just eight days in March, the Biohub created the CLIAhub (CLIA stands for Clinical Laboratory Improvement Amendments, the required certification for clinical lab testing)—a COVID-19 testing lab capable of performing thousands of tests per day. This was accomplished mainly by repurposing equipment already on hand in the facility’s research labs and borrowing equipment from other labs on campus. Over 200 volunteer scientists—graduate students, postdocs, and professors—took part in the effort.
As scientists at the CZ Biohub harnessed their life science research infrastructure to test for COVID-19, so did scientists at other academic institutions, most notably the Broad Institute, a research collaboration among the Massachusetts Institute of Technology, Harvard University and Harvard-affiliated hospitals, as well as the University of Illinois at Urbana-Champaign (UIUC). At the Broad, machines running large-scale DNA sequencing projects were repurposed within days to diagnose COVID-19; they’ve processed more than 19 million tests since March 2020, including nearly 150,000 in one day. At UIUC, researchers launched the SHIELD, an effort to conduct large-scale COVID-19 testing for the campus community. By early 2021, they were processing 10,000 to 15,000 tests per day. Repurposing these research labs required obtaining clinical testing certification and recruiting skilled scientists, but the linchpin of the effort was the availability of the high-throughput robotics.
In the standard COVID-19 testing protocol, scientists extract from mucus or saliva samples fragments of RNA, the genetic material of the SARS-CoV-2 virus that causes the disease. A technique called PCR can hunt for the virus’s RNA. If it’s present, PCR will make enough copies of it that scientists can detect it. Robotics are essential to this process. First, the mucus and saliva samples must be transferred from collection tubes into microplates that have already been filled with chemical reagents. Robotic devices that can transfer liquid from one location to another not only speed up this process but ensure standardization. “An automated liquid handler is about four times faster than a person,” says Crawford. “But more important is the consistency automation provides for testing.”
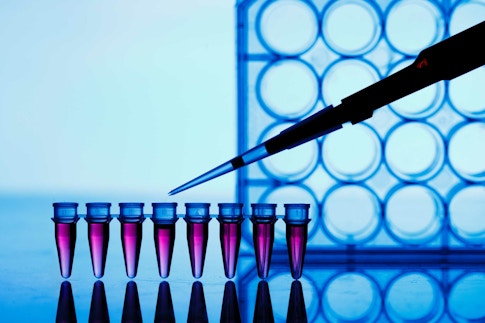
At the CZ Biohub-turned-CLIAhub, scientists sequence samples that test positive for COVID-19 to learn how the virus is spreading in the community—by, for example, pinpointing new variants from out of state or identifying local transmission hot spots. Prior to COVID-19, the CZ Biohub was working to set up a real-time global molecular monitoring network for infectious diseases, “a weather map for pathogens, to catch things just like [the COVID-19 pandemic],” says Joe DeRisi, PhD, a researcher at the University of California, San Francisco; copresident of the CZ Biohub; and leader of the CLIAhub’s COVID-19 testing efforts. “Next-generation sequencing lies at the heart of that,” he adds.
Parallel processing via rapid tests
Though the CLIAhub improved the testing capacity in the Bay Area, DeRisi points out some problems with the standard PCR method for COVID-19 testing. “Today’s robotics are both an opportunity and a pitfall,” he says. The CLIAhub and other academic COVID-19 labs benefit from nimbleness and from staffs of expert scientists unavailable at a typical county or state health department lab. Also, many academic labs have specialized PCR instruments known as sample-to-answer machines, which require specific plasticware and reagents that work only in that machine. As a result, many such labs have faced shortages of these proprietary consumables that have stalled their testing work. DeRisi’s lab analyzed supply chains and decided to use only reagents and plastics they could open-source. “You can’t run the machines if there’s no [fuel] in the tank,” says DeRisi, “and we made sure our cars didn’t run on just gas.”
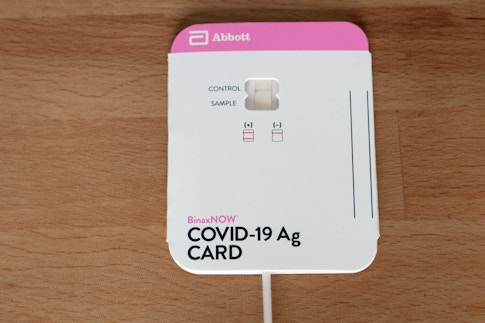
DeRisi points to another test for COVID-19—the rapid antigen test—as the ultimate parallel processing testing opportunity. Unlike PCR testing, which detects and amplifies viral RNA, an antigen test detects one or more viral proteins. In a rapid COVID-19 antigen test, a patient sample is added to a strip of paper, similar to a home pregnancy test. The presence of a colored line shows that an antibody—a molecule that specifically binds to a SARS-CoV-2 protein—embedded within the paper has bound to viral protein in the sample. In theory, hundreds of thousands of rapid tests could be distributed at once. “It’s paper-based, super cheap, and really easy,” says DeRisi. “You get an answer in 15 minutes. You have no supply chain issues, no reagents, no robot, no computer. Your kids should be able to walk into school, and the school nurse should have a stack of them,” he adds.
