
Building a New Model for Neuroscience Research
When the International Brain Lab first launched in 2017, its members knew they had a major challenge on their hands. IBL’s core mission is to create a brainwide map of a decision-making behavior, an endeavor that is simply too big for any one lab to tackle on its own. To accomplish that goal, the collaboration needs to produce and synthesize data across labs in a cohesive way that has never been attempted in neuroscience.
“Creating an entire brain map of a particular behavior is extremely ambitious,” says Catherine Dulac, a neuroscientist at Harvard University and the chair of IBL’s scientific advisory board. “The combined efforts across different labs would make it possible for the first time to understand behavior at a brainwide level.”
The first step was for researchers in 10 experimental labs from three countries to implement the same decision-making task in mice — and show that they could get consistent results. In a field where individual labs typically develop their own behavior paradigms, complete with idiosyncratic quirks (often left out of methods sections of published papers) that make them difficult to adopt elsewhere, this level of reproducibility was unheard of.
The first few months were tough, recalls Michael Häusser, a neuroscientist at University College London and an IBL investigator. Initially, the handful of labs that tested the task produced startlingly different results. A working group devoted to behavior issues went to work, creating a spreadsheet of roughly 50 different factors that could be contributing to variability — such as temperature, humidity, the animals’ age and the amount of protein in their food — and then spent six months standardizing those factors across the collaboration. “It really tested the basic model of IBL, of inter-lab experimental collaboration,” Häusser says.
At the first meeting of IBL’s scientific advisory board, Dulac remembers listening to the laundry list of possible variables with concern. But by the second meeting a year later, the problem had been solved. Indeed, the data was so consistent from lab to lab that an algorithm could not determine which group had generated it. “We were all stunned, in a good way,” Dulac says. “For me, this was the first time seeing on paper the ability of the IBL to solve an almost impossible challenge in a systematic way.” The results of those efforts were published in eLife in May 2021.
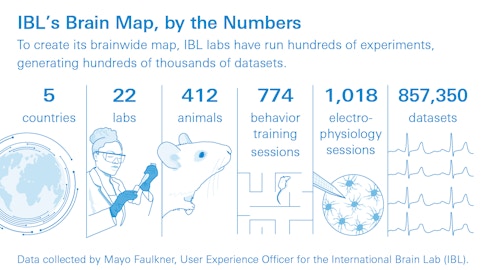
Successfully standardizing the decision-making task was just the first of many challenges IBL has to solve to be successful. As a collaboration of 22 different labs contributing to the same scientific goal, they’ve had to develop methods for sharing and analyzing large volumes of data. That’s no easy feat in a field with no standardized experimental language and little infrastructure for data sharing. And they’ve had to navigate the sociological challenges of team science, such as how to balance the needs of the collaboration and the individual, an issue of particular concern for trainees who are striving to make their mark on the field. None of these issues are new to neuroscience, but it’s only with the advent of IBL, which is funded by the Simons Collaboration on the Global Brain and the Wellcome Trust, and other large-scale projects that scientists have been forced to tackle them.
“It’s a bit of a culture revolution,” Dulac says. “It’s something that could really transform neuroscience.”
A distributed structure
Some fields of science, such as physics and genomics, have already made the move to big team efforts. But neuroscience has been slower to make the change. Most labs function largely independently, with the head of the lab acting as the primary decision-maker. Matteo Carandini, a neuroscientist at UCL and an IBL investigator, likens traditional neuroscience labs to a nautical system of captain and crew. IBL represents a substantial shift — it’s made up of a distributed network of labs that share resources and infrastructure. “No one is really captain,” Carandini says.
When developing their model, IBL’s founders took inspiration from CERN, the world’s largest particle physics lab, particularly its ATLAS project, which involves thousands of scientific contributors scattered around the world. “They really advocated this kind of self-organized, non-hierarchical model of running things,” says Zachary Mainen, a neuroscientist at the Champalimaud Center for the Unknown and an IBL investigator. “More than anything, it was proof there were models for doing science that worked in a completely different way than what we were used to.”
