
Big Data and Powerful Computing Join Forces to Link DNA Sequence and Function
The launch of the Human Genome Project in 1990 was accompanied by the hope that, when completed, the resulting full human genome sequence would help build a Rosetta stone of sorts. A way to translate — or link — differences among humans to changes in the genes themselves.
As the project progressed, however, that hope diminished. The newly decoded genome revealed that just a tiny part of it — about 1 percent — was actually made up of genes. The remaining 99 percent was designated as noncoding regions, which soon became the new focus of genomics researchers. Scientists soon learned that the noncoding parts of the genome, once derided as ‘junk DNA,’ are rich with the potential to influence the genome’s coding region in important ways, even if they are devoid of genes themselves. But drawing an unequivocal line between a particular DNA sequence and the resulting trait or disease has proved a herculean task.
“This is one of the most fundamental questions in biology, yet also one that is extremely challenging to address on a whole-genome scale while taking into account human diversity,” says Olga Troyanskaya, deputy director for genomics at the Center for Computational Biology (CCB) at the Flatiron Institute and a professor of computer science and member of the Lewis-Sigler Institute for Integrative Genomics at Princeton University.
In recent years, epigenetics — the term for the regulatory mechanism that alters a chromosome without changing its underlying DNA sequence — has emerged as a promising factor that could link DNA variation with function. Indeed, epigenetic mechanisms have been found to underlie an increasing number of health conditions, from cancer to the effects of aging to infertility.
By harnessing the breadth of epigenetic data now available to the scientific community, Troyanskaya and her team have created a predictive computational model that might just bring forth that long-awaited Rosetta stone. Called Sei (pronounced ‘say’), after a species of baleen whales, the model is a major leap forward in breadth and accuracy compared to prior models based on predictive DNA sequences. It was reported by Troyanskaya and collaborators in Nature Genetics in July of 2022.
“Sei can distinguish between DNA variations that are functionally important at the molecular level at an unprecedented scale,” says Chandra Theesfeld, a research scientist in Troyanskaya’s lab. Though the platform is in its first months, Sei is already narrowing the gap between knowing the DNA sequence and knowing what that sequence actually does.
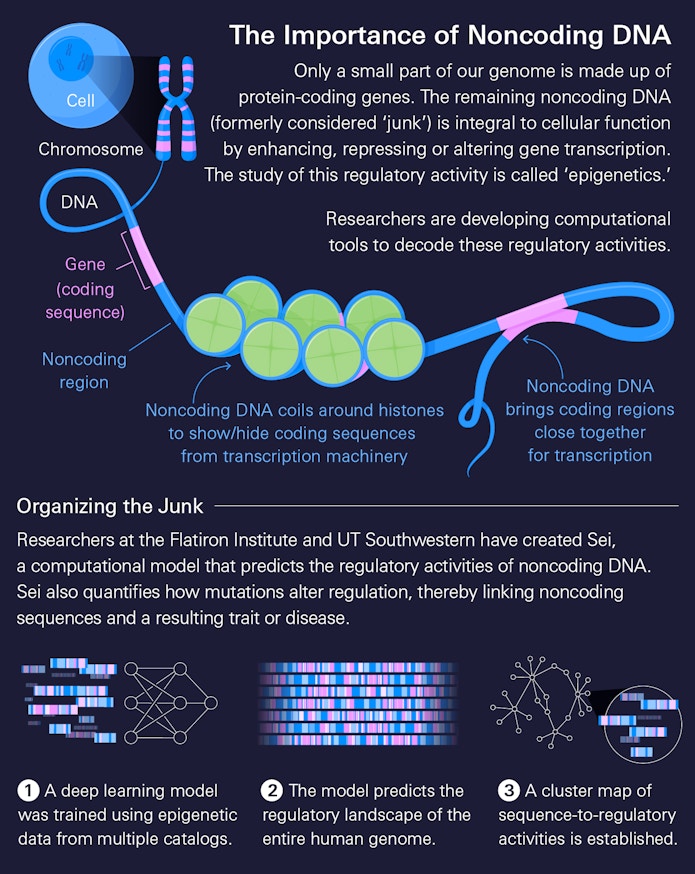
Big data form the foundation for Sei
Before Sei could make predictions on how noncoding DNA sequences regulate genes, it first had to study real-life epigenetics datasets. Such datasets, collected and curated from previously published research, effectively function as a dictionary of individual DNA sequences and their corresponding regulatory activity. Because DNA sequencing has become fast and cheap, epigenetic data abound at a scale not previously seen. In fact, the existence of these enormous data repositories helped to inspire Sei in the first place. “We thought: Can we leverage the sheer amount of publicly available data to interpret genome-wide epigenetic regulation?” says Kathy Chen, a Ph.D. student in Troyanskaya’s lab, a visiting scholar at the Flatiron Institute and first author of the Nature Genetics paper.
