
A New Era for the Neuroscience of Social Behavior
The COVID-19 pandemic has brought into stark relief the immense impact that social interactions have on our health, with people who have felt the most isolated reporting higher rates of mental health issues, substance abuse and health problems. Since the start of the pandemic, “it has become painfully clear that social isolation has really deleterious effects on people’s mood and their physical well-being,” says David Anderson, a neuroscientist at the California Institute of Technology and an investigator with the Simons Collaboration on the Global Brain (SCGB).
Despite our deeply social nature, our understanding of the neuroscience of social behaviors lags far behind that of other types of behavior. Research in flies, birds, rodents and other animals has uncovered both specialized social brain regions and brainwide support of social behaviors. But delving more deeply into the neural coding underlying these behaviors, which often involve multiple interacting animals, has been mired by technical limitations. Social settings make it particularly difficult to quantitatively measure behavior with sufficient precision, and relevant brain regions have been difficult to access for experimental observation. (For more, see “Q&A: New Tracking Techniques Reveal Unexpected Social Behaviors.”)
However, recent advances in tools for precisely monitoring behavior coupled with advances in neural imaging technologies and analysis methods are poised to revolutionize the field. On the behavior side, new methods that build on decades of progress in machine vision can track socially engaged animals’ movements in an automated way and with greater precision than ever before. “It’s now possible to track social behavior at even higher resolution and to keep track of animal identities over long recordings, allowing us to study the subtleties of social interaction in a way that was not as feasible before,” says Mala Murthy, a Princeton University neuroscientist and SCGB investigator.
On the neural side, neuroscientists are now pairing automated behavioral tracking with technologies for recording large populations of neurons and for silencing specific neurons to study the neural basis of social encounters with a level of precision that has not previously been possible. “Putting these two advances together gives us enormous power in uncovering the mechanisms that shape social interactions, arguably the most complex behaviors that animals produce,” Murthy says. Additionally, social behavior neuroscientists are beginning to employ analysis tools, historically used in cortical areas, that can consider hundreds to thousands of neurons recorded simultaneously, leading to a reevaluation of how social brain regions guide behavior.

“Social behavior is really becoming this playground, thanks to the convergence of all of these different types of methods that have become available recently,” says Lindsay Willmore, a former doctoral student at Princeton who is now at DeepMind. “Pick your favorite social brain region, pick your favorite behavior, and all of a sudden you’re able to combine them in whatever way you want.”
Balancing behavior
Neuroscientists design behavioral experiments balancing two needs: Behavior needs to be controlled enough to be quantitatively described and tied to relevant neural activity, but not so tightly controlled that it ceases to resemble ‘normal’ behavior. The appropriate limit has evolved over time and with technological advances. For example, classic primate experiments studying vision, dating to the middle of the last century, anesthetized animals to reduce behavioral variation. But with time, researchers became concerned that anesthetized brains might not work like awake brains, and new technologies for monitoring behavioral variability reduced the need for such aggressive control. Neuroscientists continue to grapple with how constrained behavior should be, and how emerging technologies can provide increasingly precise quantitative documentation of increasingly unconstrained behavior.
Over the past five to 10 years, a wellspring of new methods for automated behavior tracking has made it easier to study natural social behaviors. (For more, see “Taming the Data From Freely Moving Animals.”) These new approaches, fueled by advances in computer vision and machine learning, can quickly translate hours of raw video of behaving animals into streams of data ready for analysis, such as the precise position of an animal’s head or legs, or even sequences or libraries of inferred behavioral ‘motifs.’ A process that once required days or weeks of painstaking human work can now be completed in minutes or hours.
These methods were first developed for solitary animals, and applying them to social behavior has been no easy feat. “It’s a much harder problem than analyzing behavior of solitary animals,” Anderson says. Researchers had to find ways to correctly group the identified body parts and to keep track of each animal over multiple video frames. “Say you found the location of all the heads, all the tails, and all the left legs within a single image,” says Talmo Pereira, a neuroscientist at the Salk Institute. “If we’re going to determine what social behavior is occurring, it’s essential we know whose head belongs to whose tail.”
