A Scientist’s Quest for Better Brain-Computer Interfaces Opens a Window on Neural Dynamics
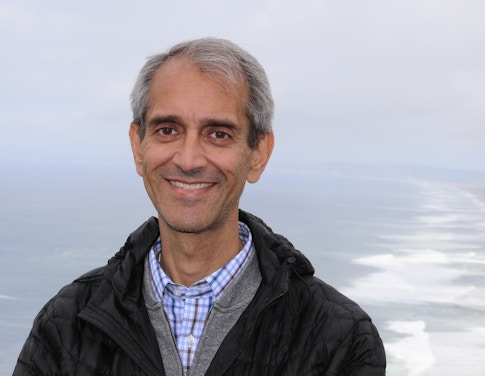
Krishna Shenoy launched his lab at Stanford University in 2001 with a lofty goal — to build more effective neural prosthetics for profoundly paralyzed people. Over the next 20 years, Shenoy and his team would make fundamental discoveries about how the brain encodes and executes motor commands, applying those insights to improving brain-computer interfaces (BCIs). A key component of that process was the development of a novel — and sometimes controversial — framework for analyzing neural activity.
Known as the dynamical systems approach, or more recently ‘computation through dynamics,’ this framework offered a way to capture the behavior of a population of neurons as a whole over time. It has since become one of the dominant paradigms for understanding how groups of cells encode and process information. Its ideas were central to the launch in 2014 of the Simons Collaboration on the Global Brain, a program dedicated to understanding population coding at single-cell resolution.
“Krishna had both a creative vision that was ahead of its time and the rigor and carefulness to pull it off scientifically,” says Bill Newsome, a neuroscientist at Stanford and one of Shenoy’s long-term collaborators, as well as a founding member of the SCGB. “I think he will be seen as a seminal figure in the history of neuroscience.”
Shenoy died in January at age 54, more than a decade after being diagnosed with pancreatic cancer.
While he was lauded for his scientific contributions, those who knew Shenoy, even briefly, noted his equally remarkable kindness and deep devotion to his trainees. He nurtured a highly collaborative lab that launched the careers of dozens of neuroscientists and engineers. “He taught me so much about how to be a good person — how to be thoughtful in every interaction, how to be super caring,” says Byron Yu, a neuroscientist at Carnegie Mellon University and one of Shenoy’s first graduate students. “He’s an excellent example of how to be a highly successful scientist and an amazing person at the same time.”
“He was both ambitious and humble; that’s rare,” says Mark Churchland, a neuroscientist at Columbia University and Shenoy’s first postdoc.
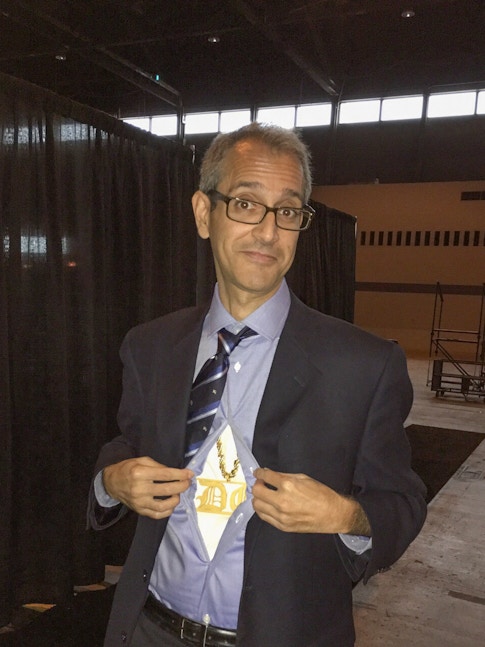
Throughout his long illness, Shenoy’s commitment to science, patients and his team remained. “Even after he got sick, he felt this duty to use the time he had to do something to benefit mankind,” Churchland says.