The research team trained Sei by feeding it data from a catalog of epigenetic profiles that list the location and types of epigenetic characteristics associated with a particular DNA sequence. In total, these profiles number 21,907, the largest to date, from over 1,300 cell lines and tissues, and cover the entire human genome. The data were collected and processed by large-scale consortiums like the Cistrome Project, Roadmap Epigenomics and ENCODE that use experimental assays to determine the epigenetic information. If Sei were learning to read, these data would represent the first words the model would learn.
Once Sei learned the massive epigenetic dictionary, the researchers then applied the model to the entire human genome reference sequence. The researchers wanted Sei to be more than a big data version of DeepSEA, built in 2015 from just under 1,000 epigenomic profiles and one of the first deep learning-based sequence models to accurately characterize the regulatory impact of DNA sequences. “With Sei, we wanted to summarize the data in this huge catalog to make a global map of integrated molecular activity,” says Jian Zhou, who completed his Ph.D. in Troyanskaya’s lab and is now an assistant professor of bioinformatics at the University of Texas Southwestern Medical Center and one of the Sei paper’s lead authors.
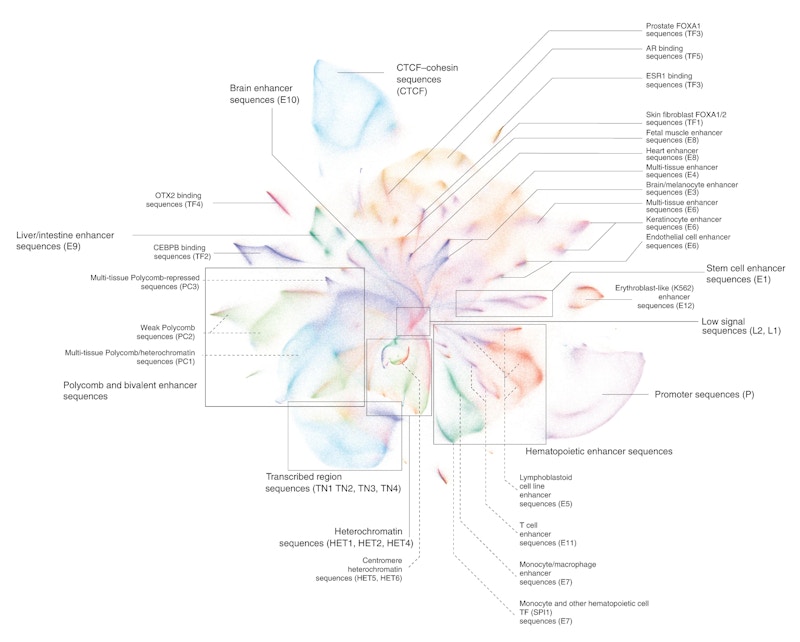
Sei’s predictions of genomic regulatory activity fan out into a map that can identify the functional impact of any DNA sequence that a scientist feeds into it. The labels on the map represent groups of DNA sequences that Sei predicts will exert similar regulatory activity, and which are therefore clustered together in ‘sequence classes.’ Importantly, the sequence classes were determined by data clustering methods, rather than by scientists who would first define them and then fit sequences into them. “We wanted the data to guide us, rather than the other way around,” says Chen. Sei assigns a particular DNA sequence to a sequence class, and thus predicts what kind of regulatory activity that sequence causes. The map shows the tissue where the regulatory activity occurs and indicates whether that activity turns a gene up or down, resulting in more or less protein. “We wanted to provide both a global interpretation and also tissue-specific regulatory function predictions,” says Zhou. With this map, scientists can see if, where and how a particular DNA sequence affects a gene, and thus the protein it codes for, and they can do this on a whole-genome scale.