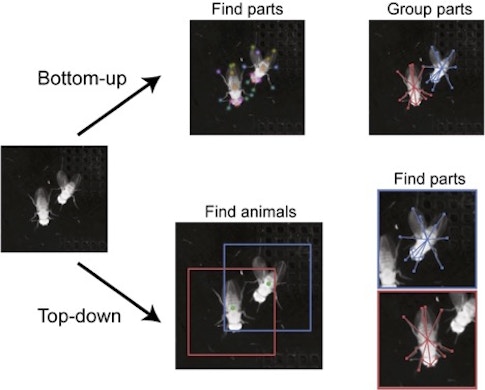
Several prominent methods have emerged over the last year, including SLEAP, developed by Pereira while a graduate student in Murthy’s lab at Princeton, and a ‘multi-animal’ extension of DeepLabCut, a popular method originally developed for individual animals by neuroscientists Mackenzie Mathis and Alexander Mathis of EPFL in Switzerland, both published in Nature Methods in April. The different methods adopt different approaches for tackling these challenges. DeepLabCut’s multi-animal extension, for example, favors a primarily ‘bottom-up’ approach to grouping body parts — finding the parts and then grouping them — while SLEAP takes a more agnostic view, guiding users about whether a bottom-up or top-down approach —finding each animal and then its parts — is likely to work best depending on their particular use cases.
A road map for combining methods
Neuroscientists are now applying these methods to study the neural basis of social behavior with a level of precision unimaginable even a decade ago. In research published in Nature in October, Willmore, together with Annegret Falkner and Ilana Witten, both SCGB investigators at Princeton, combined DeepLabCut with existing technologies for recording and activating neural activity to examine why some mice become depressed or anxious following a stressful experience — being aggressively bullied by a larger mouse — while others do not. To understand the behavioral and neural differences between these two groups, researchers had to quantitatively measure the moment-by-moment behaviors each mouse expressed, such as attacking and fleeing, when they were engaged in a stressful social interaction, as well as observe and control neural responses with temporal precision.
“This study stands out in successfully merging these cutting-edge techniques and doing so in a way that enables temporal precision with regard to linking neural activity and behavioral dynamics,” says Murthy. “It provides a road map for others who will no doubt want to take a similar approach in mammals in dissecting other complex behaviors.”
The researchers found that the resilient animals — those that did not become depressed — tended to adopt defensive postures and fought back against the aggressor, whereas the susceptible animals tended to run away more often and avoid the aggressor. “One of the behaviors that pops out as being really interesting is a defensive posture, which suggests that the resilient animals defend themselves a little bit better,” says Falkner. To identify these subtle behaviors from their precise tracking data, they used an unsupervised machine learning approach, a unique method for letting the algorithm find patterns in the data. Pinpointing these behaviors so precisely would not have been possible without automated behavioral tracking, says Willmore, “because the previous state of the art is just people eyeballing what they think the animal is doing.”

To examine the neural basis for these differences, researchers focused on the dopaminergic system, long known to play a role in reward-based learning. “We thought there might be some kind of reward-threat balance with the dopamine teaching signals, telling the susceptible versus resilient mice to balance their avoidance versus approach to the aggressor,” says Willmore. They found that resilient animals showed a sustained dopamine response when they fought back against the aggressor, suggesting they found the act of fighting back, or perhaps the stress of the encounter itself, rewarding. Susceptible animals, in contrast, showed decreased dopamine response when being attacked or when close to the bully and a large dopamine response at the end of attack periods, indicating they found the end of the stressful experience rewarding.
“If you are thinking about real-world consequences of this, what this suggests is that we need to incorporate more rewards during stressful experiences, and not just at the end of stressful experiences,” Falkner says.
Perhaps most exciting, Falkner, Witten and collaborators coupled automated behavior tracking and neural control, activating neurons during specific behaviors, like fighting back or fleeing from the bully. The goal was to enhance resilience in mice on the losing side of the battle by stimulating their dopamine terminals when they fought back, says Willmore. Incredibly, the researchers found that this worked: Stimulating dopamine neurons when the animals fought back led them to be more resilient — they showed less depressive or anxious behaviors following the encounter. However, control mice that received the same amount of stimulation during the stressful encounters but not at the moments when they fought back were also more resilient, suggesting that the precise timing of the dopamine signal may not be critical. “Our hypothesis is that increasing the rewarding component of stress is sufficient to increase resilience even if you don’t necessarily increase the incidence of this one specific, resilient-style behavior,” says Willmore.
Falkner and Witten’s team now plans to extend this approach, simultaneously stimulating and recording from multiple brain regions in the so-called social behavior neural network, a collection of subcortical brain regions governing social behaviors, while automatically tracking social behaviors. In addition to closed-loop experiments based on behavior, “we’re really interested in doing closed-loop experiments based on patterns of neural activity, as well as closed-loop experiments based on behavior and then writing patterns of activity back down to these brain regions,” Falkner says.