A vision for the field
The setting for Shenoy’s lab at Stanford was an unusual one — he launched a primate lab within the department of electrical engineering. But it was fitting for what he set out to do: to improve the nascent field of brain-computer interfaces by combining cutting-edge neuroscience and rigorous engineering. “Krishna recognized right away that brain-computer interfaces were the perfect mix of engineering, his fascination with the brain and helping people,” says Aaron Batista, one of Shenoy’s early postdocs, now at the University of Pittsburgh.
Newsome, whose lab was next to Shenoy’s, recalls watching Shenoy assemble his team. “He built a diverse group with esprit de corps, selecting engineers who really wanted to learn about the brain and neuroscientists who understood there was something to this dynamical systems approach,” Newsome says. “He had a nose for selecting amazing people, and they started generating impactful paper after paper. It soon became clear it wasn’t just a series of papers — it was a vision for the field.”
Shenoy’s first recruit was Churchland, who joined the lab as a postdoc in 2001. They pored over the literature together, discussing what made sense and what didn’t. “I was immediately inspired talking with him,” Churchland says. “He was unafraid to talk about the different ways things could work. Were there better possible explanations? If so, how do we test them?”
They discovered, for example, that neural activity recorded from the premotor cortex of monkeys as they reached in different directions was much more complex than textbook examples had led them to believe. Instead of seeing individual neurons showing a preferred movement direction, they found that neurons had complex patterns that varied from condition to condition. Churchland, Shenoy and collaborators debated why this region of the brain, whose job was to coordinate muscles, might behave that way. The answer lay in looking at populations of neurons as a whole, rather than focusing on individual cells.
Shenoy and many of his mentees came from the engineering world, and they applied a common engineering framework, known as dynamical systems, to the brain. “Those ideas are core to a lot of things we do in engineering, but only in the last 15 years have they become core to neuroscience, and I think Krishna has been essential for that,” says Chethan Pandarinath, a former postdoc in the Shenoy lab who is now at Emory University.
Engineers use the dynamical systems view to study systems that vary in time, such as fluids or the weather. According to this perspective, the dynamics of the system are governed by hidden, or latent, variables that researchers must infer by recording from noisy single neurons. In contrast to other efforts to analyze populations of neurons, such as principal component analysis, the dynamical systems approach incorporates the variable of time, says Maneesh Sahani, a neuroscientist at University College London and a long-term collaborator of Shenoy’s who, along with Yu and John Cunningham, now at Columbia, helped develop the mathematics of latent variable models and apply them to neural population data.
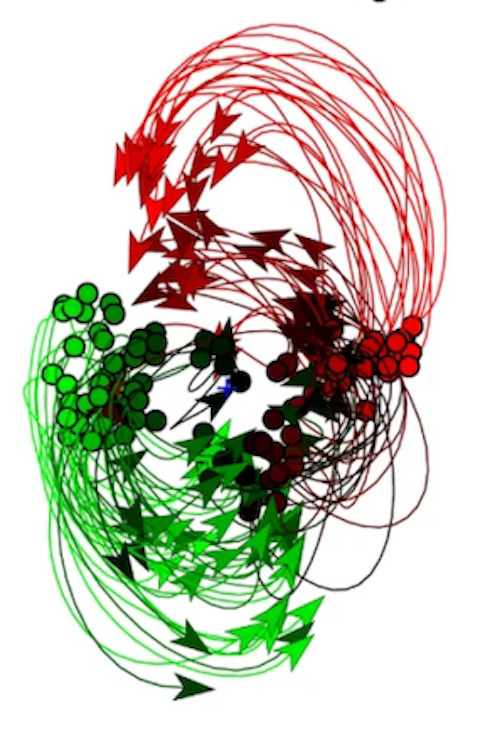
Shenoy’s focus on the motor system was the perfect place to explore this framework, Newsome says. In contrast to most sensory systems, where neural dynamics are driven by changes in the external world, “the point of the motor cortex is to generate dynamics that govern movement.”