Predicting the regulatory activity behind human traits and disease
With the global map anchored by sequence classes, Troyanskaya and her team set out to test Sei on human datasets, starting with the U.K. Biobank, a repository of genetic and health information assembled from a half-million U.K. participants. Sei examined the Biobank’s full set of genome-wide human DNA variants associated with traits and diseases. Some of these variants are associated with traits linked to disease risk, such as cholesterol levels and blood pressure. Others are more typically associated with life circumstances, like years of college completed, or whether someone is a morning person. All the traits have at least some degree of heritability, meaning that they can be influenced by genetic variation rather than just the external environment. Because Sei’s sequence classes do not overlap with one another, each variant was assigned one sequence class in the prediction. “This allows for the breakdown of heritability [of a trait associated with multiple variants] into components contributed by different sequence classes, providing a clear picture of the regulatory architecture of the trait in a way that hasn’t been done before,” says Chen.
Some groups of traits lit up sequence classes associated with the expected tissue. For example, variants connected to blood-related traits were assigned to sequence classes for those cell types. Similarly, variants associated with traits like years of college education fell into sequence classes associated with enhancer activity in the brain and stem cells.
While many of Sei’s predictions robustly validated what researchers already know about these traits, the model also predicted 83 new associations between traits and regulatory activity. Some of these new associations were particularly informative; for example, hypothyroidism was linked to enhancer activity in the immune system’s B cells and T cells. Waist-to-hip ratio, which doctors use as a risk factor for cardiovascular disease and Type 2 diabetes, was linked to enhancer activity across multiple tissues, suggesting that the epigenetic activity isn’t localized within just one tissue. These predictions can help inform follow-up experiments that would test these putative causal variant-trait relationships.
Sei has also proved particularly effective at elucidating disease-specific traits. “In many instances, scientists already know that a disease mutation is near a gene known to be relevant to the disease,” says Chen. “But whether the gene’s regulation is actually disrupted, and how, is something Sei can illuminate.” When the researchers used Sei on 853 regulatory disease mutations from the Human Gene Mutation Database, they found that many cell-specific disease mutations were predicted to affect enhancer activity in those cell types, pointing to true gene disruption. For example, a mutation causing vitamin K-dependent protein C deficiency, a disease involving the liver, was predicted to decrease enhancer activity in the liver genes. “Sei provides possible regulatory mechanisms for disease mutations that have been identified in previous studies,” says Troyanskaya.
Since many diseases are attributed to the loss of protein function (which would be seen here as a decrease in regulatory activity) it was surprising to see that a full 20 percent of the mutations were predicted by Sei to increase regulatory activity, says Zhou. Some of these predictions involve a class of proteins called CTCF-cohesins that bend DNA into loops, bringing certain stretches of DNA close together for short periods of time with significant epigenetic effects. This result highlighted the important role CTCF-cohesins may play in disease.
These and other examples are already revealing that Sei can give a definitive answer to the question, “Does this mutation affect the protein in question?” at a whole-genome scale and can suggest avenues for scientists to explore further. “From here, clinicians can go on to test individual mechanisms and see if they are consistent with what they see in patients,” says Troyanskaya.
