
Geometrical Thinking Offers a Window Into Computation
If you spent 2020 the same way I did — housebound, with an abundance of free time — perhaps you also directed your attention to the following critical but boring task: organizing your clothes. Imagine you have a two-drawer dresser, and you need to sort four types of garments: sweaters and T-shirts, either of which can be V-neck or crew neck. What’s the best way to organize them? Placing sweaters and T-shirts in separate but adjacent drawers keeps them organized and easy to find, and also reflects a natural relationship between them — they are clothing tops for different weather conditions.
When processing information, your brain faces a virtually identical problem. How should it group the information it needs so that important shared qualities are represented while key individual details are preserved? Two new lines of research by investigators with the Simons Collaboration on the Global Brain (SCGB) have tidied up our messy understanding of this problem. To do so, the researchers used a powerful set of tools based on geometry. A key method of maintaining an orderly dresser is to keep similar things close but not so close that they get mixed up. The same is true for the brain. This is why geometry — the mathematics of distances and shapes — is so helpful: It’s fundamentally about correctly defining and preserving relative distances between similar things.
“We are going beyond what people have been doing until now, which is to say whether there is any information about the task-relevant variables in the neural activity in a particular brain area,” says Stefano Fusi, a neuroscientist at Columbia University’s Mortimer B. Zuckerman Mind Brain Behavior Institute and one of the SCGB investigators working on this problem. “We really care about the format in which this information is represented at the neural population level.”
The first branch of work, recently published in Cell, uses geometrical analysis to explain how the brain simultaneously constructs two equally important things — abstract concepts (like seasonal appropriateness) and key details (like V-neck or crew neck) — to help it make decisions. The second branch, recently published in Neuron, applies similar analysis tools to understand how the brain performs a very different computation, but one where keeping information in order is just as critical — keeping track of what to do next when performing a complex movement. Both studies add to a growing body of literature that uses geometrical analysis as a window into the mechanisms the brain employs to enable intelligent behavior.
Although geometrical thinking has been used before, these two new studies have vaulted the framework to new heights. Mark Churchland, also a neuroscientist at Columbia’s Zuckerman Institute, who is spearheading the second line of research, thinks that it may be the language we so desperately need for describing the mental computations that underlie cognition and movement.
“If geometry turns out to be a natural language for discussing many computations performed by the brain, that would be extremely convenient because we have 2,000 years’ worth of tools for understanding the implications of geometry,” says Churchland, relaying a point made by his Columbia colleague Larry Abbott, a theoretical neuroscientist and SCGB investigator. “The difference between two computations is sometimes really obvious when you plot the geometry of the data.”
Shapes in the brain
In collaboration with Daniel Salzman, also a neuroscientist at Columbia’s Zuckerman Institute and an SCGB investigator, Fusi focused on understanding how neural representations critical for cognitive thought can support abstract concepts without sacrificing key details. To study this, the researchers designed a simple association task in which animals had to learn a set of rules that depended on context. For example, in one context they were rewarded for pressing a button when they saw a red pattern, while in the other they were rewarded for pressing the button when they saw a blue pattern. Although each specific rule within a context — see red, press the button, get a reward — was unique, the collection of all four rules within a single context had a common feature, namely their shared context. Likewise, the collection of rules that led to receiving a reward in either context — like pressing a button for a red pattern in one context, and for blue in the other — had a common feature, that they led to a reward.
Silvia Bernardi, a neuroscientist at Columbia, recorded the neural activity of monkeys while they performed this task, and Fusi and his team of theorists examined the neural activity to look for signatures of abstract thinking. In particular, they looked at the geometry of the representation, which considers the organization of the collective activity of many neurons for each unique rule in the task. “It’s more important to look at the geometry of the representations, rather than individual response properties of the neurons, because there are a lot of things that you can see only at the neural population level,” says Fusi.
To understand the geometry of the representation, the researchers visualized the neural activity as a set of points in a space, where each point represented the activity of the neurons for each unique condition. For example, one point represented the neural activity for ‘see the red pattern, press the button, get a reward,’ while another point would be a different combination. Their central question harks back to the task of organizing clothes: How are the points arranged so as to organize related information but not mix everything up?