Moving the conversation
In 2012, Churchland, Shenoy and collaborators published an article in Nature demonstrating key support for the dynamical systems idea. Using newly developed methods for analyzing the activity of populations of neurons, they found that population activity followed a rotational pattern during movement, providing a rule governing how population activity evolved over time. The rotational pattern started in different places depending on the desired direction of movement, providing an initial condition that shaped future activity. Churchland says that the paper was critical because it provided a concrete, dynamics-based account for how the motor cortex performs a task, explained the seemingly odd properties of single-neuron responses, and predicted population-level phenomena that could be confirmed in data.
David Sussillo, who joined the Shenoy lab in 2010, used artificial neural networks — a technique that was highly novel at the time — to provide additional support for the dynamical framework. Sussillo and collaborators trained a recurrent neural network to produce muscle activity patterns recorded during animal experiments. When they looked inside the artificial network, they found rotational dynamics, showing that these activity patterns are indeed sufficient to generate muscle commands. (For more on rotational dynamics, see “Discoveries of Rotational Dynamics Add to Puzzle of Neural Computation.”)
“I remember seeing that work and feeling for the first time there was a way to understand what the motor cortex was doing,” says Sergey Stavisky, who joined the Shenoy lab as a graduate student in 2011. In contrast to the standard approach of examining how the activity of pairs of neurons varied together, the new approach enabled researchers to analyze the time-varying activity patterns of hundreds of neurons in a manageable way. “Being able to wrap your head around what’s going on was very powerful,” says Stavisky, now at the University of California, Davis. “It felt fresh, qualitatively different — and a path forward.”
Because the dynamical systems view challenged the primacy of individual neurons in neural coding, it faced skepticism, particularly early on. Shenoy and collaborators sometimes had difficulty winning grants and publishing papers, but they kept building evidence. “Krishna’s way of doing battle with people who disagreed with him, some vehemently, was to listen, reason, discuss and ultimately do more experiments, to convince people by brute force of the evidence,” Newsome says.
In 2012, Newsome pitched a new collaboration to the Simons Foundation centered on the dynamical systems framework. “I got it directly from Krishna; he introduced me to that way of thinking,” Newsome says. Indeed, Shenoy’s work has been foundational to a number of central components of the SCGB. “He helped move the conversation from single neurons to populations of neurons, from one population of neurons to multiple populations recorded in different brain areas, from more static characterization of neural activity to dynamics and how dynamics can be important for computation,” Yu says.
Over the last decade, the dynamical systems view has become one of the predominant approaches to analyzing populations of neurons. “I don’t use the word ‘revolution’ very often, but it’s probably in the process of revolutionizing the basic paradigm for how we understand neural activity,” Newsome says.
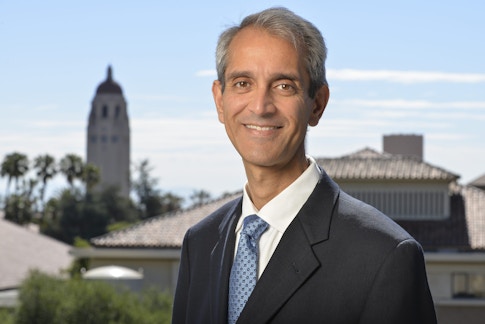
From basic science to translation
For much of his career, Shenoy ran two labs. The first focused on basic science, producing the dynamical systems work and serving as a vital test bed for improving neural prosthetics. A second translational lab, run in partnership with Stanford neurosurgeon Jaimie Henderson, applied those advances in clinical trials, dramatically improving BCI efficiency.
BCIs record neural activity directly from the brain and translate it for different uses, such as deciphering imagined speech or moving a computer cursor or robotic arm. In the early days, most BCI efforts focused on trying to decode movement signals from the motor cortex. One of Shenoy’s insights in developing BCI technology was to decode a movement’s endpoint rather than its trajectory. “Krishna realized that what matters for good BCI control was the goal, and he could decode goal information directly using premotor activity, without decoding kinematic details,” Batista says. “He got a lot of pushback from the community on this idea; it was just too unexpected.” (Ultimately, Shenoy and his team developed algorithms for decoding both goal-oriented and motor activity.)