Large-scale, centralized efforts, such as the Allen Institute, offer an alternative model. But IBL aimed for a more grassroots organization. “We are trying to find a middle way between the top-down approach and the bottom-up approach of individual labs, which have lots of creativity but are disorganized, duplicative and unprofessional in positive and negative ways,” Carandini says. The National Institutes of Health’s U19 program, launched in 2017, also supports large neuroscience collaborations. But IBL is unusual in that member labs are running the same experiments and contributing to the same central goal, in addition to studying different aspects of a central question.
IBL’s governance model is inspired by the concept of sociocracy, a system based on consent for decision-making. Rather than requiring that all members agree to a proposal, sociocracy requires that no one object to it. With IBL, members can only object if a proposal could harm the collaboration. The approach has been useful for making project-wide decisions, Mainen says. “This process helps to make sure everyone has understood the proposal and gives a chance to improve it by addressing the concerns,” he says. “This helps make for better group decisions.”
Choosing IBL’s behavioral task offered an early test of the collaboration’s ability to compromise, with heated arguments over the most appropriate level of complexity of the task. “Theorists wanted a complex, cognitively challenging task so they could mine it for analysis in the richest way,” Häusser says. “Experimentalists wanted a simpler task because they knew that teaching mice a task is hard and reproducing it across a dozen labs is especially challenging.”
Researchers ultimately decided on a modified version of the two-alternative forced-choice decision-making task. Mice turn a wheel to indicate if they’ve seen a visual of a grating on their left or right side. The contrast of the grating changes over time, as does the probability of the grating appearing on one side or the other, forcing animals to use both vision and recent experience to make their decision. “It was challenging to agree on a task, but the final task is more interesting than the original choice,” says Jonathan Pillow, a theoretical neuroscientist at Princeton University and an IBL investigator. “It captures richer aspects of both behavior and neurophysiology that we could probe with theory.”
Having a voice
In many ways, IBL’s large collaborative structure offers a major cultural change in how labs relate to each other. “Instead of competing, we made it official to share our know-how,” Mainen says. “That’s a big win for everyone.” The close relationship among labs has a number of benefits. “I have learned a ton about how other people are doing things,” says Anne Churchland, a neuroscientist at the University of California, Los Angeles and an IBL investigator. “I think my whole lab has benefited.” And postdocs gain from more in-depth interactions with investigators beyond their advisers. “That’s been a really unique thing,” says Ilana Witten, a neuroscientist at Princeton and an IBL investigator. “Multiple established PIs know them, meet with them and teach them.”
Häusser notes that building a community where everyone trusts each other has been essential for collaboration among competitive labs. “We have lots of debates and disagreements, but it’s really about building consensus rather than proving the other guy wrong,” he says. “On the whole, the fact that the IBL is so visible, and everyone is in it to make science a better place and lead by example, has kept some of people’s worst instincts in check.”
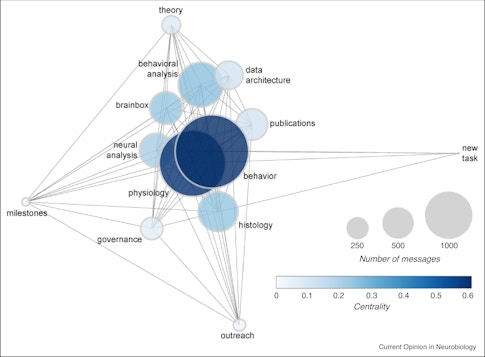
One of IBL’s core components is a system of working groups devoted to solving specific issues, such as how to deal with publications, reproducibility and trainee concerns. Members devote their time to issues that interest them, be it governance or technical challenges.