Verifying an evolutionary mechanism for the human-chimpanzee split
In August 2021, Sean Whalen, a research scientist at the University of California, San Francisco, noticed a tweet linking to the Sei preprint. Reading the paper made Whalen, a member of Katie Pollard’s lab, wonder whether Sei could be applied to his own research on human evolutionary genetics. Pollard, a professor of epidemiology and biostatistics at UCSF, director of the Gladstone Institute of Data Science and Biotechnology and a Flatiron Institute IDEA Scholar, brought the paper to her lab’s journal club to discuss Whalen’s idea. The Pollard lab is studying regions of the human genome that show signs of accelerated evolution. Genetic changes in these so-called human accelerated regions (HARs) are well known for having pushed humans down their own evolutionary path, away from that of chimpanzees, about 7 million years ago. Interestingly, recent research has shown that HARs may also play a key role in developmental and psychiatric conditions like autism and schizophrenia, presenting a complicated puzzle of neurological and evolutionary changes.
“In our experiments, we could test just a limited number of differences between humans and chimps,” says Pollard. “Sei could look at all of the differences. So we thought, let’s run it and see what it says about regulatory activity.”
The group’s experimental assays suggested a mechanism at work called compensatory evolution. A sort of ‘evolutionary backtracking,’ compensatory evolution occurs when some mutations have an effect that is opposite to the effect of others. “Perhaps at one point there were too many differences, and then evolution shifted things back,” says Pollard. “Why? Maybe the environment was changing, or maybe a new biological process evolved that turned out not to be favorable and needed to be corrected.”
In essence, Sei confirmed the team’s experimental results at a larger scale. Most of the HAR-containing variants increased enhancer activity, with other variants decreasing that activity. “Sei ended up giving us the comfort to believe in the experimental data,” says Pollard. “The results suggest that evolutionary changes may have gone too far from the human-chimp ancestor, and needed to be brought back.”
Bringing the best of the Flatiron computing power to the scientific community
Zhou was behind the naming of earlier machine learning models based on DNA sequences, like Beluga, a precursor to Sei, and Orca, which he developed in his lab at the University of Texas and which makes predictions about the 3D structure of DNA. But Sei is the model that most closely matches its namesake, one of the fastest and biggest species of whales, in power and scale.
At the Flatiron Institute, Chen worked closely with Zhou to design a computational architecture that could handle data at this scale and process it in a reasonable amount of time. This careful design inspired Aaron Wong, a data scientist and interim lead of informatics at the Simons Foundation, to create the HumanBase Sei web server, enabling researchers to run Sei on their own sequences. “If we hadn’t been at the Flatiron, with its computing team and resources, our work couldn’t have progressed nearly as quickly,” says Chen. Just as important, says Wong, who leads the development of the HumanBase platform, is the way the benefits of the Flatiron’s computing resources radiate out to users of Sei. “The Sei web application makes the Flatiron’s immense computing resources freely available to users,” he says. “They get results back quickly after they submit DNA sequences and can explore predictions through interactive visualizations.”
For Sei users outside the Flatiron Institute, the experience has been smooth. “Sei was very easy to use,” says Whalen. “Running it was straightforward, and interpreting the outputs was intuitive.” Whalen did end up needing a modification to Sei that would allow the model to consider multiple chimpanzee variants at a time. When Pollard mentioned this to the Sei team, they quickly added the needed functionality. “I appreciate how responsive the developers were,” says Pollard. “They really collaborate with users.”
While a big data approach is necessary for any kind of machine learning, the computing muscle that underlies Sei plays an equally important role. “The huge size of the data that went into Sei and the massive computing power at the Flatiron Institute came together to maximize the pattern-finding capabilities of deep learning,” Theesfeld says.
“Sei helps us answer questions about mutations and the nature of DNA variation and disease in a way we couldn’t before,” adds Troyanskaya. “And because it’s available through the HumanBase platform, it enables the greater scientific community to answer these questions as well.”
With Sei so new, excitement abounds over the mysteries of human biology that Sei can shed light on. In addition to probing the functional impact of known mutations, the model can make predictions for simulated mutations, further refining our understanding of biological cause and effect. Theesfeld envisions Sei helping to create genetics-based clinical treatment flowcharts for diseases we know little about, similar to those that currently exist for breast cancer. “We probably haven’t thought about all the different ways to use this,” she says. “Through ongoing collaborations we are applying Sei to medical genomes and only just beginning to unlock its potential.”