Shenoy also advanced the field by applying the precision of an engineer. “He made the BCI field more rigorous and quantitative, moving away from one-off demos of moving a robotic arm to thinking carefully about specific metrics for performance and progress,” Pandarinath says. “It was important to him that we quantitatively show we are making improvements, that we think about what our capabilities are and what we can offer people.”
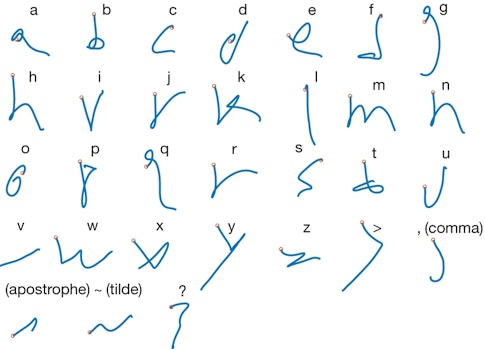
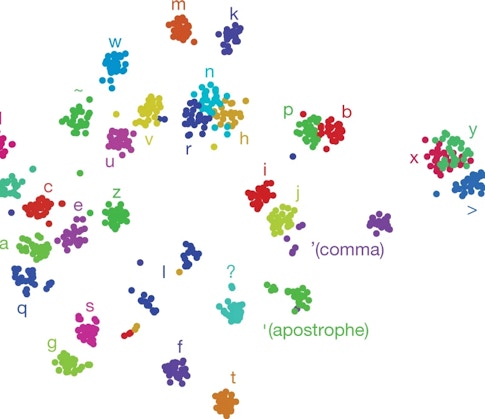
Indeed, his team set a number of records for BCI performance. In a Nature paper published in 2021, Shenoy and collaborators decoded neural activity as a BCI user imagined writing letters, producing 90 characters per minute, more than doubling the previous record for an intracortical BCI. In a preprint posted in January, Francis Willett, Shenoy, Henderson and collaborators describe a BCI that comes close to the speed of natural speech, capable of decoding speech at 62 words per minute, more than three times the previous record. “I don’t think people in the early 2000s would have predicted how quickly Krishna and his lab drove performance higher,” says Cindy Chestek, a former Shenoy postdoc now at the University of Michigan. “I would argue he is the field’s first engineer.” (For more on the work by Shenoy and collaborators on decoding human neural activity, see “Cracking the Neural Code in Humans.”)
Shenoy wanted the technologies he and others were developing to make their way to patients, and he consulted with a number of neurotech companies. He aimed to keep industry research grounded, and he wanted to ensure it was commercialized in a responsible way. “He worked closely with founders of BCI companies to educate them about what was possible and what was hype and to call them to their greatest selves,” Batista says. In a talk for the SCGB community in 2022, Shenoy predicted that progress in commercialization over the next five years could make or break the field. “If it goes well, it could start reaching hundreds of thousands of people,” he said. “It’s a very interesting time.”
Shenoy’s influence altered not just the basic approach to BCIs but the culture of the field. “BCI was a notoriously aggressive field when it started, but Krishna fostered a collaborative spirit that persists,” Batista says. “The field is different because of him.”
People first
Before his first surgery for pancreatic cancer in 2011, one of the first things Shenoy did was bank recommendation letters for everyone in his lab. He continued to update them whenever he felt his health getting worse. When Shenoy entered the hospital for the last time earlier this year, Henderson recalls, he worried about finishing a recommendation letter. (He finished it.) That dedication, even in times of great stress, illustrates Shenoy’s approach to mentorship. “Krishna was about knowing people and loving people,” Henderson says. “The science was paramount, but even more important was his care for people, particularly for trainees. His mantra was trainees first.”
A meeting with Shenoy was often a balm to stressed scientists — trainees and collaborators note his extraordinary ability to encourage people. “You’d walk into his office feeling disappointed about an experiment and walk out floating, thinking you’re working on the most important thing in the world,” Stavisky says. “He was really good at that.”