Analyzing aggression
Studying social behavior isn’t challenging simply because of the complexity of behavior. The brain circuits that govern social interactions and the analysis tools used to study them present their own challenges. Key social brain regions in mammals, for example, are very deep in the brain. The ventromedial hypothalamus, an important node in the social behavior brain network, “is pretty much located at the floor of the brain just above the roof of the mouth,” says Anderson. “And that means that it’s very invasive to stick an electrode bundle or an optic fiber down through the top of the brain.” As researchers chip away at this challenge, a view of the mammal social brain is emerging.
Sociological factors have also impacted the development of the field. “The systems neuroscience community has pretty much ignored the hypothalamus,” says Anderson. “The idea that there is complex and sophisticated circuitry in there that might actually carry out some computations is not something that has interested or attracted the attention of most systems and computational neuroscientists, simply because they assumed there wouldn’t be anything interesting to discover in that region.”
The standard approach for analyzing neural responses from social behavior circuits in mammals — long the standard throughout neuroscience — is to examine the response of each neuron individually, “what I call the ‘pie chart’ approach,” Anderson says. “Researchers go neuron by neuron, and they look to see under what conditions — when the animal is engaged in a particular behavior or interacting with a particular sex — the neuron responds.” But just as in other brain regions, that approach has limitations in social regions. For example, Anderson and his former postdoc Ann Kennedy have previously found that although stimulating the ventromedial hypothalamus can reliably elicit aggression, individual neural responses only rarely reflect these behaviors.
Analyzing neurons as a population rather than as many individual cells offers an alternative. In the cortex, this approach has sparked a revolution in the theory of how neurons produce movement. (For more, see “A Look Back: The Great Debate Over How the Motor Cortex Produces Movement.”) New results from Kennedy and Anderson, posted on the bioRxiv in April, suggest that such methods may transform the interpretation of the social behavior network as well.
The researchers showed that in the ventromedial hypothalamus, population activity as a whole better reflected aggressive behavior than the activity of individual neurons did. Population activity collectively formed a line attractor — a common computational motif in studies of cognition — and neural activity unfolding over time along the line attractor corresponded to the animal’s increasing propensity to behave aggressively. (For more, see “New Insight Into the Hidden States of the Brain.”) In recognition of her research, Kennedy, now a neuroscientist at Northwestern University, won the 2022 Eppendorf and Science Prize.
A social future
Despite these exciting findings, key questions about the neuroscience of social behavior remain. For example, many of the same challenges that plague the use of new experimental techniques in other areas of neuroscience, issues as pedestrian as lack of computing resources or software that’s difficult to install, also stifle adoption of automated behavioral tracking approaches. “It’s these little practical problems that challenge users,” Pereira says. “I routinely find that people get more hung up on opening a terminal,” a core application for issuing low-level computer commands, “than they do training a neural network.” Computer graphical user interfaces that are easy to use will be key to overcoming these hurdles.
Adopting population-based frameworks may also prove a challenging shift for the field, because researchers studying social behaviors have traditionally focused on individual cells and their unique biological features, details that population analyses often overlook. “Neurons in the hypothalamus aren’t just a uniform blob,” Kennedy says. “You have so many different cell types, communicating with each other with so many different peptides and signaling molecules.” Because of this heterogeneity, some researchers have doubts about applying population-level analyses to recordings in social behavior circuits, Kennedy says. “People don’t like stepping away from what the individual cells are doing. Each cell’s response is a function of its gene expression and connectivity, and you throw all that out the window when you look at population coding.” As the field of social behavior balances these considerations, it may encourage cortical researchers to reconsider these questions as well. (For more on cell types, see “Crossing the Divide: Defining Neurons by Both Genes and Function.”)
Despite these challenges, the field is clearly exploding. Researchers are now applying automated behavioral tracking methods to study different types of social behaviors, often in conjunction with neural recordings. At this year’s Society for Neuroscience conference in November, researchers presented work showing how SLEAP-based behavioral tracking can be used to understand the neural representation of social isolation, the effects of hormones on neural activity during social behaviors, and the neural mechanisms of fly courtship, to name a few.
As the field matures, it will offer an exciting counterpoint to classic assumptions derived from other brain regions and behaviors. For example, line attractors, a classic model in cognition and memory, have predominantly been modeled as having been formed solely through recurrent synaptic connections. Study of the hypothalamus, in contrast, has focused on other chemical processes in the brain, notably neuropeptide signaling. The discovery of line attractors in the hypothalamus provides the chance to test the role of recurrence versus neuromodulation. “We don’t know how much of the dynamical properties of the neurons that contribute to the line attractor are determined by local recurrent, synaptic connectivity versus controlled by slow acting neuromodulators,” says Anderson.