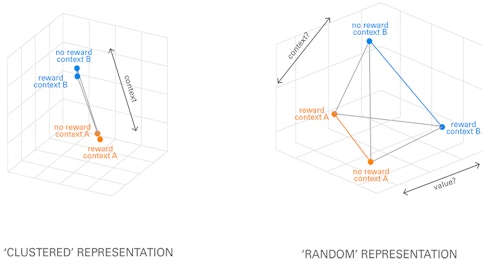
To see why this is a challenge for the brain, the researchers first considered two extreme, idealized formats: a ‘clustered’ format, where abstract concepts are favored even if information is lost, and a ‘random’ format, where all the details are retained, even if abstract concepts suffer.
A clustered representation is highly ordered, with an axis that defines a key feature of the organization, in this case what context a trial belongs to. Points sharing a context are close, and different contexts are distant, making it easy to distinguish them, but key details — was the trial rewarded or not? — get mixed up. Clustered representations are also called low-dimensional because all the points are contained within a small volume. A random representation, on the other hand, is highly disordered and spread out, with every point occupying an uncoordinated location in space. Because every point has its own spot, the chances of mixing things up is low, but this format lacks abstract concepts because the disordered placement of the points means there is no convention followed by all the components of a concept. If the monkey used this format, it would have no mental concept of context or value, but instead would just take each trial as it came without recognizing the relationship some trials share. This type of format is an example of a high-dimensional representation, because the points occupy much of the space in which they reside.
When Fusi and colleagues looked at the neural activity, they were surprised to see that it both extended upon and combined features of the two extreme cases they considered. When they plotted data from the monkeys’ prefrontal cortex and hippocampus, two brain regions central for performing this task, highly ordered shapes like cubes and squares emerged, a natural extension of a clustered representation. Just as context was ordered along a single line in the clustered format, here two variables — context and value — were ordered along two perpendicular directions to form a square, creating two abstract concepts simultaneously.

But they also found that these shapes were slightly distorted in key ways, further increasing the dimensionality of the shapes, just like the spread-out random representation. Although the square structure was preserved, they found that each point was a little further spread out in other directions, further increasing the brain’s ability to categorize things related to the task, but not at the expense of the orderly structure it had built to form abstract concepts.
“It’s a very nice compromise because on the one hand, you have the nice generalization properties of the low-dimensional structures, but at the same time, you also have the flexibility of the high-dimensional neural representation,” says Fusi.
In both brain regions, the ordered geometry the researchers found meant that the brain did construct abstract concepts even though these concepts were not explicitly defined for the animal. Furthermore, this rigid structure, in conjunction with the mild distortions superimposed on top of it, made the representations extremely detailed — each individual point, although ordered, was spread out enough that it could reliably be distinguished from all the others. This would be like further organizing your T-shirt and sweater drawers by not only putting V-necks on the left and crew necks on the right, but also pushing some items forward and some to the back; doing so would create a second degree of order beyond the separate drawers themselves, while also creating a little more space for other items you might acquire in the future.
These findings strongly suggest that these key brain regions achieve the critical but delicate balance between encoding details and forming abstract concepts that cognition requires. The findings may also shed light on neuropsychiatric disorders. “A lot of psychopathology is actually an impairment in the ability to abstract and to generalize,” says Bernardi, who is also a psychiatrist. “Patients that present with problems that look like they are emotional, like borderline personality disorder, really have difficulty generalizing a set of circumstances to other instances.”
The geometry of trajectories
A key advance in Fusi and Salzman’s approach was considering the geometry of the neural representation to draw conclusions about the computational properties inherent in that representation. Churchland, an expert in the motor system and an SCGB investigator, has sought to extend these geometrical insights to neural population trajectories — neural activity that evolves over time — to understand the key computational principles underlying movement.
The basic problem Churchland wanted to tackle was the following: When we perform a movement that is constructed from multiple repeated parts, like rotating a bicycle pedal, how do we ‘know’ what specific part we are performing at any moment? Have we rotated the pedal three times, or four times? To answer this question, Churchland and Abigail Russo, who recently completed her doctorate in his lab and is now a postdoctoral researcher at the Princeton Neuroscience Institute, analyzed the neural activity of monkeys as they rotated a hand-held pedal (in much the same way as we pedal a bicycle) while navigating a virtual environment, like a video game. Critically, the task wasn’t a joyride for the monkey to pedal through as it wished — it had to pedal for a certain number of cycles, say seven, and then stop.