To make sure that researchers, including postdocs and graduate students, and staff, such as technicians and programmers, have a voice in IBL’s direction, leadership meetings include both the heads of the different working groups and representatives of staff and postdocs. “That’s really important because we noticed decisions were being made without sufficient consultation,” Häusser says. For example, early on, Olivier Winter, a programmer who came to IBL from the oil industry, wasn’t involved in leadership discussions of software and data analysis. “Including him in these discussions has been enormously helpful,” Häusser says. When researchers had issues properly synchronizing cameras with the behavioral apparatus, PIs did not prioritize the issue, Häusser recalls. Winter flagged it as a serious problem that was corrupting data.
The collaboration’s non-hierarchical structure also proved valuable in standardizing the task across labs, says Carandini, who chairs the behavior working group. “In this project, by far the most important voices were not correlated with rank,” he says. Technician Ines Laranjeira, now a graduate student, noticed that different labs fed their animals different diets, potentially contributing to differences in motivation. “She insisted on getting exactly the same food, and the problems we were having went away,” Carandini says. (He notes that they made a number of changes simultaneously.)
Baked into the organization of IBL is a deep connection between experimentalists and theorists. Half the teams are experimentalists and half are theorists, who are developing new tools for analyzing data and new models of decision-making. This balance has been essential to the project’s success. Involving theorists from the earliest stages, including selecting the task, has also been crucial, Häusser says. “Traditionally, theory is tacked on to the project rather than an integral part of it,” he says. “By involving theorists before the first kilobyte of data was collected, we structured everything so they get what they want.” A team of theorists and experimentalists is now preparing IBL’s first neurophysiology data paper. “The paper reflects the cohesive effort of the entire team,” says Hannah Bayer, IBL’s director, who is involved in strategic planning and coordination. “It’s not that the experimentalists did one section and theorists did the last figure; it’s much more integrated.”
Working closely with collaborators who think differently is valuable for trainees, who may carry that practice into their own labs, Dulac says. “Trainees will have an experience like nowhere else, where they are trained in an experimental task but also able to understand the reasoning of a theory colleague.”
Balancing the individual and the collective
One of IBL’s biggest sociological challenges has been to figure out how to balance the needs of the project as a whole versus the needs of individual contributors. For example, IBL’s central goal of creating a brainwide map of a decision-making behavior is an enormous, multistage effort that requires synthesizing data from different labs and brain regions. Along the way, the collaboration is producing large-scale platform papers, such as the eLife behavior paper, and general resources, such as a data architecture, that are already available to the broader community.
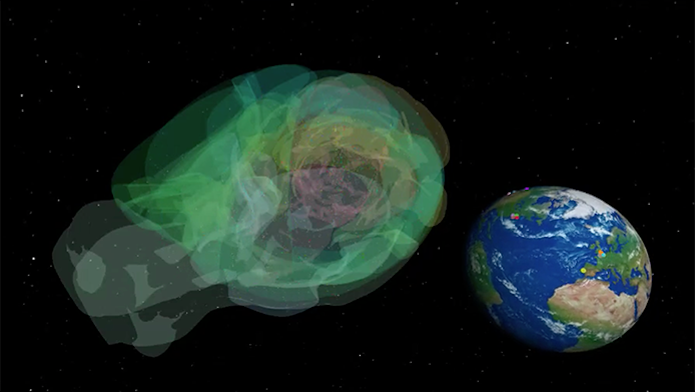
By clicking to watch this video, you agree to our privacy policy.
These projects have many more contributors than a typical neuroscience paper — IBL itself is the first author on platform papers — which presents a challenge to the field’s general model of publishing and credit. Postdocs on the job market often rely on first-author publications to highlight their potential for success as a future lab head. “Where you are on the author list and what symbols you have next to your name is the currency of academia,” Häusser says.