The main driver of today’s rapid test strip technology was in fact the human pregnancy test, developed in the 1970s in an NIH lab. In 1970, scientists knew the body secreted a hormone called human chorionic gonadotropin (hCG) during pregnancy and in the presence of certain cancers, but they had no way to precisely measure hCG in the blood. Researcher Judith Vaitukaitis, MD, who helped develop the hCG test, said in an oral history that she and her colleagues “had no idea of the impact [our work would have] on early pregnancy detection [and] abnormal pregnancy detection.”
The researchers also surely had no idea that 50 years later, their success in the basic science of hormone detection would lead to a test to diagnose a novel virus during the first global pandemic in a century. The first paper-based lateral flow tests entered the market in the late 1980s. In 2007, Abbott received approval from the U.S. Food and Drug Administration for a paper-based malaria rapid antigen test; it looks similar to the company’s new COVID-19 rapid antigen test but uses blood rather than mucus. If rapid antigen tests had been deployed routinely during the first year of the 2020 pandemic, their throughput would have dwarfed that of PCR testing. “That is where we need to be,” says DeRisi.
Pinnacle of high-throughput
Artificial intelligence (AI) approaches to fighting COVID-19 such as machine learning represent the pinnacle of high-throughput technologies. Machine learning expands on traditional robotics, which often imitates humans, to comb through mountains of data and make connections on a level beyond the abilities of individual scientists. Machine learning’s origins stretch back to the same physics breakthrough responsible for laboratory robotics: the transistor. Again, an invention and a need converged; the invention of the transistor propelled computing power forward, while the field of genomics provided the enormous datasets in need of robust, automated computation.
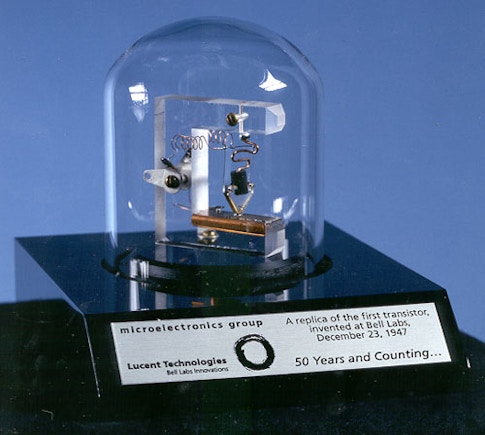
Machine learning is now aiding all aspects of the fight against COVID-19, from identifying networks of genes that are activated in the kidney cells of individuals with diabetes and that may predispose them to COVID-19, to screening images of cells infected with SARS-CoV-2 to find those responsive to drug treatment.
While necessity was the mother of invention for Takátsy in the 1950s, a shift beginning in the 1980s in how science was done established the high-throughput technologies that labs today rely on. Instead of querying a single gene or a handful of drug candidates, scientists have increasingly sought to make discoveries from millions of data points. First, they needed to generate such data—and advances in automated systems, such as liquid handling and DNA sequencing, brought forth the desired deluge of information. Then its analysis confounded researchers. So next, computer scientists developed platforms such as machine learning, deep learning, and AI to scour this deluge of data and hunt for correlations. As scientists use these technologies to combat COVID-19, one can’t help but wonder what will be in our arsenal when we need to fight the next pandemic—and what basic science research will uncover in the meantime that may prove useful.
Going forward, it will be important to fund a diverse array of basic science research projects—not just those that seem the most immediately fruitful or prudent. In some cases, as Leroy Hood discovered in the 1980s, private philanthropy may be the only way to push such research forward. The microplate, arguably the most common and useful tool in today’s research labs, took 30 years to catch on in the scientific community. Though now a success, it was not always viewed that way. Likewise, novel technologies of today, like single-cell analyses, may take years to become mainstream.
In research, says Meldrum, “you have to take calculated risks. If you have no failures, you’re being too conservative.”
About the Author
Susan Reslewic Keatley is a freelance science writer and aspiring medical thriller author living in Baltimore County, Maryland. She has a Ph.D. in Chemistry, and her interests include computational biology, genomics, and drug discovery.
This article first appeared as part of the Science Philanthropy Alliance‘s COVID-19 Basic Science Prequels series. Decades before COVID-19 gripped the world, basic science researchers were laying the groundwork for lifesaving medical and technological breakthroughs now central to the world’s pandemic response. With support from the Kavli Foundation and the Simons Foundation, the Science Philanthropy Alliance has enlisted a team of science writers to explore these science origin stories. Each month, the COVID-19 Basic Science Prequels will unpack the people, history, and serendipitous discovery behind topics that now dominate our daily lives.