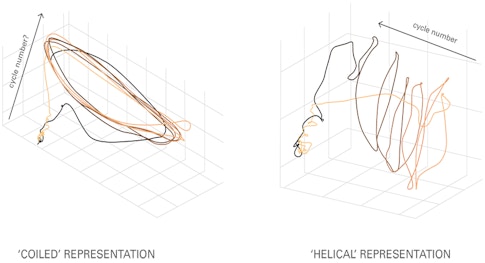
“He keeps track of where he is because he needs to know when he’s going to stop,” says Churchland. “And he needs to know when he’s going to stop before he stops because the pattern of muscle activity that you need to stop is very different from the pattern of activity that normally moves the hand. So somewhere you need to be able to know, ‘Am I on pedal cycle 2 of 7, or 6 of 7?’ Otherwise, how could you possibly generate the right pattern of activity to stop?”
To understand how the brain does this, the research team recorded the animal’s neural activity in two motor-related brain regions: the supplementary motor area (SMA) and the primary motor cortex (M1). Instead of looking at static points, as Fusi had, they plotted the activity over time, corresponding to periods when the animal was pedaling for multiple cycles. Visualization provided a striking distinction between the two brain regions. Activity in M1 created a circle over the course of a single pedal rotation but looped back onto itself, like a coiled rope, for each rotation. While activity in the SMA also traced out circles for a single rotation, it continued to evolve as the animal cycled, forming a helix.
Using this visualization, Churchland hypothesized that the SMA is the key brain region for keeping track of cycle number. Because each cycle of a helix occupies a unique volume in space, each loop could be used to demarcate a unique cycle. “You still have the information about where you are in the moment, but you’ve added this additional source of information, and now all of a sudden cycle 2 of 7 and cycle 6 of 7 are in very different parts of state space,” says Churchland. State space refers to the geometrical coordinate system the researchers used to examine the neural responses. In contrast, the geometry of M1, which traces the same path over multiple cycles, couldn’t possibly be used to distinguish different cycles. “Motor cortex knows what pattern of muscle activity you’re generating in the moment. But it cannot tell you whether you’re on cycle 2 of 7 or 6 of 7. It just has no idea,” says Churchland.
Geometrical thinking makes what once seemed confusing almost painfully obvious: The most natural solution to keeping things organized, even time itself, is to simply to spread them out. Incredibly, using similar analyses on neural recordings from very different brain regions from animals performing very different tasks, Churchland identified a principle eerily similar to that found by Fusi: By carefully organizing its activity the brain can retain the moment-by-moment details while simultaneously keeping track of the big picture.
A universal language of computation
As technologies progress for observing more of the brain in greater detail, a key challenge will be the development of a common mathematical language to simplify and unite disparate but related sources of data. Many scientists think that geometry is that language, as it allows researchers to move away from the specifics of the neural systems they study to consider more theoretical properties of computing systems. “The power of thinking in geometric terms is that it’s a level of abstraction that you can apply to other datasets,” says Russo. “You can compare across individuals, across species, across brain regions, across different tasks using the same metric.”
This power is not limited to biological computing systems but extends to artificial computing systems as well, such as networks of artificial neurons simulated on a computer. These artificial neural networks can be constructed to mimic the computational properties uncovered in data using geometrical analysis and can themselves be understood using geometrical analysis. For example, in their work Fusi and Salzman constructed artificial neural networks to perform the exact same task their monkeys performed. Using their geometrical approach, they found the exact same geometry in the activity of the artificial network. Likewise, Churchland combined geometrical analyses and artificial neural networks to both confirm and enable him to understand what he saw in his data: Networks trained to perform the cycling task had difficulty stopping if they didn’t represent a helix somewhere in their activity.
Many researchers think that the combination of geometrical analysis, neural recordings and artificial neural networks is the key for driving our understanding of artificial and biological computing systems forward in a profound way. “The connection between what the real networks are doing and what the artificial networks are doing also becomes very clear when you plot things as shapes in state space,” says Churchland. “Your eyes can immediately see the important features that connect those solutions and immediately see past the little differences that really don’t matter.”
Lacking a basic descriptive language, early analyses of complex neural datasets left some terrified that the brain might just be a tangled mess, beyond our understanding. Geometrical analyses have shown us that, although the data are complicated, with the right language we can uncover an orderly, principled structure in the brain, even if that structure is challenging to find. “When this approach can be successful, it can be very demystifying,” says Churchland. “Suddenly, all of these funny things that might have required a variety of different explanations, or just seemed odd, or been the kind of thing where somebody would be like, ‘Oh yeah, well, you know, biology is complicated,’ or ‘biology is messy,’ now they just all make sense. Geometrical thinking has the ability to do that a lot of the time. And then you can move on and try to understand the system more deeply rather than to pretend that that complexity is not there or dismiss it by calling it messy.”