Shenoy’s former mentees marvel at how generous he was with his time, even after his cancer diagnosis. “I know he has 100 things on his to-do list, but when I sat down with him, it felt like he had the whole afternoon for me,” Yu says. “That is something that was amazing and truly special about him.” That dedication led Shenoy to be chronically late, a trait affectionately dubbed ‘Krishna time.’ “He was always late because he was giving the person before you whatever they needed,” Churchland says. “‘Krishna time’ was a joke, but it was a sign of commitment.”
Shenoy nurtured both a highly collaborative and a highly independent lab. Trainees worked together in teams, and Shenoy consulted lab members before welcoming a new addition. “Everyone felt that they were part of the same team at all times,” Chestek says. Shenoy also encouraged people to direct their own research, asking newcomers to the lab what they wanted to work on. “That’s how he called people to be their best, by inviting them to design their own project,” Batista says. “Our lighthearted metaphor is that he set the initial conditions and let dynamics unfold from there — we were the dynamics. Every now and then he would tap the fish tank to get us to pay attention to some interesting thing.” Partnerships born in the lab were often long-lived as well. Batista and Yu, for example, have been collaborating ever since a suggestion Shenoy made 20 years ago.
Shenoy’s mentees continued to consult him long after they left the lab. “He was my emergency adviser — I called him every time there was a disaster in my new lab,” Chestek says. Batista also continued to reach out to Shenoy for advice and support. “He stayed invested in us — he seemed to have the ability to drop everything and give you all the time you needed,” Batista says. “I don’t know how he did it.”
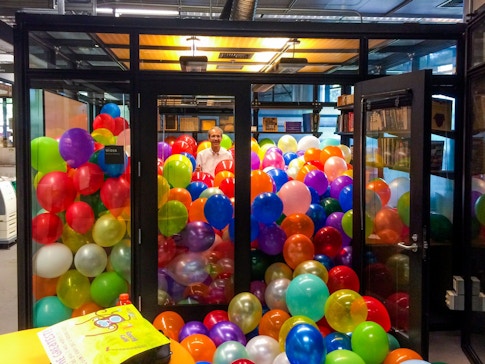
Shenoy was a notorious prankster, especially in his early years, though his collaborators and mentees are largely mum about the substance of those pranks. Churchland recalls a post-conference escapade that found the pair on the roof of a building at the University of Cambridge fleeing security guards. “We knew him as brilliant but playful and free-spirited,” Batista says. “He would happily lose half an afternoon playing pranks on someone knowing full well that it would come back to him.” His trainees returned that playfulness in kind, dousing him in Gatorade when he won tenure and celebrating his birthday by filling his office with hundreds of balloons.
In one oft-cited annual tradition, current and former lab members would gather for dinner and take turns telling an embarrassing story about themselves. “It was an icebreaker that made people seem human to each other,” Yu says. On a deeper level, it helped to create an environment where people felt free to share challenges and failures, Chestek says. (As in the famous Las Vegas saying, the content of those stories remained at the dinner table.)
His legacy lives on in his mentees, who attempt to emulate his approach, channeling Shenoy in their interactions with trainees. “What would Krishna do?” became a popular refrain. “The perspective I got from Krishna was to get the best people and believe in them,” Sussillo says. “I would rather have a project fail because someone went out on a limb than because of micromanagement.” Yu reminds himself to be compassionate, to consider the conversation from the trainee’s perspective and to orient the conversation to them.
“We all talk about Krishna as if he were a saint,” Churchland says. “He wasn’t, of course, but he was much closer to perfection than almost anyone else I know.”
—
For more on Shenoy’s life and work, see these obituaries written by some of his former mentees:
Batista AP, Pandarinath C, Yu BM. Krishna Shenoy (1968-2023). Neuron 2023;111(6):764-766.
Churchland MM and Nuyujukian P. Krishna V. Shenoy (1968–2023). Nature Neuroscience 2023.