It’s unclear how the field will value individual contributions to platform papers. “I feel like we live in a half-changed world in which people recognize the need for team science but don’t yet fully appreciate individuals who contribute to such efforts,” Churchland says. The Allen Institute has made major contributions to neuroscience, as have big collaborations like the MICRONS project, she notes. But figuring out how to properly credit the people involved in these efforts lags behind the scientific advances themselves. “We don’t know how to recognize the collaborative nature of science,” she says. Faculty search committees, for example, still rely heavily on first-author papers. “It remains to be seen how much future employers will value big platform papers,” says Guido Meijer, a postdoc in the Mainen lab.
To address the issue, IBL launched a working group focused on publications and credit assignment. They developed a more fine-grained credit statement than is typical in the field, rating an individual author’s detailed contribution to many aspects of a project, such as conception, software, analysis and experiments. With some pressure from IBL, eLife changed their policy and published the credit matrix for the behavior platform paper.
In addition to working on platform projects, postdocs can develop a personal project, which can result in more traditional first-author papers. Though postdocs must split their time between the two efforts, their personal projects benefit from access to the collaboration’s infrastructure and data.
Though IBL postdocs may grapple with some challenges not present in traditional labs, they also benefit from more intensive networking. Meijer, for example, notes that he has weekly meetings with PIs he otherwise might never have met, which may help with future job searches. “If you did something important on a platform paper, you are known to 21 labs as someone who gets stuff done,” Carandini says. “You have made your name in a chunk of the systems neuroscience community.”
Witten says this is a pivotal time for IBL, as big team projects are wrapping up and researchers are transitioning to working more on individual projects. “I think the IBL is becoming a good model for balancing the individual and the team, and hopefully that will have a broader impact on the field,” she says. It’s too early to say how well this balance will work, and how IBL postdocs will fare on the job market — the pandemic’s effect on hiring makes this difficult to assess in the near term. But Bayer says the collaboration’s first few alums have gone on to faculty positions.
A broad model
IBL members hope their efforts to grapple with issues of collaboration, credit and infrastructure will have a broader impact on the field. Indeed, some organizations are already looking to IBL as a potential model for large-scale collaboration. “The International Brain Initiative is looking very closely at how we deal with conflict resolution, data-sharing and ethics,” Häusser says. “There are lots of problems we have had to solve that I think will benefit the field and beyond.” Other fields with replication challenges, such as computational psychiatry, have reached out to IBL for advice. And a proposal for a primate version of IBL is in the works.
Tools and other resources developed by IBL will also likely benefit the field, which is in desperate need of easy-to-use systems for data-sharing and analysis but has lacked incentives to create them. (For more, see “The Data-Sharing Problem in Neuroscience.”) To fill this void, the collaboration developed a number of new tools, including infrastructure for saving, sharing and analyzing data across teams, and software for visualizing large electrophysiology datasets, such as Neuropixels data. “We get requests for those all the time,” says Bayer, who notes that tools are available on the IBL website.
Churchland, who is part of IBL’s reproducibility working group, thinks the lessons IBL learned from standardizing behavior, electrophysiology and other factors will benefit the field more generally. Every IBL experiment includes an electrophysiological recording from the same ‘repeated’ site, enabling comparisons across labs. “We have a ton of data from each lab; it’s really unique in the world,” Churchland says. “We have learned so much about where variability comes from and how to address it.” For example, the group discovered that significant variability arises from even tiny differences in electrode placement. And though IBL members have eliminated most lab-to-lab variability, it still persists from experiment to experiment. “It comes down to biology — animals are different from each other,” Churchland says. “It means a lot of variability is at the biological level, which is intimidating but interesting.”
Tackling this variability is part of IBL’s next major challenge. Reproducible electrophysiology results across labs are essential for combining data into an interpretable brainwide map. So just as researchers had to standardize the behavior task across labs, they now have to standardize electrophysiological procedures. “To do this, we have to build a quantitative model of all the different factors — task-related, behavioral-related and everything else — that contribute to the variability in electrophysiological recordings,” Bayer says. “This really gets to the heart of the neuroscientific endeavor.”